Introduction
The principal objective of image enhancement isto enhance the understandability or view of data in images for human watchers. It is also helpful to give a better contribution for other automated image processing techniques. The graphic display for analysis become more accessible by accentuating or sharpening image features, for example, edges, boundaries, or contrast [1]. The inherent information contents of the data don't increase in the enhancement process. However, it expands the dynamic range of the picked features with the goal that they can be distinguished effortlessly. Nowadays, this has been turned into the concentration of the focus in numerous fields, for example, public security, archaeology, military, medical imaging and three-dimensional image [2]. The image enhancement techniques focus attention on certain features of interest in an image and draw out the features in an image that is obscured. The contrast adjustment, filtering, morphological filtering, and deblurring are the various enhancement techniques. A modified version of the original image is achieved through these image enhancement operations and can also be utilized as a preprocessing initiative to upgrade the after effects of image analysis techniques [3]. Frequency based domain image enhancement is a technique, which is utilized to give details of the study of mathematical functions or signals regarding frequency as well as operating them on the transform coefficients of the image, for example, discrete cosine transform (DCT) [4], Fourier transform, discrete wavelet transform (DWT). The motive of utilizing thisterm is to improve the image by controlling the transform coefficients. There are a few merits of Frequency based image enhancement such as including the low complexity of the calculations, facile vision as well as the easy applicability of special transformed domain properties, controlling the frequency composition of the image. The appearance of low-quality images is enhanced by working with traditional methods of image enhancement.
Super-resolution is a technique which is used to create a high-resolution image by taking one or various low-resolution images. This method is conceivable to describe an image or high-frequency components which may be missed because of numerous causes, ambient light from different elements, as well as the low number of camera sensory cells, the movement of the camera and not alter the camera's focal point.
The lost features of the image can be recovered by using this process of super-resolution. Also, super-resolution minimizes the blurring and aliasing of images and interpolates or predicts lost information from accessible evidences. In numerous applications of machine vision and image processing, the Super-resolution methods are used. Today, advancements came in systems; introducing higher precision and more processing power, which has made more consideration of super-resolution methods based on software. This technique is utilized for many monitoring machines, for example, face recognition, identification and license plate recognition, remote sensing, automatic target recognition, medical image processing, for example, CT and MRI and converting video to different standards (for example, converting NTSC to HDTV), image enhancing, processing of satellite images, microscopic image processing and astronomical image processing.
Adaptive Shock Filters
[Jinsheng Xiao et al.,] discussed that an adaptive shocking filtering model has following properties:
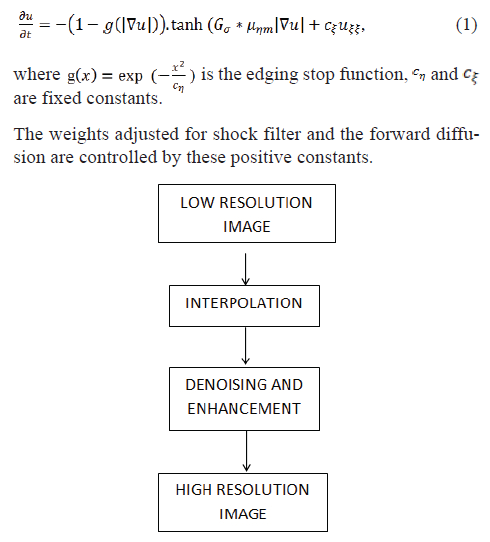
Fig 1: Flow chart of Image Super-Resolution and Enhancement
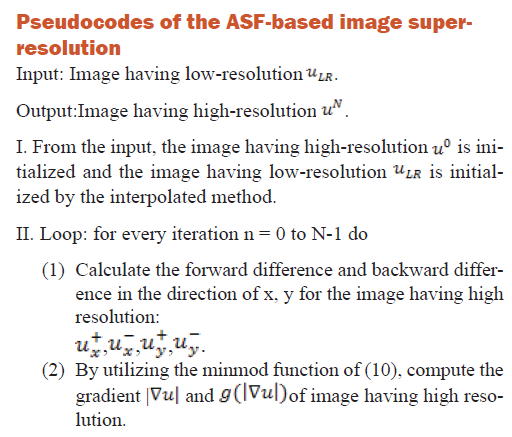
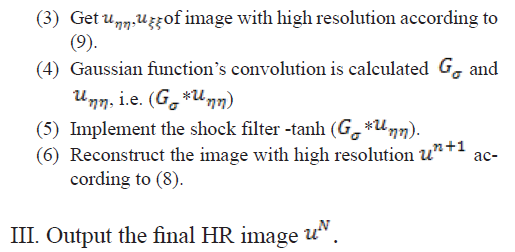
Artificial Bee Colony
In the Artificial Bee Colony model, there are three categories of honey bees: employed bees, onlookers and scouts. In this colony, this is supposed that there is merely one artificial employed bee for each and every food source. Also, the number of food sources in the model is equal to the number of employed bees around the hive. Firstly employed bees would go to their food source as well as after returning they dance on their hives. The food source of employed bee has become abandoned and turns into a scout and begins to look for searching another food source. The remaining group which is onlookers, watches the dances of employed bees as well as chooses food sources relying on their dances. Some of the key steps of the algorithm are given beneath:
