IJCRR - 13(12), June, 2021
Pages: 226-232
Date of Publication: 22-Jun-2021
Print Article
Download XML Download PDF
An Improved Firefly Algorithm Integrated with Recurrent Neural Network (RNN) for Face Recognition
Author: Indranil Lahiri, Hiranmoy Roy
Category: Healthcare
Abstract:Background: Face recognition (FR) is a promising biometric trait widely used for authentication in several applications like finance, military, security, surveillance and so on in daily life. Deep learning involves several processing layers for learning data representations with feature extraction at multiple levels. Hence, deep FR techniques with hierarchical architecture which puts pixels together to represent invariant face has drastically improved the recognition performance and promoted real-time applications successfully. Objective: In this research, an Improved FireFly (IFF) algorithm is developed to recognize face whose performance is estimated by the integration of RNN network. Method: Based on the selected dataset, RNN involved in analysis of facial features with inclusion of improved firefly algorithm (RnnIFF). Result: The results stated that proposed approach provides higher value of accuracy, precision and sensitivity expressing 90%, 90% and 91% respectively. Also, Mean Square Error (MSE) and Peak Signal to Noise ratio (PSNR) is evaluated and comparatively examined with existing techniques. The simulation results illustrated that proposed RnnIFF exhibits significant performance for recognition of faces.
Keywords: Recurrent Neural Network, Improved Firefly, Facial parts, Facial features, Classification, Peak signal to noise ratio, Alexnet, Convolutional neural networks
Full Text:
Face recognition (FR) is extensively used in applications like security and financial sectors, say electronic payments. In the recent decades, FR has attracted the researchers. At the beginning, several classical approaches encountered bottlenecks due to some limitations like computing power and capability of the model designed.1,2 As recurrent neural networks (RNNs) were introduced and due to the increase in hardware capability, several limitations were eliminated rapidly, and thus various FR approaches based on RNNs were developed.3The fundamental concepts of FR are feature extraction and their classification. Feature extraction discovers several discriminative features from the input which helps the classifiers to have a greater impact on recognition rate.
In the training set, due to the redundant facial images, the samples are exemplified by the low dimensional features extracted from face.4 By using these extracted features, computational cost is reduced and recognition rate of the classifiers is improved.
For real-time applications, changes in illumination, occlusions, facial expressions and varying pose are noted in a probe which makes the extracted features inefficient. An image can be represented effectively by the process of segmentation which retains the informative regions of the image. Neural network based approaches can provide better classification accuracy. But, these methods are in need of numerous training samples. Further, it is believed that few feature extraction techniques namely Principle Component Analysis (PCA),5 Independent Component Analysis (ICA)6 and Linear Discriminate Analysis (LDA),7 contribute less towards classifications, as they are linear representation approaches. Hence, there arises a necessity to develop a classifier for the FR system that uses the more discriminative information of the probe image is varying and corrupted situations.8
Generally, the less number of grayscale or color images limits the discriminating ability of FR system. Moreover, with PCA, Gabor filter or Support Vector Machine (SVM), spatial parameters extracted from grayscale or color images, are poor if the image has pose and illumination variations and hence thereby results in low accuracy. Many researchers use hyperspectral imaging facial datasets as they have large volume of images.9 Hyperspectral imaging approach provides better FR results by capturing spectral features from face images which provide additional information for classification. The accuracy of the image is increasedwhich depends on the spatial based approaches; moreover, the facial space distance areminimized by inter-object distance. At this circumstance, hyperspectral imaging approach helps to improve the performance as several features were used.10
