IJCRR - 13(18), September, 2021
Pages: 117-123
Date of Publication: 26-Sep-2021
Print Article
Download XML Download PDF
A Survey on Analysis and Classification of Breast Cancer
Author: Nandish Sonali, Prathibha Ramapura Javaregowda
Category: Healthcare
Abstract:Introduction: The reports obtained after pathological examinations on breast cancer have been digitized by sophisticated machines and stored as Electronic Health Records (EHRs). These EHRs contribute to Computer-Aided Diagnosis and for better clinical decision support. Objectives: Understanding of various machine learning techniques used for the classification of standard and real-time breast cancer datasets. Reviewing of classification of various types of dataset like images, text, numerical values etc., on breast cancer. Methods: In this paper, a rigorous literature survey has been made on the classification of the dataset on breast cancer using various machine learning methods on standard datasets like Breast Cancer Dataset, Wisconsin Breast Cancer Dataset, Wisconsin Breast Cancer Diagnostic Dataset, Wisconsin Breast Cancer Prognostic Dataset and Surveillance Epidemiology and End Results Dataset etc. In the literature, it has been observed that some of the authors have worked on the classification of datasets that are collected from different hospitals. Images of the breast have been analyzed by looking at the property of luminance, colour and shape variation, texture, reaction to biomarkers and many other factors. For understanding proliferation in breast cancer, various scoring systems are used. They include Bloom-Richardson Score, Masood Score, Modified Masood Score, Robinson's Score and many others. The EHRs containing the records in text form on breast cancer have been interpreted using Natural Language Processing approaches like text segmentation, named entity recognition and part of speech tagging etc., and classified using machine learning approaches. Results: Classification of breast cancer has been made on different types of datasets using machine learning methods and the range of accuracy obtained is between 75.60% and 99.86%. Conclusion: Most of the existing classifiers are binary classifiers to classify breast cancer datasets into benign and malignant classes. However, it is necessary to design multiclass classifiers for building a precise clinical decision support system and to provide targeted therapy for cancerous patients using cost-effective diagnostic methods.
Keywords: Breast Cancer, Digital Image Processing, Scoring System, Natural Language Processing, Pathology, Cytopathology, Histopathology
Full Text:
Introduction
Cell division is a normal process in the human body where cells grow old or become damaged and die and new cells take their place.. Breast cancer develops from breast tissue when cells in the region grow out of control.1
Diagnosis and prognosis of breast cancer are very important for its effective clinical management and treatment. It is noted that the lack of proper detection of breast cancer has increased the number of cases in India and the World. Breast cancer affects nearly 34% of women between the age of 20 and 100 years worldwide2 and it is expected to cross 1,00,000 patients annually in India.3
Computer-Aided Diagnosis (CAD) is used to enhance the diagnosis and prognosis of breast cancer. Under pathology, breast cancer detection is mainly based on the cell morphology and architecture distribution. Breast cancer can be classified into benign and malignant by considering nuclei detection, nuclei segmentation and the number of nucleoli using Digital Image Processing.4 The standard approach to analysing the image dataset includes analysis of Haematoxylin (H) & Eosin (E) images.5 Natural Language Processing approaches are used for text report segmentation, clinical information retrieval, part-of-speech tagging, named entity recognition and context extraction.6 We can observe that authors Fushman et al.,7 have discussed that Clinical Decision Systems (CDS) have improved practitioner’s performance by 60% in the reviewed cases of breast cancer. Most commonly, the binary classification is made which divides breast cancer into benign and malignant classes. Another approach used for breast cancer diagnosis is the grading system.8,9,10 A review of the classification of breast cancer on different types of datasets using machine learning approaches is given in the next section.
Methodology
An extensive literature review has been made on the analysis of breast cancer considering Histopathology and Cytology data in numeric, image and text form. The data is obtained from standard datasets, cancer registries and real-time data from various hospitals.
