IJCRR - 14(7), April, 2022
Pages: 06-11
Date of Publication: 05-Apr-2022
Print Article
Download XML Download PDF
Smart SOP's Surveillance System using Deep Neural Network
Author: Chaman Lal, Zahid Ali, Shagufta Aftab, Suresh Kumar Beejal, Mehwish Shaikh, Ambreen Fatima
Category: Healthcare
Abstract:The global pandemic Corona virus sickness 2019 had a devastating impact on the world, according to statistics received by the World Health Organization. A study shows that about 33 million people are affected by the virus and more than 1 million deaths are reported worldwide. Currently, there are no specific vaccines or treatments for COVID-19. Now, WHO recommends that wearing a mask and following the SOP's can help us in preventing the spread of the virus. There are only few studies about face mask detection based on image analysis. To create a safe environment in public places, educational institutions, & shopping malls we are proposed an effective idea based on computer vision and AI which is focused on real-time automated monitoring through the camera to detect people whether they are wearing a mask or not, also checks body temperature by the sensor-based model. In this proposed system modern deep learning algorithms have been mixed with different techniques to detect the unmasked faces and temperature and generate warning alerts by capturing images of the people not wearing masks and if the person has temperature, then it will also generate an alert of having temperature. This system favors society in preventing the spread of the virus as it has already infected 33 million people worldwide. The goal is to evaluate the face detection through image processing to prevent society from the spread of global pandemic disease and pollution.
Keywords: COVID-19, SOP’s, CNN, Computer vision, DNN, & Sensor-based model
Full Text:
INTRODUCTION
The spread of COVID-19 has created global health crises. In December 2019, before the coronavirus was proclaimed a global pandemic, a new infectious respiratory disease arose in Wuhan, China, infecting thousands of people and resulting in 170 recorded deaths. The disease was dubbed COVID-19 by the 1World Health Organization (Coronavirus disease 2019). Coronavirus expanded fast over the world, posing major health, economic, environmental, and social issues to the entire world population. As a result, an increasing number of people are concerned about their health, and governments regard public health to be a major priority1 People should use masks to reduce the risk of virus transmission and keep social space between persons to prevent the infection from spreading, according to the WHO. The identification of face masks and temperature has become a computer vision challenge to aid society. This model explains how to prevent the virus from spreading by detecting a person's temperature and whether they are wearing8 a mask in public settings in real-time. It’s a real-time temperature measurement, and the flow of people can pass through quickly to avoid staying long and reduce the risk of cross-infection. The model is a combination of a lightweight neural network, and computer vision with the transfer of learning techniques to detect the unmasked faces and temperature through image processing by real-time monitoring of the people. Since the data set to detect the mask can be harder to extract, we use transfer2,9,10 learning techniques. Using Open-CV helps in deep learning the model3,7,11 and helps to meet the real-time requirements so if any person is detected with temperature or not following the SOP’s i.e., not wearing a mask, it takes a picture of the person not wearing the mask and send violation alert or notification will be sent to the system to take necessary actions.
BACKGROUND
As the global pandemic has infected over millions of people and caused more than 1 million deaths are reported worldwide and no proper solution has been discovered to stop the spread of the coronavirus. The COVID-19 virus is primarily transmitted through droplets of saliva or nasal discharge when an infected person coughs or sneezes, thus respiratory14 etiquette is very important (for example, by coughing into a flexed elbow). There are no COVID-19 vaccinations or therapies available currently. However, numerous clinical trials testing potential therapies are currently underway. As clinical findings become available, WHO will continue to offer updated information5. Now, WHO recommends that people should take safety measures and should follow the SOP’s and wear masks to prevent the spread of the virus4,6. A significant indication of COVID-19 infection is a rise in body temperature. Thermal screening is now carried out with handheld contact-free thermometers, which necessitates health personnel approaching the individual being checked, placing them at risk of infection. It's also nearly impossible to take the temperature15,16 of every single person in a public place.