The traditional deep FR system generally aligns the faces first usingsimple affine transformations which is then fed into convolutional neural networks (CNNs) for extracting identity-preserving features. As this transformation removes only pose variations related to in-plane, still out-plane pose variations exists thereby causes misalignments in faces. Consequently, the accuracy of the FR system is very low when out-plane pose variations are more. To deal with this issue, one of the two following options can be used; aligning images with some additional technology like 3D face alignment11or improving the capability of CNN’s to extract pose-invariant features.11
LITERATURE REVIEW
After investigating the face recollection approaches of image processing, it is found that few methods were successful and those are described here below.12 In the visible light destination approach was introduced for identifying the visual system, least squares were employed for grouping the various poses. The approach was liable to noise. In large-scale face recognizer was suggested which was capable of respondingto the complexity of document asymmetry. For producing, productive universal dataset, a novelapproach was presented image recognition by incorporating fluctuations of data where more effectiveusefulfeatures were extracted.13
In a novel approach to authenticate face was developed which was capable to handle a various pose combinations.14,15 a mutual Bayesian adaptation approach was suggested for modifying GMM to precisely predict the inconsistencies in facial expressions. In a broad space gradient inclusion method which focused on a novel Frenet picture to accept 3-D silhouette-invariant face and posture. Additionally, definedonly one image in the permanent collection to implement a novel approach to detect face with smiling as well as appearance patterns. 16 A 3D Stochastic Facial Emotion Recognition Synthetic Adjustable model was designed and implemented for repeating a 3D method from the original human image using a full 2D anterior imageeitherwith expressions or with no expressions. In the near future, the envisaged FR system can be used inuncontrolledface recognition which is stable to a wide range of patterns related to faces. In an auto encoder was introduced which had the ability to produce a strong-level ethnicity characteristicsfrom posture inconsistencies. Next, empowered personality featuresbydisplacing mechanical auto encoders’ target principles to generic transmissions. A tri-task CNN was considered for facial recognition in which classification and identification were the major role and other functions were projections of illuminations and poses.18 In changes within the image were examined based onthepixel density, amplification etc.,and revealed a training approach for compensating body transition.17
PROPOSED METHODOLOGY
An overview of the proposed approach for identification and recognition of facial features is discussed in this section. To enhance the recognition rate, the proposed system incorporates facial parts and features included for facial recognition. Figure 1 illustrates the working of the proposed RnnIFF for facial recognition.
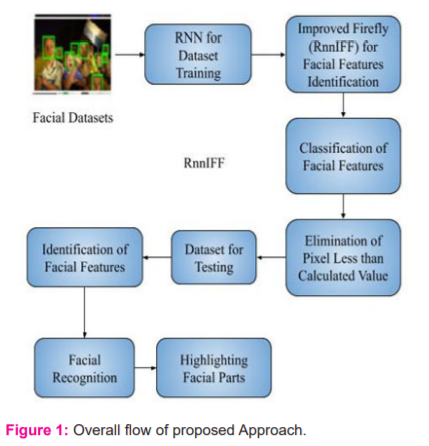
Based on the framed phases, collected facial images to recognize are processed and examined to estimate the performance of the proposed RnnIFF method comparatively with other related methods. The following section explains the proposed approach in detail.
Proposed RNN with Improved FireFly (RnnIFF)
RnnIFF comprises of three stages namely partness map generation, candidate window ranking using the faceness scores, face proposal refinement to detect face. In the beginning, as demonstrated in Figure2,the five layers of RNNs takes a full image x as input. To reduce the computational time, deep layers are shared by all these RNNs. At the top convolutional layer, by averaging, the weights of all the label maps, every RNN generates a partness map. Every partness maps specifies the position of a particular component in the facial image like eyes, mouth, nose, hair and beard, represented as he, hm, hn, ha and hb respectively. All of the abovepartness maps are combined as a face label map hf which specifies the locations of the face clearly.
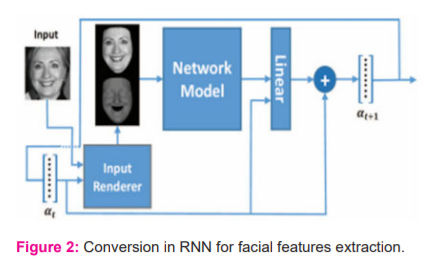
In the second stage, windows are ranked based on their faceness scores. These scores were extracted from partness maps relating to the configurations of the various facial parts, as depicted in Figure 2. As an example, considering as in Figure 3, the local region of ha is covered by the candidate window applied in convolution layer 1–7whose faceness score is obtained by dividing the upper part values with its lower part values. This is because hair is present at the top region of face. The final faceness score is the average of all the scores of these parts.