Review on Classification of Breast Cancer using Standard Datasets
Classification of Breast Cancer using Histopathological Numerical Data
Authors Delen et al., have made survival analysis of breast cancer on Surveillance, Epidemiology and End Results (SEER) dataset and classified them into survivability and non-survivability of the patient using Artificial Neural Network (ANN), Decision Tree (DT) and Logistic Regression (LR) approaches and obtained accuracy of 91.2%, 93.6% and 89.2% respectively.11 Authors Qin et al., have analyzed the imbalanced SEER dataset and obtained an accuracy of 76.59% and Area Under the Curve (AUC) of 76.78%, respectively.12 Authors Rajesh et al., have used the C4.5 classification algorithm on SEER dataset to classify patients into either the ‘Carcinoma in situ’ group or ‘Malignant potential’ group and obtained an accuracy of 92.2%.13
Rathore et al., have analyzed SEER dataset by using an ensemble classification approach using DT, Naïve Bayes (NB), Multiple Association Rule for predicting the survivability of breast cancer patients and the best accuracy obtained is 71.87%.14 Swetha Karya has analyzed SEER dataset with DT classifier and achieved a classification accuracy of 93.62% with a sensitivity of 96.02% and a specificity of 90.66%.15 The authors Umesh et al., have analyzed SEER dataset using the association rule mining method and obtained a sensitivity of 56.32%, specificity of 91.78% and accuracy of 87.72%.16,17 The authors Yeulkar et al., have used C4.5 and NB classifier on SEER dataset containing breast cancer samples for the period 1975 till 2013 and obtained an accuracy of 98.1% for C4.5 and 95.85% for NB approach.18
Classification of Breast Cancer using Cytological Numerical Dataset
Various researchers have worked on publicly available standard datasets of University of California Irvine Machine Learning Repository like Breast Cancer (BC) Dataset, Wisconsin Breast Cancer (WBC) Dataset, Wisconsin Breast Cancer Diagnostic (WBCD) Dataset, Wisconsin Breast Cancer Prognostic (WBCP) Dataset with 286, 699, 569 and 198 Samples Respectively.19
BC and WBC dataset
Lavanya et al., have used the Classification and Regression Technique (CART) for analysis on BC and WBC datasets and obtained an accuracy of 69.23% and 94.84% respectively.20 Authors Paulin et al., have analyzed the WBC dataset using Feedforward Neural Network (FNN) to obtain the highest diagnostic performance of 99.26 % using 6 neurons.21 The authors Salama et al., have analyzed the WBC dataset and obtained the highest accuracy of 97.5% by using an ensemble classifier containing five classifiers viz., i) J48, ii) MultiLayer Perceptron (MLP), iii) NB, iv) SVM and v) k-NN classifier with Principal Component Analysis (PCA).22 The authors Inan et al., have analyzed the WBC dataset using a hybrid approach including Apriori Algorithm and PCA together with ANN classifier. They have used 10-fold cross-validation and obtained average classification accuracy of 98.29%.23 Authors Tintu et al., have analyzed the WBC dataset using MLP, SVM, NB, Fuzzy C-Means (FCM) for breast cancer diagnosis. The best accuracy was obtained for FCM with a training accuracy of 97.13 % and a testing accuracy of 98.62%.24 Ravikumar et al., have analyzed the WBC dataset and obtained the best results for the SVM classifier with an accuracy of 97.59%, the sensitivity of 98.10% and specificity of 96.60%.25
The authors Grewal et al., have analysed the WBC dataset and obtained a sensitivity of 95% and specificity of 98.8%.26 The authors Kathija et al., have analysed the WBC dataset by using NB and SVM classifier along with 10-fold cross-validation technique. The best accuracy of 95.6% is obtained with the sensitivity of 97% and specificity of 100% using the NB Classifier.27 Chaurasia et al., have proposed prediction of benign and malignant conditions on standard WBC dataset. The authors have used six classifiers viz., i) NB, ii) RBF, iii) J48, iv) SVM, v) K-NN and vi) RBF tree. The highest accuracy obtained is 97.36% for NB.28
WBCD dataset
Lavanya et al., have used CART for analysis on the WBCD dataset and obtained an accuracy of 92.97%.20 The authors Salama et al., analyzed the WBCD dataset and obtained the highest accuracy of 97.7% by using an ensemble classifier containing SVM and MLP classifier.22 Shweta Karya has analyzed the WBCD dataset using a decision tree classifier and obtained the best accuracy of 93.62%.15 Menaka et al., have analyzed WBCD datasets using SVM with RBF and obtained an accuracy of 97.37% respectively.29
The author Leena Vig has applied SVM, NB and Random Forest (RF) classifiers with 100 decision trees on the WBCD dataset and achieved the best accuracy of 95.64% with a sensitivity of 97 % and specificity of 94 %.30 Hazra et al., have analyzed data by considering only 5 features on 32 features from the WBCD dataset using an ensemble of NB and SVM classifiers and obtained an accuracy of 97.4%.31 The author Agarap has proposed a model with MLP that gave the best performance measure with an accuracy of 99.04%.32