To overcome this problem, we proposed a computer vision approach to help society in maintaining the environment12,13 in which SOP’s are followed strictly. We proposed a computer vision system model which is supposed to check the temperature of people and whether people are wearing masks or not by real-time monitoring of the people and if someone is captured not wearing mask or if they have body temperature then it will notify the authorities by sending them the warning alerts with captured images. It will help society in preventing the spread of the virus in public places.
RELATED WORK
Neural networks (NNs) are compositional models with more generic nodes and less interpretability than the previous models. The most well-known use of Convolutional NNs20,23. These models, in the form of DNNs, did not emerge as extremely successful on large-scale picture classification tasks until recently19. However, their detecting potential is restricted. Using multi-layer Convolutional NNs, scene parsing has been attempted as a more detailed kind of detection21. DNNs have been used to solve the problem of medical image segmentation18,22, which has a similar high-level purpose but uses a much smaller network with different features, loss function, and lacks apparatus to distinguish between multiple instances of the same class, is perhaps the most similar method to ours.
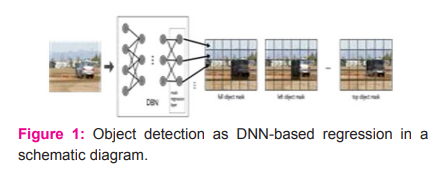
We perform object box extraction after regressing to object masks over many sizes and huge image boxes. The acquired boxes are fine-tuned by repeating the technique on the subpictures, which are cropped using the current object boxes. We just show the whole object mask for clarity, but we use all object masks.
-
CONVOLUTIONAL NEURAL NETWORK
CNN's are a sort of feed-forward neural network with many layers. Convolutional neural networks have layers with small neuron collections that each observe a small piece of an image. The results from all the collections in a layer partially overlap to provide the overall visual picture. Each filter takes a set of inputs, conducts convolution, and optionally adds non-linearity to the mix17. In picture and facial recognition, the most widely used algorithm is CNN. CNN is a sort of artificial neural network that extracts features from input data using convolutional neural networks to enhance the number of features.
-
DEEP NEURAL NETWORK
Image recognition is a specialty of deep25 neural networks (DNNs). The purpose of neural networks, which are computer systems that identify patterns, is pattern recognition. The name comes from the fact that their design is based on the structure of the human brain. In this system, there are three types of layers: input, hidden layers, and output. The input layer receives a signal, the hidden layer processes it, and the output layer produces a decision or forecast based on the input data. The network's layers are made up of interconnected nodes (artificial neurons) that execute calculations. Neural networks extract features directly from the data with which they are trained, removing the need for experts to manually extract them.
-
OBJECT DETECTION
Object detection, a subset of computer vision, is an automated approach for finding interesting objects in a picture in relation to the background. [Fig: 1] shows two images with foreground objects. The convolutional DNN established by is the foundation of our network. It has a total of seven layers, the first five of which are convolutional and the latter two of which are fully linked. As a non-linear transformation, each layer employs a corrected linear unit. In addition, max pooling21 is present in three of the convolutional layers. We suggest the reader to for more information.
-
TENSORFLOW BASED DETETCTION
The human visual system is fast and accurate, and it can execute complicated tasks like identifying many items at once, so humans can detect and identify an object in real-time. Because of the availability of massive datasets and machine learning methods, computers can now detect and classify multiple images with high accuracy, just like humans. Google Brain TensorFlow is an open-source toolkit for numerical computing and large-scale machine learning that makes it easier to gather data, train models, serve predictions, and refine future outcomes. TensorFlow is a software that mixes Machine Learning and Deep Learning models and algorithms. The steps for detecting objects in real-time are very similar to those described before. All we need now is an additional dependent, which is Open CV.
e. DETECTION BASED ON DNN
As illustrated in [fig. 2], the basis of our technique is a DNN-based regression towards an object mask. We may create masks for the entire object as well as parts of it using this regression model. We can get masks of many objects in a picture using a single DNN regression [fig. 2]. We use the DNN localizer on a small selection of huge sub-windows to improve the precision of the localization even more.
