IJCRR - 13(12), June, 2021
Pages: 107-111
Date of Publication: 22-Jun-2021
Print Article
Download XML Download PDF
A Study on Activity Recognition Technology for Elderly Care
Author: Hemalata Vasudavan, Sumathi Balakrishnan, Raja Kumar Murugesan
Category: Healthcare
Abstract:Background: It is a global challenge to assist the ever-growing elderly populations' needs. There are needs related to healthcare, ageing in place, security, social and well-being of the elderly. Activity recognition enables continuous monitoring and prevents a hazardous situation such as falls. Objective: This paper discusses the type of sensors and processes involved to support the elderly to stay in their home independently and continue their routine activities. Although this technology has been around for a long time, it has not been implemented in elderly homes. Conclusion: This multi-level processing system has many benefits if the data is processed to fit the needs of the resident ambient environment. However, the majority of the research detects single user environment almost perfectly. This paper focuses its attention on research on composite activities and multi-users in an ambient intelligent environment. In addition to that, there are also discussions on the benefit of activity recognition to the elderly, healthcare-related Activity Recognition (AR) and the technology supporting AR.
Keywords: Activity Recognition, Multi-user, Multi-inhabitant, Smart Home technology, Ambient Assisted Living, Elderly
Full Text:
INTRODUCTION
According to the United Nations (2017), it is estimated that the 65 and over age group across several different countries will rise to 28% by 2050. Figure 1 shows 2016 statistic in Malaysia, revealing that 6% of the 31.7 million population is elderly and further predicted that by the year 2030, the elderly population will make up to 15% of the total population.1
The expectancy age of Ageing for various regions such as African, Eastern Mediterranean, European, Western Pacific, Americas, South East Asia and other parts of the world from 1950 to 2050 all shows a definite increase in the numbers.2
Elderly Centered Activity Recognition
With the increase in the elderly population, issues such as the quality of life, prolonged independent living, reducing caregivers’ time and healthcare costs should be tackled carefully. This research discusses activity recognition (AR) environment and Ambient Assisted Living (AAL). Currently, there are many smart technologies developed to enhance the lifestyle of assisted elderly including their safety, security and surveillance, healthcare, social connectedness, social isolation, wealth management services, and many others. In recent research, services for the methods and applications have been classified into five which are Health
Monitoring, Safety Monitoring, Nutrition Monitoring, Social Network, Localization, and Navigation (Figure 1).3 All of these elements are important and includes some aspects of outdoor and indoor requirements.4
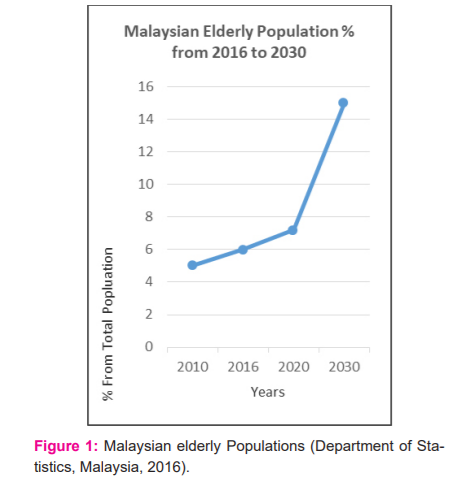
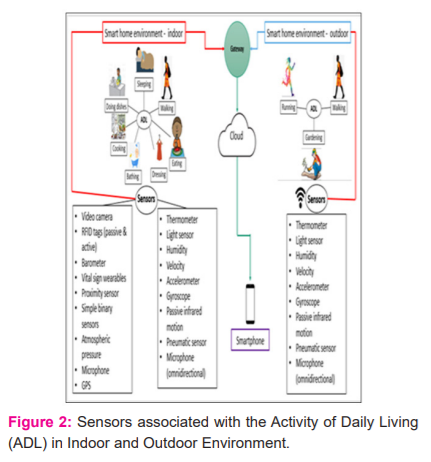
These environments are found to be smartly assisting the elderly and at the same time give great relief for the next of keens and caretakers of the elderly as it can provide support in terms of supervision, alerting in case of emergency, and prevention of potential fatality. The principles of AAL is similar to smart home technologies except that it is skewed to monitoring special conditioned residents such as the disabled and elderly.2 This intelligent environment allows the elderly to Age in Place (AIP). Figure 2 shows an example of multiple sensors sensing the Activity of Daily Living (ADL) in an indoor and outdoor environment. Many research proved that using multiple sensors increases the accuracy of recognizing types of activity. Some of the most common sensors found in smart homes are accelerometer which detects object’s motion and movement, gyroscopes, on the other hand, supports to find objects rotations and magnetometers sensors detect the strength of magnetic fields.