WBCP dataset
Authors Tintu et al., have analyzed the WBCP dataset using MLP, SVM, NB, FCM for breast cancer prognosis and obtained 100% True Positive (TP) and 87% True Negative (TN) rates.24 The authors Wolberg et al., have built a neural network model on the WBCP dataset for prognosis prediction. They obtained a probability that 50% of patients would be disease-free when the period considered for breast cancer recurrence was less than or equal to 5 years from the time of occurrence of cancer and 90% of patients would be disease-free when the period considered was greater than 5 years.34 Senturk et al., have made their analysis on these standard datasets using Rapid Miner 5.0 data mining tool with an accuracy of 98.4%.35
The best performance obtained by various approaches on each type of standard dataset is given in Table 1.
Review on Classification of Breast Cancer using Grades or Scoring System
In recent years, the analysis of breast cancer has been expanded from binary to multiclass classification. Hence, the concept of grading or scoring the lesions has been considered.36 The known methods of grading or scoring include Bloom-Richardson Score (BRS), Modified Bloom-Richardson Score (MBRS), Masood Score (MS), Modified Masood Score (MMS) and many others. Under these methods, the characteristics of breast lesions are measured and an interval of value is fixed with a particular grade or score.37, 38
Classification of Breast Cancer on Histopathological Numerical Dataset
The authors Meyer et al., have classified 631 patients from St. Luke’s Hospital, USA using BR Score and have obtained a kappa statistic of 0.38.39 Rekha et al., have proposed an MBR grading system on 50 breast carcinoma cases from a tertiary centre at Mysore, India and have obtained a histopathological correlation of 86%.40
Classification of Breast Cancer using Cytological Numerical Dataset
Authors Mridha et al., have used Masood’s score on 62 breast cancer patients from the All India Institute of Medical Sciences and obtained specificity for FNAC technique for carcinoma between 89% to 98% and sensitivity between 93% to 98%.41 Nandini et al., have proposed an MMS system to classify 100 lesions samples into four categories with an accuracy of 96%.42 Sheeba et al., have made the comparison of both MS and MMS methods on 100 cases collected at Kilpauk Medical College, Chennai, India. The Cyto-histological correlation is 88% and the accuracy of MMS is 84%.43 The authors Cherath et al. have collected a dataset of 207 cases in a tertiary health centre in South India, to analyse the samples using the MS and MMS approaches and obtained an overall accuracy of 97.5%, the sensitivity of 94.5% and specificity of 100%48. It is also validated that MMS is a better scoring system than MS.44-45
Review on Classification of Breast Cancer using Image Dataset
A computer-Aided Diagnosis (CAD) algorithm has been developed for the detection and prediction of diseases and to assist the pathologist for better clinical decision making. Under pathology, histopathological analysis is considered as the golden standard by pathologists.46
Classification of Breast Cancer using Histopathological Image Dataset
The authors Jelen et al., have used a database that consists of 110 FNA Biopsy (FNAB) images from the University of Wroclaw, Poland. There are 44 images with high malignancy and 66 images with intermediate malignancy. They have used the SVM framework to assign a malignancy grade based on pre-extracted features with an accuracy of up to 94.24%.46 The authors Cosatto et al., have used 208 histopathological images from St Luke's Hospital, Chesterfield, USA, to identify the Cancer Nuclei, using the Hough transform and Active Contour Model for segmentation. The authors have used an SVM classifier for morphology and texture-based classification and obtained 92% of True Positive Rate and 72% of Kappa statistical measure.47 Fatakdawala et al., have considered H&E stained breast biopsy cores at The Cancer Institute of New Jersey. For a total of 62 HER2+ breast biopsy images, the Expectation-Maximisation based segmentation with Geodesic Active Contour with Overlap Resolution (EMaGACOR) was found to have a detection sensitivity of over 90% and a positive predictive value of over 78%.48
The authors Basavanhally et al., have used a total of 41 H&E stained breast biopsy samples from 12 patients at The Cancer Institute of New Jersey, USA. to successfully distinguish the samples of high and low lymphocytic infiltration levels with classification accuracy greater than 90% using SVM Classifier.49