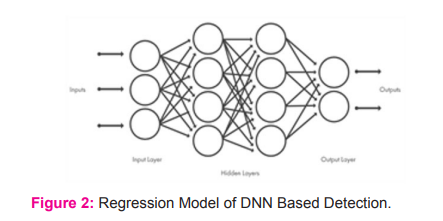
system requirement
-
TENSORFLOW:
Tensor flow is a free software package that may be used for a range of data flow and differentiable programming tasks. It's a symbolic math library that's also used in machine learning applications like neural networks. It's used by Google for both research and manufacturing. TensorFlow was built by the Google Brain team for internal use. On November 9, 2015, it was made available under the Apache License 2.0. TensorFlow's architecture enables computation to be distributed over a range of platforms.
b. OPEN CV
Open CV is a collection of programming functions geared primarily at real-time computer vision. Willow
Garage now supports it, which was originally built by Intel. The library is cross-platform and free to use, thanks to the open-source BSD license.
c.MT CNN
Multi-Task Cascaded Neural Network (MTCNN) Fig.
4 is an acronym for Multi-Task Cascaded Neural Network. A convolutional neural network is a type of neural network that recognizes faces and facial landmarks in images. It consists of three neural networks coupled in a cascade.
d.KERAS
Keras is a neural network library written in Python. As a foundation, it can be built atop TensorFlow, Microsoft Cognitive Toolkit, Theano, or PlaidML. It's user-friendly, modular, and expandable, to facilitate deep neural network research quickly.
e.LINUX
Compared to other operating systems, Linux is more stable and secure (OS). Linux and Unix-based operating systems have fewer security vulnerabilities since the code is regularly checked by many developers. Its source code is also available to anyone.
f.PI INTERFACING CAMERA
The Pi 4 can be used for a vast array of image recognition tasks, and the creators of the device seem to have recognized.
g.LCD DISPLAY
LCD display will be used to display pictures of person wearing masks or not wearing masks which are detected by the system. Also, it will help in displaying messages like when it detected an unmasked face.
h.SENSOR FOR TEMPERATURE DETECTION.
The sensor will be needed to measure temperature24 on real time in [fig. 3].
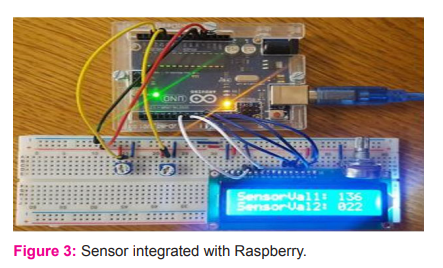
METHODOLOGY
Using Deep Learning algorithms, it was possible to detect temperature and unmasked faces. Our project is divided into two phases: training the model by giving data set and implementing the detection system based on image processing. For this purpose, we divide it into different phases mentioned below:
a.DATA COLLECTION
In phase 1 we will collect the data set that will help us in training the model that how the system detects if the person is wearing mask and following SOP’s or not. For this purpose, we will take different pictures of people wearing masks and not wearing masks to train the model on what measures the system will identify who is wearing masks or who is not wearing mask in public places. Some examples are shown in figure below with masks, without masks, hand masks, with or without masks in one frame and confusing images without masks.5,6 Also, we will give data set to recognize the body temperature automatically by sensor.15,16
b.MODEL TRAINING
Due to limited size of data set of face mask it is difficult for learning algorithms to learn better features. Our proposed system uses transfer learning techniques to train the system model [fig. 4] that on what measures will it detect the temperature and checks if the person is wearing mask or not. The data set is loaded into the project directory, and the algorithm is trained using photographs of people wearing masks, without masks, and images that are perplexing14 without masks. All the images and data set for thermal screening of body temperature from phase 1 creates a baseline for our project it will help the algorithms to learn objects which shows that transfer learning can increase the face detection performance by 3 to 4% and enhance the accuracy level. The model is trained by using TensorFlow for better image processing compiled with MTCNN algorithm which improves the accuracy level by 99.99%.