As AR can help to fix these issues that can help facilitate seamless monitoring services in their own homes which indirectly helps to delay ageing as the elderly are very comfortable in their own space.3,4 Opportunity to recover from illness in their own home leaves positive impacts. This also can be a benefit to their mental wellness. Figure 3 shows a statistic on the living arrangement of ageing in Japan. Such statistic for other regions and other latest report was difficult to trace but Japan is always advanced in this area as their percentage of the ageing population has increased tremendously.5
These statistics prove that a significant increase in living with spouse alone, living alone and staying in old folks homes. To be able to receive better and necessary assistance in their own home and home care centres, the current acceptance of the activity recognition model needs to be explored thoroughly.
HEALTHCARE-RELATED ACTIVITY RECOGNITION
Several authors have recognized the potential of a Human Activity Recognition (HAR) system that specializes in identifying patterns and expert systems.6 This approach would improve the cost of services for healthcare which eventually takes off the burden of the elderly.7 Healthcare systems are needed to ensure the life of the elderly remain as smooth as their younger days and minimizing their suffering from old age and ailments such as Alzheimer’s.8 Currently, there is a growing range of sensor system which can devise automatic activity recognition that possibly rolls out to other great applications that supports healthcare, comfort, and security.9
Other than that behavioural monitoring, location detections, and other vital information that will be essential to assist when the help arrives, thus can optimize the geriatric care services10. Nighttime wandering is one of the symptoms of severe Alzheimer and can be a nightmare for the caretakers. Through a customized ambient support system, Alzheimer patients can benefit as it can help them to stay at home and regain a circadian cycle, Figure 3. 11
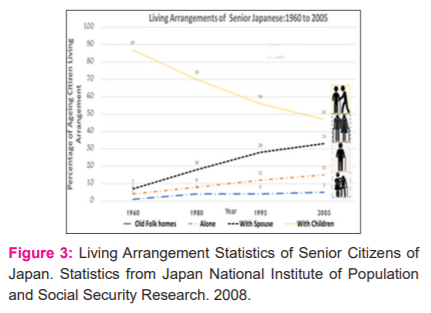
PROBLEM WITH ACTIVITY RECOGNITION SOLUTION
There is not a single solution to solve the problems faced by the AAL actors4. The challenge is to improve activity recognition performance, interpretation and inference. Therefore it raises many questions on the effectiveness of classifiers and sensors, obstruction in the environment, the pervasiveness of gadgets, privacy and security of data, the accuracy of the result, variation in result of similar activity, sensor calibrations, and sensor placements, self-healing of technology, recognition latency and performance of battery life. Most importantly the activity classification for the specific actor in that environment has to be accurate.
Although many research works points out that smart home systems do assist greatly in AIP12 but problems still exist in terms of connecting these well-designed robotic systems to other systems. This is due to the lack of a unified platform and integration of the platforms. To stress the significance of interoperability, emphasized the connectivity, standards and communication protocols plays a vital role in maintaining a home network.13
In a multi-user environment, the gathered data have ambiguities in its interpretation and prediction. In another research, they proposed crowdsourced landmarks to support a multi-user environment.14 In any case, this will require numerous data exchange between server and residents to gather the crowdsourced landmark information, which eventually slows down the system.
Each of the ADLs consists of simple and complex steps. Activity recognition remains a challenge for simultaneous, interleaved, parallel, sequential activities. The pattern of the simple activities leads to the main activities and these are called the composite activities15.