The authors' Beck et al., have developed a Computational Pathologist (C-Path) system to measure a rich quantitative feature set from the breast cancer epithelium and stroma which has 6642 features, from two independent cohorts of breast cancer patients namely the Netherlands Cancer Institute (NCI) cohort, with 248 samples and the Vancouver General Hospital (VGH) cohort, with 328 samples where both cohorts had the value of Probability P ≤ 0.001.50
Wang et al., have done colour recognition by applying a fuzzy inference system and combining RGB and CIE LAB colour space. The approach is used on a standard ICPR12 dataset with the combination of Hand Crafted (HC) features and features derived from Convolutional Neural Networks (CNN). The data has been analyzed using a combination of HC and CNN and obtained an F-Measure of 73.45% .51 Spanhol et al., have classified breast cancer images of Breakhis dataset by using deep features also called DeCAF. DeCAF features are neither HC nor fully automated in nature. They have obtained a classification accuracy of 90%.52 The authors Beevi et al. have proposed a Krill Herd Algorithm to differentiate mitotic and non-mitotic groups. They have obtained a Precision of 62.50% and a Recall of 93.75%.53 Jiang et al., have developed Breast cancer Histopathology image Classification Network (BHCNet) for binary classification of images using Breakhis dataset and obtained performance between 98.87% and 99.34%.54 The authors Dabeer et al., have analyzed 7909 images stained by H&E and paraffin on Breakhis dataset and classified using CNN and obtained an accuracy of 99.86%.55
Review on Classification of Breast Cancer containing Text Data
Electronic Health Records are most commonly available in the form of text data. Text data contains a lot of valuable clinical information to ascertain the exact cause and status of any disease. For diagnosis and prognosis of breast lesions, clinical data in the form of text can be extracted to obtain conclusions about the exact condition of breast cancer using machine learning approaches.56, 57
Classification of Breast Cancer using Histopathological Text Data
The authors Carell et al.,58 have designed an abstraction search for breast cancer recurrence using clinical notes of 1472 patients obtained from Group Health Research Institute, Seattle, USA to identify the recurrence of breast cancer. The clinical Text Analysis and Knowledge Extraction System (cTAKES) method is used for analysis and achieved 93% of sensitivity and 95% of specificity.
Rani et al.,59,60 have used 150 de-identified reports from the Christian Medical College, Vellore, India and proposed a pTNM classifier where T denotes Tumour, N denotes Lymph Node and M denotes Metastases and obtained performance measures for cancer stage was 61.48% for logistic regression and decision tree and 100 % for RF.
NLP-based clinical analysis is carried out by Buckley et al, on breast pathology reports obtained at the Massachusetts General Hospital, USA and obtained had a sensitivity of 99.1% and specificity of 96.53%.61 Authors Zeng et al., have considered a dataset from North Western University Feinberg School of Medicine to retrieve data for pTNM classification and the measurement is made by measuring feature co-efficient. The authors obtained partial sentences from Meta Map with a feature coefficient of 0.66 for recurrent breast cancer and 0.46 for non-infiltrating intra-ductal carcinoma.62
The authors' Ling et al., have used records from Stanford Health Care, USA using regularized logistic regression model for recurrent Metastatic Breast Cancer (MBC) classification on 146 patients. The MBC classifier achieved an AUC of 91.7%.63
Xie et al., have used an end to end NLP technology to process pathology reports. A total of 249 breast cancer cases from the Cancer Registry (CANREG) were considered. The authors have interpreted 437 breast cancer concept terms and 14 combinations of cancer terms to identify terms related to breast cancer and obtained an accuracy of 96%.64
Further, the authors Banerjee et al., have used many NLP modules namely Report Segmentation, Sentence Splitter, Named Entity Tagging and Sentence Selection on the Onco SHARE database. They obtained a sensitivity of 83% and a specificity of 73%.65 In the paper by author Minerd, all the NLP approaches including Rule-based and ML-based approaches for breast cancer on text report analysis has been elaborately reviewed.66
Results
In literature, it is observed that the breast cancer dataset has been analyzed by researchers using various machine learning approaches on standard datasets and obtained the best accuracy of 99.26%. For image data, the best accuracy of 99.86% is obtained by using CNN on 7909 Histopathology images that are collected from Breakhis dataset. Considering the grade or scoring system, the best accuracy of 97.5% is obtained by MMS on 207 samples collected from a tertiary centre in South India. The best accuracy of 96% is obtained by using TIES on 249 reports that are taken from the cancer registry database.