1. For all employed bees, the initial food sources are produced.
2. Repeat the step until all employed bees get the food sources.
3. Then all employed bees remind food sources in their memory and go towards those food sources and afterward, a neighbor source determined, and then calculates the nectar amount and started dancing in the hive.
4. Onlookers choose and select their food sources by observing the dance of employed bees, and then go to that selected food source. She evaluates its nectar amount after choosing a neighbor around that.
5. When the scouts discover the new food sources, the abandoned food sources are resolved as well as substituted with those new food sources.
6. The elite food source is finalized from all of the available food sources.
7. UNTIL (requirements are met).
Discrete Wavelet Transform
The discrete wavelet transform is an execution of the wavelet transform utilizing a discrete set of the wavelet scales as well as translations complying with various characterized rules. Also, that type of transform disintegrates the signal into a mutually orthogonal set of wavelets. This transform is the primarydistinction from the CWT and its implementation of the discrete time series sometimes known discrete-time continuous wavelet transform. Similar to the case in Fourier analysis, the DWT is invertible, so that the initial signal can be totally recuperated from its DWT representation. Not at all like the DFT, the DWT, indeed, alludes not simply to a single transform, but instead a set of transforms, each with an alternate arrangement of wavelet basis functions. Haar wavelets and the Daubechies set of wavelets are two of the most common wavelets.
There is a scaling function from which the wavelet should be created and this scaling function portrays the scaling properties. There is a constraint that the scaling functions should be orthogonal to its discrete translations suggests various mathematical restrictions that are specified all around such as the dilation equation
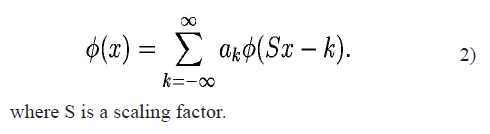
Related Work
C. Munteanu et al. (2004) [16] has described a new approach to automatic Image enhancement. It is a visually more agreeable, low noise output to get the image as an input image. Therefore, image enhancement is a troublesome undertaking task to automate the procedure of analyzing and attempting to dispose of human intervention. This paper is another automatic image enhancement technology powered by a developmental adaptation process introduction. They have new objective criteria for enhancement, according to the test and try to find the best image. Due to the high complexity of criteria planned. They are the best for a global search strategy as an evolutionary algorithm (EA). They contrasted their technique with other automatic enhancement methods, such as contrast stretching and histogram equalization. The conclusions as far as both subjective and objective evolution demonstrates the excellency of their process.
Kabir et al. (2006) [17] has presented a technique for image contrast enhancement based on Block based intensity-pair distribution. Added intensity distribution utilizing the whole image, instead of the estimated intensity takes this image-pair algorithm proposed and an expanding distribution according to the intensity of the pixel map. A linear magnitude mapping function is utilized in place of non- linear to control over-enhancement and the contrast stretch. This preserves the gray level linear mapping.
Block local information easily contrast enhancement, intelligent edge brings out information and removes the noise from the image. For an extended analysis of image features, the approximate algorithm is the best.
Tokuda et al. (2007) [18] has defined thatgamma correction image quality adjustment parameter one of the image quality enhancement support system reflecting user subjectivity. Optical gamma value’s derivation as an optimization problem is studied in this. To reflect user subjectivity, a support system by Interactive Evolutionary Computing image quality enhancement is realized. This technology is a gamma value derived from manually, image quality and verified by comparison with etymology.
Jafar et al. (2007) [19] has proposed that contrast enhancement is an essential step in virtually every image processing and computer vision application to achieve the needed level of enrichment different applications for different methods. Consumer electronics such as any enhancement techniques to enhance both local and global details should be able, yet not at the expense of decay in the quality of the image. He called his early work on the advancement of new methods-based histogram equalization in the current multi-level component image contrast enhancement. This method is effective and simple and visible image at least distortion with both global and local contrast enhancement to allow thresholding the grey level histogram equalization method, multiple classic and connected component analysis taking advantage of the capabilities of Visual and quantitative evaluation technique for achieving this goal than revealed.