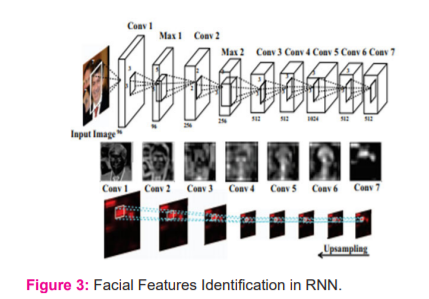
In this case, there is a vast possibility to prune numerous false positive windows. Particularly, this proposed approach has the ability to cope with face occlusions, as visualized in Figure 3, where windows ‘A’ and ‘E’ are obtained by objectness19 only when several windows are projected, whereas their rank is in top 50 when this method is utilized. Finally, multitask CNN is trained to refine the proposed candidate windows, where both the bounding box regression and classification of face are optimized.
Improved FireFly (IFF)
Firefly algorithm (FA) is a familiar stochastic approach for optimization which was introduced by Liu20. This algorithm depends on the illumination of firefly where most of them are bright. This illuminations helps in attracting the prey and opposition. Each firefly sends illumination signals to other firefly. Generally, FA is based on attractiveness and brightness21 which are familiar rules of FA.
-
As fireflies are unisex, it attracts all other fireflies irrespective of sex.
-
The Attraction between two fireflies is directly proportional to Intensity of light or luminance hence brighter ones are more attractive. Firefly having low light intensity moves towards brighter ones.
-
Brightness of the firefly is achieved through cost function or fitness function which is used for searching purpose.
Mathematically, FA is represented as follows. Brighter firefly j attracts firefly i whose movement is obtained by
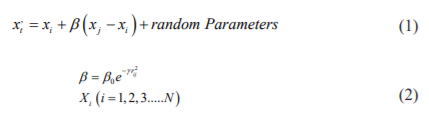
Where, xi and xj represent the position of firefly i and firefly j respectively. is the updated position and
is the initial position of firefly. ϒ is considered as attractive force between firefly.
is the relative distance between two fireflies.
Multilevel thresholding for the grayscale image is a very challenging task. Metaheuristic algorithm can be used to obtain the threshold value with-in range [0, L-1]. Firefly is one of the best metaheuristicalgorithm for maximizing the entropy measure of histogram. FA can be effectively used with levy flight to find optimum threshold value.
Step 1: Generate the population
randomly within the range.
Step 2: Define the Kapoor’s Entropy method as Objective function.
Step 3: Initialize Absorption coefficient ϒ, Maximum Attraction β0, Step Size as levy Flight, maximum iteration
Step 4: Calculate the fitness value for each firefly using

Step 5: Firefly updates its position towards brighter one using

Step 6: Repeat step 3 to 5 until maximum iteration is reached.
Step 7: Estimate the optimum threshold value with which the facial parts are segmented.
Among several segmentation evaluation metrics Peak-signal-to-noise ratio (PSNR) provides significant performance measure. Moreover, computational time and values of objective function are also used as parameters to determine the quality of the image segmented. Usually, PSNR is utilized for approximating the supremacy of the image and the relativity between the original and segmented image.
Facial Part Identification
A deep network which is trained on common objects, for exampleAlexNet16, is unable to provide precise location of faces. There exists several ways to learn partness maps but the most direct one is using the image as input and its pixelwise segmentation label map as target which is broadly used in image labeling5. Another is classifying faces and non-faces at image-level which is well suited for training images that are well-aligned.
However, complex background disorderoccurs as the supervisory information is insufficient for face variations. The feature maps with morenoiseoverwhelm the original position of faces. As an example, an ‘Asian’ face is differentiated from that of a ‘European’. As the attributes of hair are related, they are grouped together. Likewise the other regions too as in Table 1.Various CNNs are involved in modeling different facial regions. Thereby, if one region is occluded, the other regions can be identified by CNNs.
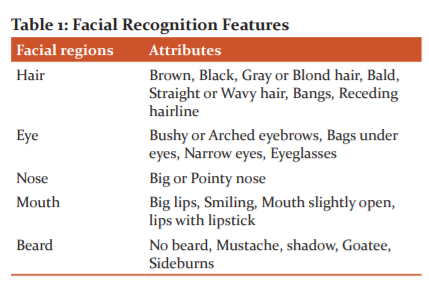
Face Detection
The windows proposed for this approach achieved by faceness have a greater recall. For further improving it, these windows are refined by face classification and bounding box regression with the help of RNN whose function is similar to AlexNet16. Particularly, AlexNetis fine-tuned using AFLW and PASCAL VOC 2007 face images21. For classification, the window is assigned a positive label and the ground truth bounding box is greater than 0.5; or else negative. For false positive values, RNN produces a vector [−1, −1, −1, −1].