Discussion
From the literature review, it is observed that breast cancer is manifested by abnormal growth of tumours in various parts of the breast. Some of the common areas of tumour growth observed under histopathology include the nipple, areola, lymphocytes, nodes, etc. Under cytology, it is observed by morphological changes in cell, nucleus and nucleoli.
Conclusion
Most of the existing classifiers are binary classifiers to classify breast cancer data into a benign and malignant classes. However, it is necessary to design multiclass classifiers on breast cancer datasets for precise clinical decision support to provide targeted therapy for cancerous patients.
Source of Funding: We hereby declare that there is no funding involved in our work.
Conflict of Interest: Nil
Acknowledgement
The authors are also grateful to authors/editors/publishers of all those articles, journals and books from where the literature for this article has been reviewed and discussed.
Authors’ Contribution
The author Nandish Sonali has contributed by reviewing the papers related to the topic and submitted the inference. The author Prathibha Ramapura Javaregowda has contributed by editing the content of the paper.
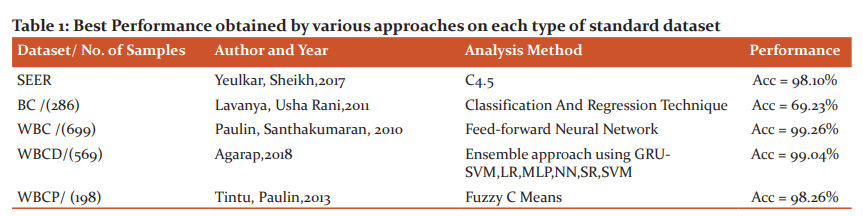
References:
-
Mayo Clinic (US). Breast cancer - diagnosis and treatment; 2020.
-
American Cancer Society (US), Breast cancer Risk and Prevention; 2020.
-
Siegel RL, Miller KD, Jemal A. Cancer statistics 2019. CA Cancer J Clin. 2019 Jan; 69(1):7-34.
-
Veta M, Pluim JP, Van Diest PJ, Viergever MA. Breast cancer histopathology image analysis: A review. IEEE Trans Biomed Eng. 2014 Jan 30; 61(5):1400-11.
-
Blumenthal D, Tavenner M. The “meaningful use” regulation for electronic health records. New Engl J Med. 2010 Aug 5; 363(6):501-4.
-
Ahern TP, Beck AH, Rosner BA, Glass B, Frieling G, Collins LC, et al. Continuous measurement of breast tumour hormone receptor expression: A comparison of two computational pathology platforms. J Clin Pathol. 2017 May 1; 70(5):428-34.
-
Demner-Fushman D, Chapman WW, McDonald CJ. What can natural language processing do for clinical decision support?. J Biomed Inform. 2009 Oct 1; 42(5):760-72.
-
Cross SS. Grading and scoring in histopathology. Histopathol. 1998 Aug; 33(2):99-106.
-
Rakha EA, Reis-Filho JS, Baehner F, Dabbs DJ, Decker T, Eusebi V, et al. Breast cancer prognostic classification in the molecular era: The role of histological grade. Breast Cancer Res. 2010 Aug; 12(4):1-2.
-
Chintalapani SR, Bala S, Konatam ML, Gundeti S, Kuruva SP, Hui M. Triple-negative breast cancer: Pattern of recurrence and survival outcomes. Indian J Med Paediatr Oncol. 2019 Jan 1; 40(1):67-74.