Chen et al. (2008) [20] has proposed a new fuzzy based contrast enhancement technique for remote sensing images.Traditional advancement methods frequently involve highly areas in black and bright local brightness details based on fuzzy set theory. Fuzzy set theory intended use grey due to the traditional division by values to avoid cracks. The main concept of the theory is that the elements of an interval [0, 1] instead of binary value membership degree. Hence, a complex process can be considered as an algorithm involves three stages: first, the satellite image gray-level c-fuzzy membership space is transformed by means of clustering. Secondly, each cluster of the suitable stretch model is constructed of the same membership base. Third, image gray-level space stretches back to the gray value changes by merger of each cluster. From the outcomes, obviously the, proposed technique can enhance the image conception and higher key measurement.
Lee. he et al. (2008) [21] has suggested that a new color image enhancement algorithm based on MSR. Appropriate wavelet base input image disintegrated in three levels to be selected. Then decompose the process input image into different enhancements algorithms employed in three levels. Then decompose the different enhancement algorithms Wavelet coefficients and coefficients were employed to scale. Wavelet coefficients, each level character, after a revised analysis of Adaptive non-fast mask technology, which improved image contrast, more clear and colorful image processing was used. Experiments show the projected algorithm enhanced some outdoor images obviously.
Gaps in Study
[Jinsheng Xiao, Guanlin Pang, et al.,] discussed that by conducting the review, it had been found that the various gaps in earlier work on image super-resolution and enhancement techniques.
1. The effect of noise and edge preservation is ignored in existing super-resolution and enhancement technique.
2. The use of artificial bee colony while super-resolution and enhancing the image is also ignored by existing researchers
3. The majority of existing research focuses on natural images. The effects of image super-resolution and enhancement on face images are ignored.
Methodology
PROPOSED ALGORITHM
1. Start by reading the image
2. Apply DWT on the image
3. It splits the image into four bands of data-LL, LH, HL, and HH.
4. Then apply adaptive shock filter on LL.
5. Afterward, apply artificial bee colony to find optimistic adjustment value.
6. Then adjust the actual image using optimistic artificial bee colony.
7. It will give the improved versions of all bands of data as seen in flow chart-Improved LL
8. Then apply inverse DWT.
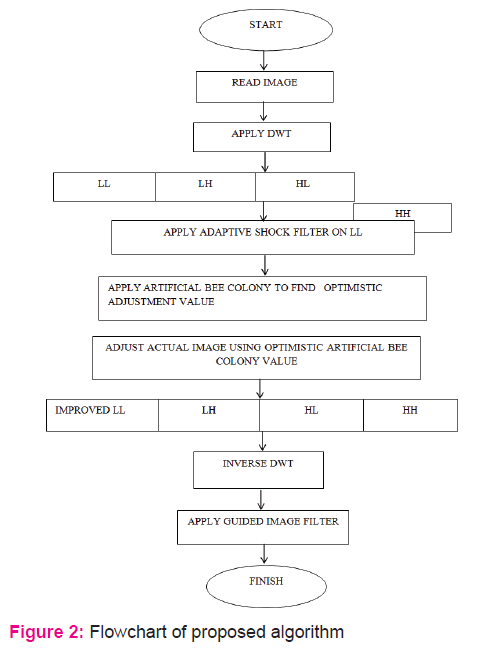
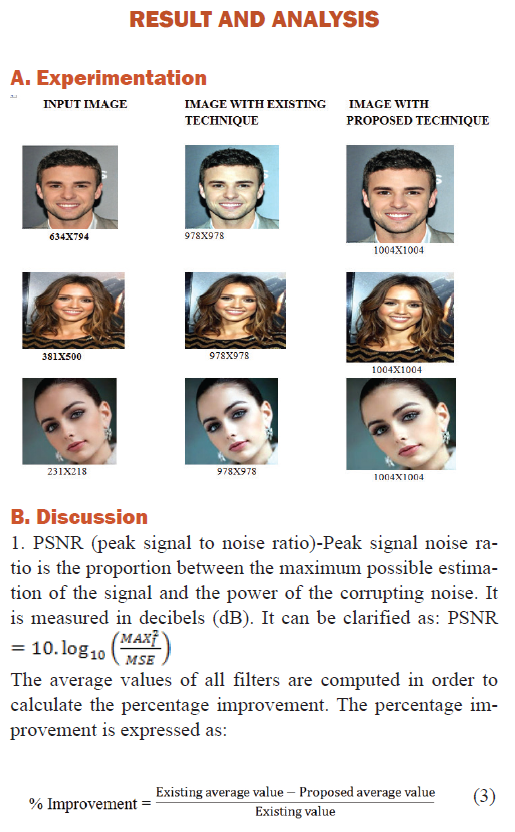
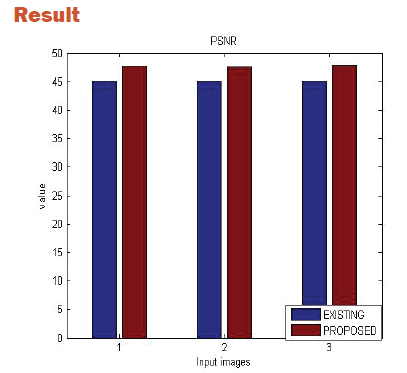
Discussion
PSNR is calculated by taking three images. From the graph, it can be easily depicted that the PSNR using Artificial Bee Colony is more than that of PSNR using Adaptive Shock Filters. PSNRs in the case of proposed technique are 47.7104, 47.5603 and 47.8784.