EXPERIMENTAL ANALYSIS
Training datasets: CelebFaces dataset is used for training attribute-aware networks with 87,628 web-based images. All the images are labelledwith 25 facial attributes which are divided into five categories as in table 1. Around 75, 000 images were randomly selected for training while the rest was reserved for validation. For detecting face, 13,000facial images were chosen from AFLW dataset with pose variations and 5,700 images from the PASCAL VOC 2007 dataset. From LFW dataset, 2,900 images were selected as it manually provides hair as well as beard superpixel labels. With 68 dense facial landmarks, boxes for eye, nose and mouth are labeled manually.
Intersection over Union (IoU) was used as a metric for evaluating the algorithm. IoU threshold is fixed to 0.5. Particularly, an object is detected whenIoUis more than 0.5. Detection rate, precision and recall were involved to evaluate the effectiveness of the algorithm.Figure 4 presentsthe overall flow of proposed RnnIFF in face recognition. In figure 5, faces identified from the available dataset are presented.
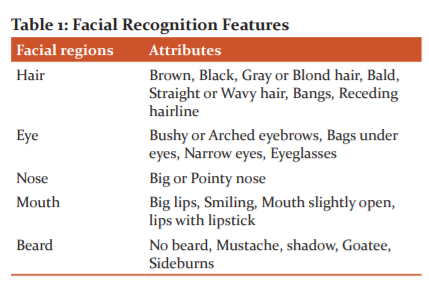
Figure 4: Face Recognition Process in RnnIFF
Figure 4, 5 illustrates that this approach significantly outperforms conventional approaches. In table 2, parameters measured for various input images like accuracy, precision and specificity are presented.
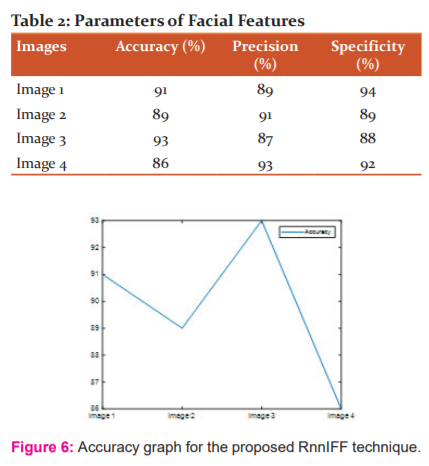
The figure 6 shows the accuracy calculated for the entire input image. X- axis gives the image representation and Y-axis gives accuracy measurement. It denotes that accuracy for proposed technique ranges between 85 and 90. This is the optimized accuracy attained by our proposed technique.
The figure 7 shows the precision calculated for the input images. X-axis gives the image representation and Y-axis gives precision measurement. It denotes that precision for proposed technique ranges between 85 and 95. This is the optimized precision attained by our proposed technique.
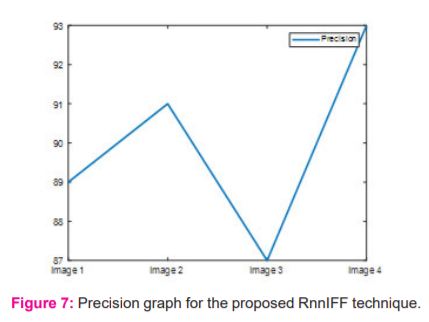
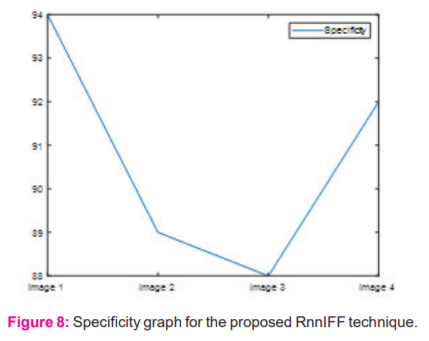
The above figure 8 shows the specificity calculated for the entire input image. X- axis gives the image representation and Y-axis gives specificity measurement. It denotes that specificity for proposed technique ranges between 85 and 90. This is the optimized specificity attained by our proposed technique.