-
Delen D, Walker G, Kadam A. Predicting breast cancer survivability: A comparison of three data mining methods. Artif Intell Med. 2005 Jun 1; 34(2):113-27.
-
Liu YQ, Wang C, Zhang L, editors. Decision tree-based predictive models for breast cancer survivability on imbalanced data. Proceedings of the 3rd international conference on Bioinformatics and Biomedical Engineering; 2009 Jun 11-13. Beijing, China, IEEE.
-
Rajesh K, Anand S. Analysis of SEER dataset for breast cancer diagnosis using C4. 5 classification algorithm. Int J Am Res. 2012 Apr; 1(2):72-7.
-
Rathore N, Tomar D, Agarwal S, editors. Predicting the survivability of breast cancer patients using an ensemble approach. Proceedings of 2014 International Conference on Issues and Challenges in Intelligent Computing Techniques, 2014 Feb 7-8. Ghaziabad, India, IEEE.
-
Karya S. Using data mining techniques for diagnosis and prognosis of cancer disease. Int J Cont Soc Tech. 2012 Apr; 2(2):55-66.
-
Umesh DR, Ramachandra B. Big data analytics to predict breast cancer recurrence on SEER dataset using Map Reduce approach. Int J Contr Ass. 2016 September; 150(7):7-11.
-
Umesh DR, Ramachandra B, editors. Association rule mining based predicting breast cancer recurrence on SEER breast cancer data. Proceedings of 2015 International Conference on Emerging Research in Electronics, Computer Science and Technology; 2015 Dec 17-19.Mandya, India, IEEE.
-
Yeulkar K, Sheikh R. Utilization of Data Mining Techniques for Analysis of Breast cancer Dataset Using R. Int J Res Ass.2017 2(30):406-10.
-
University California Registry (US), Breast Cancer Datasets;2020.
-
Lavanya D, Rani KU. Ensemble decision tree classifier for breast cancer data. Int J Tech Contr Soc. 2012 Feb 1; 2(1):17-26.
-
Paulin F, Santhakumaran A. Back propagation neural network by comparing hidden neurons: Case study on breast cancer diagnosis. Int J Contr Ass. 2010 Jun; 2(4):40-4.
-
Salama GI, Abdelhalim MB, Zeid MA. Experimental comparison of classifiers for breast cancer diagnosis. Proceedings of Seventh International Conference on Computer Engineering & Systems 2012 Nov 27-29.Cairo, Egypt, IEEE.
-
Inan O, Uzer MS, Y?lmaz N. A new hybrid feature selection method based on association rules and PCA for detection of breast cancer. Int J Cont Res. 2013 Feb; 9(2):727-9.
-
Tintu PB, Paulin R. Detect breast cancer using fuzzy C means techniques in Wisconsin prognostic breast cancer (WPBC) data sets. IJCATR. 2013; 2(5):614-7.
-
Kumar GR, Ramachandra GA, Nagamani K. An efficient prediction of breast cancer data using data mining techniques. IntvJ Engg Tech. 2013 Aug; 2(4):42-7.
-
Kaur Grewal R, Pandey B. Two Level Diagnosis of Breast Cancer Using Data Mining. Int J Cont Ass. 2014 Mar; 89(18):975-978.
-
Kathija A, Shajun N. Breast cancer data classification using SVM and Naive Bayes techniques. Int J Res Contr Engg. 2016 Dec; 4(12):21167-75.
-
Chaurasia V, Pal S. Data mining techniques: To predict and resolve breast cancer survivability. Int J Cont Soc Med Conf. 2014 Jan 29; 3(1):10-22.
-
Menaka K, Karpagavalli S. Breast cancer classification using support vector machine and genetic programming. Int J Res Engg. 2013 Sep; 1(7):1-8.
-
Vig L. Comparative analysis of different classifiers for the Wisconsin breast cancer dataset. OALJ. 2014 Sep 1; 1(6):1-7.
-
Hazra A, Mandal SK, Gupta A. Study and analysis of breast cancer cell detection using Naive Bayes, SVM and ensemble algorithms. Int J Cont Ass. 2016; 145(2):39-45.