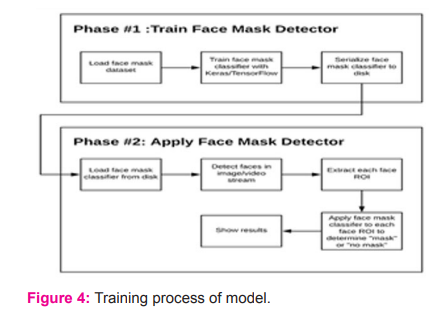
c.MODEL IMPLEMENTATION
After training the model we must implement the algorithm-based system i.e., MT CNN algorithm with the camera to automatically track the public areas to prevent the spread of COVID-19. The camera will help the trained model to detect on real-time in public places if a person is not wearing masks or have temperature. The system will also help in maintaining a hygienic environment in public places. Also, it improves the accuracy level by 99.99%.
d.MODEL TESTING
Once the model is completely trained and is given all the data set to measures the detection of people not wearing mask, we check the accuracy level of the model based on the data set given to it by showing the bounding box with green colour. If the person in the bounding box is not wearing a mask or the mask is not visible to the camera or if the person is having temperature15,16 it will change it colour into red and capture its image and will generates a warning and send alert to monitoring authorities with face image. To begin, a CNN technique7 is utilized to create a huge number of bounding boxes that span the entire image5,6 (that is, an object localization component). Then, for each of the bounding boxes, visual features are extracted. The system accuracy level is approximately 99.99%.
We can see how the model identify the images with and without masks with red and green boundary boxes. The red box will appear when the camera captures any faces without mask and temperature body then it will generate alerts and notify the authorities otherwise the boundary box will be green showing that people are wearing masks.
RESULT AND ANALYSIS
In all the images we include in the data set we have given some images of people wearing masks, some not wearing masks and some confusing images and some techniques on how to measure the temperature of the body. The data set was used to train the model which gives the accuracy of 99.99% 8,9,13. When the model is completely trained by implementing the deep learning algorithms, it will help the society in maintaining the SOP's in public areas when the camera monitors any person in the boundary box without mask or have temperature it will automatically generate warning alerts and send the notification to the monitoring authority with the face image that violates the rule or have body temperature. Resulting in an environment where we can prevent the spread of this global pandemic coronavirus and polluted environment.
The above pictures are showing the results of a person who is captured by the system not wearing mask and at the same time it is showing the results with a message "Please wear mask to defeat corona" in a green boundary box. Below has one more person detected not wearing mask.
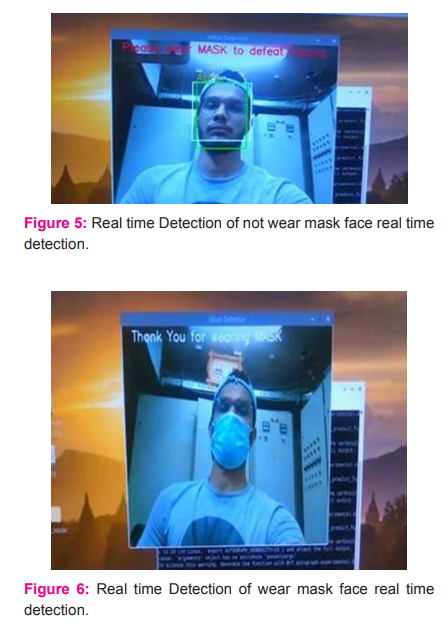
As in this pic, we can see the system does not recognize the name of the person it is because we have not feed its data in the system, but it can still detect the not wear mask [fig. 5] and wear mask [fig. 6] faces.