Partial occlusion are explicitly handled in this approach by gatheringthe face likeliness via part responses. The speed was achieved by sharing the layers from conv1 to conv5 as part responses of the face were captured only in the layer conv7 as depicted in figure 2.The speed of this approach is comparatively lower than19. Particularly, this method shows that a CNN structure enjoys a 2.5× speedup without accuracy loss. This method is also benefited from the latest model compression technique8. In table 3 PSNR measured for different images are presented.
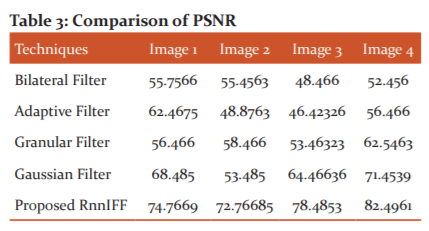
In table 2 comparative PSNR values of existing filters and proposed RnnIFF facial recognition method is presented. The observed results demonstrated that proposed RnnIFF approach exhibits higher PSNR value rather than conventional filtering concept. The performance of RnnIFF technique for all 4 images are 20% higher than that of the conventional filtering facial recognition technique.
From figure 9, it is observed that the proposed RnnIFF exhibits higher PSNR value than bilateral filter, adaptive filter, granular filter and Gaussian filter. In table 3 MSE measurement of the existing and proposed RnnIFFapproaches are presented.
The above table 4 illustrates the MSE performance of existing filtering and the proposed RnnIFF approaches. The RnnIFF approach provides a minimal MSE value of 0.02466 , 0.046656, 0.0058665 and 1.79666 for images 1 to 4 through which it is considered that proposed RnnIFF approach exhibits superior performance rather than existing techniques.In figure 10, comparesthe proposed RnnIFF with the existing bilateral filter, adaptive filter, granular filter and Gaussian filter.
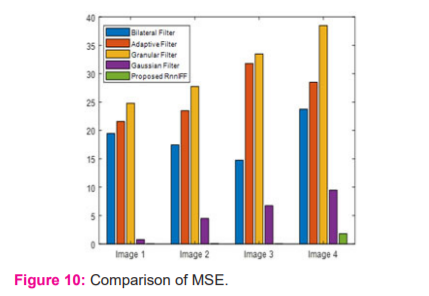
From figure 10, it is observed that proposed RnnIFF exhibits superior performance with minimal MSE value compared with existing techniques.
CONCLUSION
Facial recognition has been utilized in vast range of application due to its security features. In the past, approaches based on neural network were widely applied to locate faces. In this paper, a RNN based approach for facial recognitionis proposed. The proposed approach incorporated RNN with improved firefly algorithm (RnnIFF) for identification of faces. By identification of facial parts, the proposed RnnIFF approach estimated the facial features to perform facial recognition. The evaluation of proposed RnnIFF exhibited that through significant training and testing process facial features were effectively identified. The proposed RnnIFF achieved the accuracy level of 90%, precision value of 90% and specificity value of 91%. The comparative analysis of PSNR and MSE with existing techniques states that the proposed RnnIFF expressed higher PSNR level of approximately 20% and MSE of approximately 10%. The facial features of image expressed that proposed RnnIFF provides improved performance rather than conventional techniques.
ACKNOWLEDGMENT
Authors acknowledge the immense help received from the scholars whose articles are cited and included in references to this manuscript. The authors are also grateful to authors / editors / publishers of all those articles, journals, and books from which the literature for this article has been reviewed and discussed.
Conflict of Interest: Nil
Source of Funding: Nil
Author’s Contribution:
I / We both have equally contributed to this article interms of data collections and research methodologies
References:
-
AbdAlmageed W, Wu Y, Rawls S, Harel S, Hassner T, Masi I and Nevatia R, “Face recognition using deep multi-pose representations IEEE Winter Conference on Applications of Computer Vision (WACV), pp. 1-9, 2016.
-
Agarwal A, Singh R, Vatsa M and Ratha N, “Are image-agnostic universal adversarial perturbations for face recognition difficult to detect?”,IEEE 9th International Conference on Biometrics Theory, Applications and Systems (BTAS), pp.1-7, 2018.