-
Agarap AF, editors. On breast cancer detection: an application of machine learning algorithms on the Wisconsin diagnostic dataset. Proceedings of the 2nd International Conference on Machine Learning and Soft Computing; 2018 Feb 2-4; Phu Quoc Island, Viet Nam. Association for Computing Machinery, New York, USA, 2018.
-
Chaurasia V, Pal S, Tiwari BB. Prediction of benign and malignant breast cancer using data mining techniques. J Am Contr Tech. 2018 Jun; 12(2):119-26.
-
Wolberg WH, Street WN, Heisey DM, Mangasarian OL. Computerized breast cancer diagnosis and prognosis from fine-needle aspirates. Arch Surg. 1995 May 1; 130(5):511-6.
-
Senturk ZK, Kara R. Breast cancer diagnosis via data mining: performance analysis of seven different algorithms. Comp Sci Eng. 2014 Feb 1; 4(1):35-46.
-
Elston CW, Ellis IO. Pathological prognostic factors in breast cancer. I. The value of histological grade in breast cancer: experience from a large study with long?term follow?up. Histopathol. 1991 Nov; 19(5):403-10.
-
Abraham B, Sarojini TR. Cytological scoring of breast lesions and comparison with histopathological findings. J Cytol. 2018 Oct; 35(4):217-22.
-
Srinivasan R, Rekhi B, Rajwanshi A, Pathuthara S, Mathur S, Jain D, et al. Indian academy of cytologists guidelines for collection, preparation, interpretation, and reporting of serous effusion fluid samples. J Cytol. 2020 Jan; 37(1):1-11.
-
Meyer JS, Alvarez C, Milikowski C, Olson N, Russo I, Russo J, et al. Breast carcinoma malignancy grading by Bloom–Richardson system vs. proliferation index: Reproducibility of Grade and Advantages of Proliferation Index. Mod. 2005 Aug; 18(8):1067-78.
-
Rekha TS, Nandini NM, Dhar M. Expansion of Masood's Cytologic index for breast carcinoma and its validity. J Cytol. 2013 Oct; 30(4):233-6.
-
Mridha AR, Iyer VK, Kapila K, Verma K. Value of the scoring system in classification of proliferative breast disease on fine-needle aspiration cytology. Indian J Pathol Microbiol. 2006 Jul; 49(3):334-8.
-
Nandini NM, Rekha TS, Manjunath GV. Evaluation of scoring system in cytological diagnosis and management of breast lesion with the review of the literature. Indian J Cancer. 2011 Apr 1; 48(2):240-5.
-
Sheeba D, Chitrakala S. Palpable Breast Lesions: Cytomorphological Analysis and Scoring System with Histopathological Correlation. Int J Dent Med Sci. 2016 Oct; 15(10):25-9.
-
Cherath SK, Chithrabhanu SM. Evaluation of Masood’s and Modified Masood’s Scoring Systems in the Cytological Diagnosis of Palpable Breast Lump Aspirates. J Clin Diagn Res. 2017 Apr; 11(4):EC06-11.
-
Agarwal C, Chauhan V, Pujani M, Singh K, Raychaudhari S, Singh M, et al. Masood’s and Modified Masood’s Scoring Index: An Evaluation of Fine Needle Aspiration Cytology of Breast Lesions with Histopathological Correlation. Acta Cytol. 2019; 63(3):233-9.
-
Jele? L, Fevens T, Krzy?ak A. Classification of breast cancer malignancy using cytological images of fine-needle aspiration biopsies. Int J Appl Math Comput Sci. 2008 Mar 1; 18(1):75-83.
-
Cosatto E, Miller M, Graf HP, Meyer JS, editors. Grading nuclear pleomorphism on histological micrographs. Proceedings of 19th International Conference on Pattern Recognition 2008 ;8:1-4.Tampa, Florida, USA, IEEE.
-
Fatakdawala H, Xu J, Basavanhally A, Bhanot G, Ganesan S, Feldman M, et al. Expectation–maximization-driven geodesic active contour with overlap resolution (EMaGACOR): Application to lymphocyte segmentation on breast cancer histopathology. IEEE Trans Biomed Engg. 2010 Feb 17; 57(7):1676-89.