Simple activities are recognized as actions or events.16 These simple activities such as walking and sitting are easy to recognize, activities that have more than one event is difficult to recognize. Thus, more effort is required to recognize these complex activities. Research shows that when there is the presence of multi inhabitant in an intelligent ambient with activity recognition it is deemed to be a detriment as the majority of systems does implicit assumptions based on object detection and their location to the user.17
One study has categorized high-level activities into three that is action (single user), interaction (multiple users) and group activity6.
The technique used in research determines that the smart relation between correlations and constrain which provides a simpler understanding of the recognition of activity in a complex environment.27 Figure 4 shows a compilation of types of the technique used to measure single and multiple users in AR environment taken from various research papers.
To recognize activities effectively there are three stages involved that are activity sensing, activity modelling, and finally the inference of the type of activity. This is also believed to support a multi-user environment.28
As mentioned accuracy improvement depends on multiple sensors and continuous data sensing15, however, this will result in a high number of data to be processed. Many methods such a data fusion, or sensor fusion are taken to classify the activity and also eliminate the redundancy. Deep Learning and Machine learning support in extracting appropriate data to recognize desired activities. Among its steps feature selection and feature extraction algorithms supports extracting relevant data that accurately produce the type of activity. Additionally, temporal reasoning refines the correctness of the algorithms23. Findings show that although there are many methods to do sensor fusion, most of the research does not specify any reasons for their usage.30 The same research also compared heterogeneous sensors to homogenous sensors which showed that the former gives better performance. From that finding, it is suggested that to get better performance in recognizing activities consider research on the optimal sensor combination that may give a better result.
TECHNOLOGY SUPPORT AND SOLUTION
There are four approaches to activity recognition, which is some using wearable devices, video-based device, devices in an ambient environment and Smartphone-based pervasive devices 5 and also robotics. Ubiquitously networked robotics has also become part of the activity recognition approach. It is used in the new applications in which robotics systems will render various assistive services. The contextual information that is collected will be assisting the robot to automatically provide services.21 Computerized approaches focusing on continuous, objective, and quantitative assessment.23 Since the accuracy of the recognition of the activity is a challenge for real-world situations, implementation of temporal reasoning improves the accuracy of their algorithms. To identify (activities in real-time, using online sensor streaming and also a set of different statistical spatiotemporal features through the proposed windowing technique is used.1 Recognizing multi-user is using data location too determines the position of the user indoors by using an indoor positioning module (IP) that is based on Bluetooth technology. Another more efficient method is to analyze the data from inertial and acceleration sensors embedded on the user’s wearable device or smartphone to track his or her movement. Additionally, this can also be achieved in using vision-based techniques for human activity recognition.31,32
These days the smartphone makes it possible for ubiquitous healthcare service for anyone because the smartphones have become a necessity in everyone’s life.12 These smart devices itself gets more sophisticated with sensing capabilities, better algorithm computing capabilities and intensive algorithms on the mobile devices.5
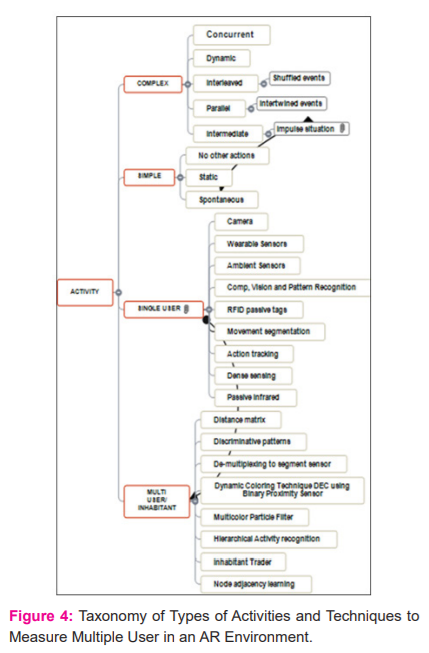
Most smartphones are equipped with motion and location sensors such as accelerometer, light sensors, magnetometer and GPS. They can be integrated with the AAL system that works together with other environmental sensors through collecting information that determines environmental conditions such as CO2 level, temperature and humidity 18 and Smartphones rapid improvement on its memory, a fast processor(s) and the powerful battery provides a new opportunity for HAR researches as no additional devices are needed (Figure 4). The most interesting improvement to smartphones is their capability to do high-level programming with various visualizations. 24
Although the battle in improving the performance and accuracy of recognizing activities in a multi-user environment continues the future work must cater to this large variability of activities. Especially as human started to multi-task even more than before. Therefore it is essential to continuously monitor the living condition through fusing data acquired from various wearable and mobile sensors, video, infrared camera, image or object detections with other technologies such as IoT, Machine Learning, Human Activity Recognition, deep learning and Cognitive IOT. 17, 23
CONCLUSION
Activity recognition safeguards the elderly’s safety in emergencies and identifies changes in their body by continuous unobtrusive monitoring and accurate activity, lifestyle and behavioural profiling stress the importance of monitoring activities of daily living (ADLs) to continuously determine their safety as they age. Activity recognition in a multi-user activity home has to be improved to enhance the living standard of the elderly. This is essentially where AR’s effectiveness is revolutionizing. Through powerful data processing capability from the ambient intelligent environment. AAL comes into the picture because its main aim is to aid the elderly and the disabled to care for themselves independently in their own homes.