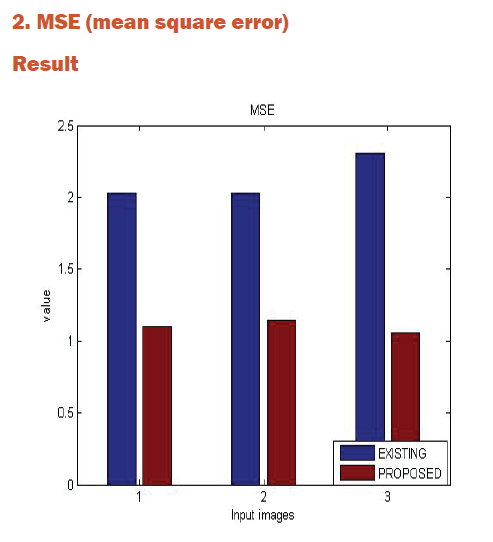
Discussion
Mean square error is to figure an error signal by subtracting the test signal from the reference and afterward computing the average energy of the error signal. This can be calculated as:
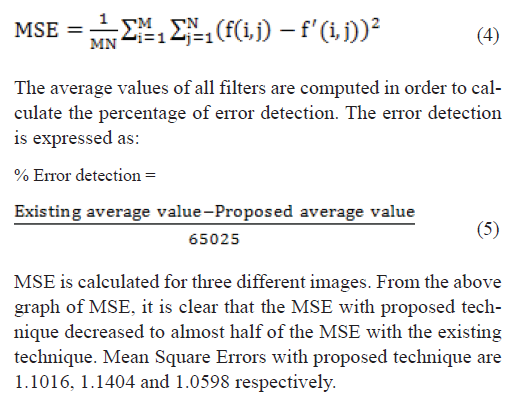
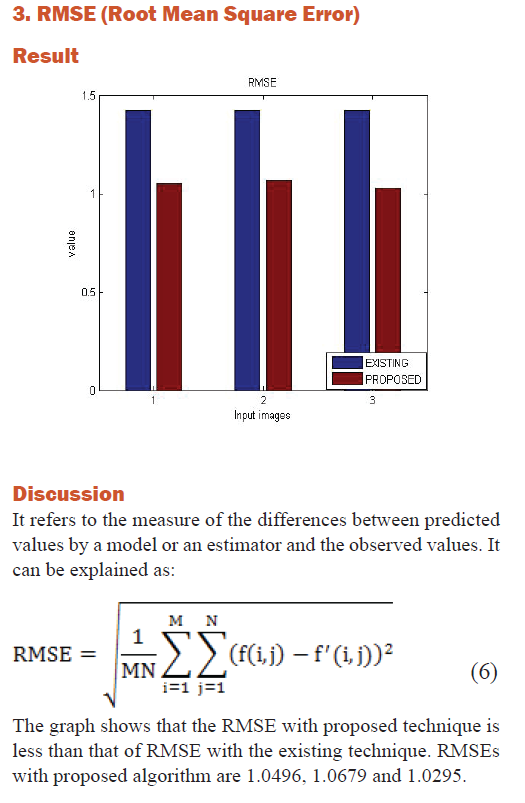
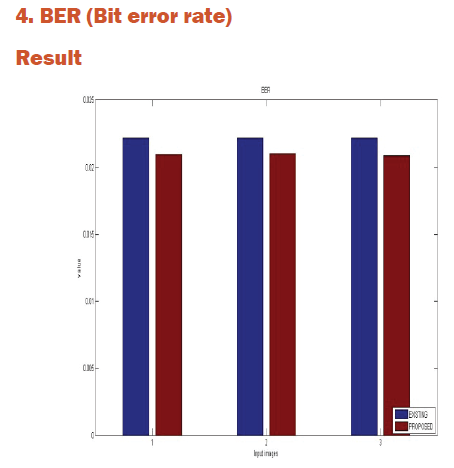
Discussion
BER is the rate of occurrence of errors in a transmission system. It can be simplified as number of bit errors separated by the aggregate number of transferred bits during a considered time interval. This definition can be simplified into a basic equation:

BER is calculated using the proposed technique on three different images. From the graph, it is clear that the BER with proposed technique starts decreasing. The calculated Bit Error Rates for three images are 0.02096, 0.021026 and 0.020886.