-
Basavanhally AN, Ganesan S, Agner S, Monaco JP, Feldman MD, Tomaszewski JE, et al. Computerized image-based detection and grading of lymphocytic infiltration in HER2+ breast cancer histopathology. IEEE Trans Biomed Engg. 2009 Oct 30; 57(3):642-53.
-
Beck AH, Sangoi AR, Leung S, Marinelli RJ, Nielsen TO, Vijver MJ, et al. Systematic analysis of breast cancer morphology uncovers stromal features associated with survival. Sci. Transl. Med. 2011 Nov 9; 3(108):108-13.
-
Wang H, Roa AC, Basavanhally AN, Gilmore HL, Shih N, Feldman M, et al. Mitosis detection in breast cancer pathology images by combining handcrafted and convolutional neural network features. J Med Imaging. 2014 Oct; 1(3):0340031-8.
-
Johri P, Sen Saxena V, Kumar A. Rummage of Machine Learning Algorithms in Cancer Diagnosis. Int J Health Med. 2021; 12(1):1-5.
-
Beevi KS, Nair MS, Bindu GR. A multi-classifier system for automatic mitosis detection in breast histopathology images using deep belief networks. IEEE J Transl Eng Health Med. 2017 Apr 25; 5:1-7.
-
Jiang Y, Chen L, Zhang H, Xiao X. Breast cancer histopathological image classification using convolutional neural networks with small SE-ResNet module. PloS one. 2019 Mar 29; 14(3):65-74.
-
Dabeer S, Khan MM, Islam S. Cancer diagnosis in the histopathological image: CNN based approach. Med Inform. 2019 Jan 1; 16:1-5.
-
Hughes KS, Zhou J, Bao Y, Singh P, Wang J, Yin K et al. Natural language processing to facilitate breast cancer research and management. Breast J. 2020 Jan; 26(1):92-9.
-
Datta S, Bernstam EV, Roberts K. A-frame semantic overview of NLP-based information extraction for cancer-related EHR notes. J Biomed. Inform 2019 Dec 1; 100:1-39.
-
Carrell DS, Halgrim S, Tran DT, Buist DS, Chubak J, Chapman WW, et al., Using natural language processing to improve the efficiency of manual chart abstraction in research: the case of breast cancer recurrence. Am J Epidemiol.2014 Mar 15; 179(6):749-58.
-
Gladis D, Manipadam MT, Ishitha G, editors. Breast cancer staging using natural language processing. Proceedings of 2015 International Conference on Advances in Computing, Communications and Informatics; 2015 Aug 10-15, Kochi, India.
-
Rani GJ, Gladis D, Mammen J, editors. Classification and Prediction of Breast Cancer Data derived Using Natural Language Processing. Proceedings of the Third International Symposium on Women in Computing and Informatics 2015 Aug 10-15. Kochi, India.
-
Buckley JM, Coopey SB, Sharko J, Polubriaginof F, Drohan B, Belli AK, et al. The feasibility of using natural language processing to extract clinical information from breast pathology reports. J Pathol. Inform. 2012; 3(1)1-8.
-
Ling AY, Kurain AW, Caswell-Jin JL, Sledge Jr GW, Shah NH, Tamang SR, et al. Using Natural Language Processing to Construct a Metastatic Breast Cancer Cohort from Linked Cancer Registry and Electronic Medical Records Data. J Am Med Ass. 2019 Dec;2(4):528-37.
-
Zeng Z, Espino S, Roy A, Li X, Khan SA, Clare SE, et.al. Using natural language processing and machine learning to identify breast cancer local recurrence. BMC Bioinform. 2018 Dec; 19(17):65-74.
-
Xie F, Lee J, Munoz-Plaza CE, Hahn EE, Chen W. Application of Text Information Extraction System For Real-Time Cancer Case Identification In An Integrated Healthcare Organization. J Pathol Infor. 2017 Jan; 8(48); 1-15.
-
Banerjee I, Bozkurt S, Caswell-Jin JL, Kurain AW, Rubin DL. Natural Language Processing Approaches to Detect the Timeline Of Metastatic Recurrence Of Breast Cancer. JCO Clin. Cancer Inform. 2019 Oct; 3:1-2.
-
Minerd J. A Novel Strategy for Mining Medical Records. Recent Res.2020.
|