ACKNOWLEDGMENTS
The authors also wish to express gratitude to the management of Asia Pacific University of Technology & Innovation (APU) for their support.
CONFLICT OF INTEREST
The authors involved in the current study does not declare any competing conflict of interest.
FUNDING AND SPONSORSHIP
No fund or sponsorship in any form was obtained from any organization for carrying out this research work.
References:
[1] Al Machot F, Mayr HC. Improving human activity recognition by smart windowing and Spatio-temporal feature analysis. InProceedings of the 9th ACM International Conference on PErvasive Technologies Related to Assistive Environments 2016;29: 1-7.
[2] Al-Sharqi R, Mourshed M, Rezgui Y. Progress in ambient assisted systems for independent living by the elderly. Springer Plus. 2016 Dec 1;5(1):624.
[3] Avgerinakis K, Briassouli A, Kompatsiaris I. Activity detection and recognition of daily living events. InHealth Monitoring and Personalized Feedback using Multimedia Data. Springer, Cham. 2015; 15:139-160..
[4] Calvaresi D, Cesarini D, Sernani P, Marinoni M, Dragoni AF, Sturm A. Exploring the ambient assisted living domain: a systematic review. J Amb Intellig Hum Comp. 2017 Apr 1;8(2):239-257.
[5] Cao L, Wang Y, Zhang B, Jin Q, Vasilakos AV. GCHAR: An efficient Group-based Context-Aware human activity recognition on the smartphone. J Para Distr Comp. 2018 Aug 1;118:67-80.
[6] Ismael A, Jayabalan M, Al-Jumeily D. A Study on Human Activity Recognition Using Smartphone. J Adv Res Dyn Cont Syst. 2020; 12(05): 795-803.
[7] Chernbumroong S, Cang S, Yu H. A practical multi-sensor activity recognition system for home-based care. J Adv Res Dyn Cont Syst. 2014;66:61-70.
[8] Cook DJ. How smart is your home. Sci. 2012 ;335(6076):1579-81.
[9] Hasan AR, Nair PL. Urbanisation and growth of metropolitan centres in Malaysia. Malaysian Journal of Economic Studies. Sci. 2017 Jun 2;51(1):87-101.
[10] Fattah SM, Sung NM, Ahn IY, Ryu M, Yun J. Building IoT services for ageing in place using standard-based IoT platforms and heterogeneous IoT products. Sens Prod. 2017;17(10):2311.
[11] Fernandez-Carmona M, Bellotto N. Online inference comparison with Markov logic network engines for activity recognition in AAL environments. In2016 12th International Conference on Intelligent Environments (IE) 2016 ;14:136-143.
[12] Ghose A, Sinha P, Bhaumik C, Sinha A, Agrawal A, Dutta Choudhury A. UbiHeld: ubiquitous healthcare monitoring system for elderly and chronic patients. InProceedings of the 2013 ACM conference on Pervasive and ubiquitous computing adjunct publication 2013; 8: 1255-1264.
[13] Lutze R, Waldhör K. Personal health assistance for elderly people via smartwatch based motion analysis. In2017 IEEE International Conference on Healthcare Informatics (ICHI) 2017;124-133.