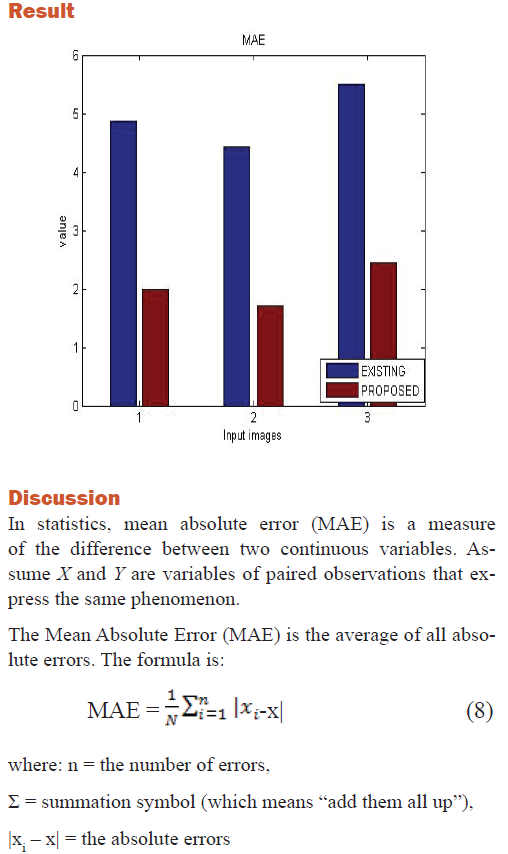
Mean Absolute Error is calculated on three images using proposed algorithm. The achieved result is approximately half to theMAE with existing technique. MAEs with proposed technique are 1.9859, 1.7054 and 2.446.
Conclusions
This paper has tended to the issue of face super-resolution and enhancement in light of artificial bee colony. First, discrete wavelet transform is employed in the facial image which separates smooth variations and sharp variations. Then the adaptive shock filter is applied to various bands of data. Then, artificial bee colony is used to find optimistic adjustment values, which adjusts the actual image using optimistic ABC. This will give the improved bands of data. Finally, inverse discrete wavelet transform is employed in the image to reassemble the various classes of data into a reconstructed image. Finally, the guided image filter is applied to preserve edges in more optimistic manner and then an enhanced image along with super-resolution is reconstructed. The experimental outcomes prove that the proposed algorithm has preferable outcomes over the current technique based on adaptive shock filter model both in quantity and quality.
Acknowledgement
It is a great pleasure to express my deep sense of gratitude and heartfelt thanks to the various persons who have been of enormous help in one way or the other of successful completion of this research. They not only inculeated in me the fath and confidence but offered very tangible, moral, pyschosomatic support in every resect.
First and foremost I would like to express my gratitude to my thesis guide Prof. VikramMutneja for his guidance, advice and constant support throughout my research work. He has been a great source of motivation and always eager to help. It was an absolute pleasure to work under his guidance. Out of deep sense of gratefulness I express my sincere thanks to him. This research would have never acquired its existence without his help and cooperation. I also want to express my respects to Dr. Rajni, HOD of Electonics and communication Engineering Department.
I would like to thank all my family members and staff of the department of Electonics and communication Engineering, SBSSTC for their generous help in various ways for the completion of this research.
Least butnot last specially thanks to my family and friends. I am especially indebted to my parents for their love, sacrifice and support. They are my first teachers after I came to this world and have set great examples for me about how to live, study and work.