The proposed system can detect the temperature and unmasked faces by real-time monitoring of the people using image processing and data entry.
The system is user-friendly; we can get the data of captured images easily.
The system monitors the people in the boundary box and if it detects a person having a temperature or without mask it will change the color of the boundary box into red.
The system keeps the detected picture in the database for future use.
The system will notify the authorities if it detects temperature or unmasked faces by capturing their pictures automatically.
Data entry and image processing of large data set can improve the accuracy level of the system.
This system with more modification will help the society in future.
The system has accuracy level of 99.99%.
CONCLUSION
The proposed system will contribute to the healthcare sectors through which we can help society in preventing the spread of the pandemic virus COVID-19 even after the vaccine is invented. Detection of temperature and unmasked faces by image processing and data entry, which can contribute to public healthcare. The architecture is based on the two main phases which are training face mask and temperature detector and implementing the face mask and temperature detector. To extract large data set, we used transfer learning technique to train the model. If someone is captured not wearing mask it will automatically generates warning alerts with image of the person to take necessary actions to maintain the SOP’s based environment and to prevent the spread of the coronavirus. On training the model, we got an accuracy of 90%. As a result, it may be used to measure temperature and monitor uncovered faces in crowded places such as train stations, bus stops, markets, streets, mall entrances, schools, and colleges.
ACKNOWLEDGEMENT: The researchers whose works are cited and listed in this paper's references have been of tremendous assistance to us. The laboratory facility for the analysis deserves special mention. The authors express their gratitude to individuals who contributed to the study's improvement for being patient.
Source of Funding: Nil
Conflict of Interest: None
Author’s contribution:
Chaman Lal: writing of final drafts and data collection.
Zahid Ali: Manuscript Final Editing
Shagufta Aftab: writing of first draft.
Suresh Kumar B: Manuscript Editing.
Mehwish Shaikh: Editing of first draft.
Ambreen Fatima: writing of second draft.
References:
[1] Covid CD, Team R, Covid CD, Team R, COVID C, Team R, Bialek S, Gierke R, Hughes M, McNamara LA, Pilishvili T. Coronavirus disease 2019 in children—United States, February 12–April 2, 2020. Morbidity and Mortality Weekly Report. 2020 Apr 10;69(14):422.
[2] Hadayer A, Zahavi A, Livny E, Gal-Or O, Gershoni A, Mimouni K, Ehrlich R. Patients wearing face masks during intravitreal injections may be at a higher risk of endophthalmitis. Retina. 2020 Sep 1;40(9):1651-6.
[3] Zhao F, Li J, Zhang L, Li Z, Na SG. Multi-view face recognition using deep neural networks. Future Generation Computer Systems. 2020 Oct 1; 111:375-80.
[4] Laine S, Karras T, Aila T, Herva A, Saito S, Yu R, Li H, Lehtinen J. Production-level facial performance capture using deep convolutional neural networks. In Proceedings of the ACM SIGGRAPH/Eurographics symposium on computer animation 2017 Jul 28 (pp. 1-10).
[5] Liu Y, Gayle AA, Wilder-Smith A, Rocklöv J. The reproductive number of COVID-19 is higher compared to SARS coronavirus. Journal of travel medicine. 2020 Mar 13.
[6] Feng S, Shen C, Xia N, Song W, Fan M, Cowling BJ. Rational use of face masks in the COVID-19 pandemic. The Lancet Respiratory Medicine. 2020 May 1;8(5):434-6.
[7] Kumar A, Kaur A, Kumar M. Face detection techniques: a review. Artificial Intelligence Review. 2019 Aug;52(2):927-48.
[8] Liu L, Ouyang W, Wang X, Fieguth P, Chen J, Liu X, Pietikäinen M. Deep learning for generic object detection: A survey. International journal of computer vision. 2020 Feb;128(2):261-318.