-
Antipov G, Baccouche M and Dugelay J. L, “Boosting cross-age face verification via generative age normalization”, IEEE International Joint Conference on Biometrics (IJCB), pp.191-199, 2017.
-
Bansal A, Castillo C, Ranjan R and Chellappa R, “The do's and don'ts for cnn-based face verification”, Proceedings of the IEEE International Conference on Computer Vision Workshops, pp.2545-2554, 2017.
-
Bao J, Chen D, Wen F, Li H and Hua G, “Towards open-set identity preserving face synthesis”, Proceedings of the IEEE Conference on Computer Vision and Pattern Recognition, pp.6713-6722, 2018.
-
Cao Q, Shen L, Xie W, Parkhi O. M and Zisserman A, “Vggface2: A dataset for recognising faces across pose and age”, 13th IEEE International Conference on Automatic Face & Gesture Recognition (FG 2018),pp. 67-74, 2018.
-
Cao K, Rong Y, Li C, Tang X and Change Loy C, “Pose-robust face recognition via deep residual equivariant mapping”, Proceedings of the IEEE Conference on Computer Vision and Pattern Recognition, pp.5187-5196, 2018.
-
Chen B, Deng W and Du J, “Noisy softmax: Improving the generalization ability of dcnn via postponing the early softmax saturation”, Proceedings of the IEEE Conference on Computer Vision and Pattern Recognition, pp.5372-5381, 2017.
-
Deng W, Hu J and Guo J, “Compressive binary patterns: Designing a robust binary face descriptor with random-field eigenfilters”, IEEE transactions on pattern analysis and machine intelligence, vol.41, no.3, pp.758-767, 2018.
-
Deng W, Hu J and Guo J, “Face recognition via collaborative representation: Its discriminant nature and superposed representation”, IEEE transactions on pattern analysis and machine intelligence, vol.40, no.10, pp.2513-2521, 2017.
-
Kotia J, Bharti R, Kotwal A and Mangrulkar R, “Application of Firefly Algorithm for Face Recognition”, Applications of Firefly Algorithm and its Variants, pp.147-171, 2020.
-
Duong C. N, Quach K. G, Jalata I, Le N and Luu K, “Mobiface: A lightweight deep learning face recognition on mobile devices”, arXivpreprint arXiv:1811.11080, 2018.
-
Goel A, Singh A, Agarwal A, Vatsa M and Singh R, “Smartbox: Benchmarking adversarial detection and mitigation algorithms for face recognition”, IEEE 9th International Conference on Biometrics Theory, Applications and Systems (BTAS) , pp.1-7, 2018.
-
Goswami G, Ratha N, Agarwal A, Singh R and Vatsa M, “Unravelling robustness of deep learning based face recognition against adversarial attacks. Thirty-Second AAAI Conference on Artificial Intelligence, 2018.
-
He L, Li H, Zhang Q Sun Z, “Dynamic feature learning for partial face recognition”, IEEE Conference on Computer Vision and Pattern Recognition (CVPR), 2018.
-
Howard A. G, Zhu M, Chen B, Kalenichenko D, Wang W, Weyand T and Adam H, “Mobilenets: Efficient convolutional neural networks for mobile vision applications”, arXiv preprint arXiv:1704.04861, 2017.
-
Hu L, Kan M, Shan S, Song X and Chen X, “LDF-Net: Learning a displacement field network for face recognition across pose”, 12th IEEE International Conference on Automatic Face & Gesture Recognition (FG 2017), pp.9-16, 2017.
-
Lezama J, Qiu Q and Sapiro G, “Not afraid of the dark: Nir-vis face recognition via cross-spectral hallucination and low-rank embedding”, Proceedings of the IEEE conference on computer vision and pattern recognition, pp.6628-6637, 2017.
-
Li Q, Jin S and Yan J, “Mimicking very efficient network for object detection”, Proceedings of the IEEE Conference on Computer Vision and Pattern Recognition, pp.6356-6364.
-
Liu W, Wen Y, Yu Z and Yang M, “Large-margin softmax loss for convolutional neural networks”, International Conference on Machine Learning, vol.2, no.3, 2016.
-
Liu W, Zhang Y. M, Li X, Yu Z, Dai B, Zhao T and Song L, “Deep hyperspherical learning”, Advances in neural information processing systems, pp.3950-3960, 2017.
|