[14] Maekawa T, Kishino Y, Sakurai Y, Suyama T. Activity recognition with hand-worn magnetic sensors. Personal and ubiquitous computing. 2013 Aug 1;17(6):1085-94.
[15] Malazi HT, Davari M. Combining emerging patterns with random forest for complex activity recognition in smart homes. Applied Intelligence. 2018 Feb 1;48(2):315-30.
[16] Manca M, Parvin P, Paternò F, Santoro C. Detecting anomalous elderly behaviour in ambient assisted living. InProceedings of the ACM SIGCHI Symposium on Engineering Interactive Computing Systems 2017 Jun 26 (pp. 63-68).
[17] Ni Q, García Hernando AB, Pau de la Cruz I. A context-aware system infrastructure for monitoring activities of daily living in the smart home. Journal of Sensors. 2016;1.
[18] Pereira JD, da Silva e Silva FJ, Coutinho LR, de Tácio Pereira Gomes B, Endler M. A movement activity recognition pervasive system for patient monitoring in ambient assisted living. InProceedings of the 31st Annual ACM Symposium on Applied Computing 2016;4:155-161.
[19] Radziszewski R, Ngankam H, Pigot H, Grégoire V, Lorrain D, Giroux S. An ambient assisted living nighttime wandering system for elderly. InProceedings of the 18th International Conference on Information Integration and Web-based Applications and Services 2016;368-374.
[20] Samuel SS. A review of connectivity challenges in IoT-smart home. In2016 3rd MEC International conference on big data and smart city.Int Comm Bri Disp. Sci Comp. 2016;3:1-4.
[21] Sebbak F, Benhammadi F. Majority-consensus fusion approach for elderly IoT-based healthcare applications. Ann Telecomm. 2017 Apr 1;72(3-4):157-71.
[22] Siegel C, Hochgatterer A, Dorner TE. Contributions of ambient assisted living for health and quality of life in the elderly and care services-a qualitative analysis from the experts’ perspective of care service professionals. Bri Med Geriatrics. 2014;14(1):112.
[23] Singla G, Cook DJ, Schmitter-Edgecombe M. Incorporating temporal reasoning into activity recognition for smart home residents. InProceedings of the AAAI Workshop on spatial and temporal reasoning 2008;53-61.
[24] Suto J, Oniga S, Lung C, Iroha I. Recognition rate difference between real-time and offline human activity recognition. In2017 International Conference on Internet of Things for the Global Community (IoTGC) 2017;10:1-6.
[25] Van Kasteren T, Noulas A, Englebienne G, Kröse B. Accurate activity recognition in a home setting. InProceedings of the 10th international conference on Ubiquitous computing 2008;21:1-9.
[26] Yao L, Sheng QZ, Benatallah B, Dustdar S, Wang X, Shemshadi A, Kanhere SS. WITS: an IoT-endowed computational framework for activity recognition in personalized smart homes. Computing. 2018 Apr 1;100(4):369-385.
[27] Alam MA, Roy N, Misra A. Tracking and Behavior Augmented Activity Recognition for Multiple Inhabitants. IEEE Transactions on Mobile Computing. 2019; 30(8): 452-456.
[28] World Health and Organization. U.S. Department of Health and Human Services. Global Health and Aging, 2011.
[29] Azimi I, Rahmani AM, Liljeberg P, Tenhunen H. Internet of things for remote elderly monitoring: a study from a user-centred perspective. Journal of Ambient Intelligence and Humanized Computing. 2017 Apr 1;8(2):273-289.
[30] Aguileta AA, Brena RF, Mayora O, Molino-Minero-Re E, Trejo LA. Multi-Sensor Fusion for Activity Recognition—A Survey. Sens Prod. 2019 Jan;19(17):3808.
[31] Okeyo G, Chen L, Wang H. Combining ontological and temporal formalisms for composite activity modelling and recognition in smart homes. Fut Gen Comp Syst. 2014;39:29-43.
[32] Jang B, Kim H, Kim JW. IPSCL: An Accurate Indoor Positioning Algorithm Using Sensors and Crowdsourced Landmarks. Sens Prod. 2019 Jan;19(13):2891.
|