[9] Wang Z, Wang G, Huang B, Xiong Z, Hong Q, Wu H, Yi P, Jiang K, Wang N, Pei Y, Chen H. Masked face recognition dataset and application. arXiv preprint arXiv:2003.09093. 2020 Mar 20.
[10] Liu W, Wang Z, Liu X, Zeng N, Liu Y, Alsaadi FE. A survey of deep neural network architectures and their applications. Neurocomputing. 2017 Apr 19; 234:11-26.
[11] Matrajt L, Leung T. Evaluating the effectiveness of social distancing interventions to delay or flatten the epidemic curve of coronavirus disease. Emerging infectious diseases. 2020 Aug;26(8):1740.
[12] Khandelwal P, Khandelwal A, Agarwal S, Thomas D, Xavier N, Raghuraman A. Using computer vision to enhance safety of workforce in manufacturing in a post covid world. arXiv preprint arXiv:2005.05287. 2020 May 11.
[13] Ristea NC, Ionescu RT. Are you wearing a mask? Improving mask detection from speech using augmentation by cycle consistent gains. arXiv preprint arXiv:2006.10147. 2020 Jun 17.
[14] Yadav S. Deep learning-based safe social distancing and face mask detection in public areas for covid-19 safety guidelines adherence. International Journal for Research in Applied Science and Engineering Technology. 2020 Jul;8(7):1368-75.
[15] Lal C, Ahmed A, Siyal R, Beejal SK, Aftab S, Hussain A. Text Clustering using K-MEAN. International Journal. 2021 Jul;10(4).
[16] Livanos NA, Hammal S, Nikolopoulos CD, Baklezos AT, Capsalis CN, Koulouras GE, Charamis PI, Vardiambasis IO, Nassiopoulos A, Kostopoulos SA, Asvestas PA. Design and interdisciplinary simulations of a hand-held device for internal-body temperature sensing using microwave radiometry. IEEE Sensors Journal. 2018 Jan 9;18(6):2421-33.
[17] Uçar A, Demir Y, Güzeli? C. Object recognition and detection with deep learning for autonomous driving applications. Simulation. 2017 Sep;93(9):759-69.
[18] Schulz H, Behnke S. Object-class segmentation using deep convolutional neural networks. In Proceedings of the DAGM Workshop on New Challenges in Neural Computation 2011 Aug 30 (pp. 58-61).
[19] Krizhevsky A, Sutskever I, Hinton GE. Imagenet classification with deep convolutional neural networks. Advances in neural information processing systems. 2012;25.
[20] LeCun Y, Bengio Y. Convolutional networks for images, speech, and time series. The handbook of brain theory and neural networks. 1995 Apr;3361(10):1995.
[21] Farabet C, Couprie C, Najman L, LeCun Y. Learning hierarchical features for scene labeling. IEEE transactions on pattern analysis and machine intelligence. 2012 Oct 24;35(8):1915-29.
[22] Ciresan D, Giusti A, Gambardella L, Schmidhuber J. Deep neural networks segment neuronal membranes in electron microscopy images. Advances in neural information processing systems. 2012;25.
[23] Song C, Zeng P, Wang Z, Zhao H, Yu H. Wearable continuous body temperature measurement using multiple artificial neural networks. IEEE Transactions on Industrial Informatics. 2018 Jan 15;14(10):4395-406.
[24] Shagufta A, Chaman L, Suresh Kumar B, Ambreen F. Raspberry Pi (Python AI) for Plant Disease Detection. Int J Cur Res Rev. 14(3), February 2022, 36-42, http://dx.doi.org/10.31782/IJCRR.2022.14307.
[25] Tharun Pranav S V, Anand J. Detection of COVID-19 from Chest X-ray Images using Concatenated Deep Learning Neural Networks. Int J Cur Res Rev. 14(3), February 2022, 53-59, http://dx.doi.org/10.31782/IJCRR.2022.14310.
|