IJCRR - 13(10), May, 2021
Pages: 165-169
Date of Publication: 19-May-2021
Print Article
Download XML Download PDF
An Intuitive Framework to Segment the Fetal Brain Abnormalities using Improved Semantic Blend Segmentation Algorithm
Author: N. Suresh Kumar, Amit Kumar Goel, Tapas Kumar
Category: Healthcare
Abstract:Introduction: In this day and age, Machine Learning in clinical imaging introduces an energizing time with reengineered and rethought clinical abilities. Deep Learning aids deep further to make physicians feel like a walk in the park with a handful of more desirable resources. Aim and Objective: The Research focuses to classify and segment the abnormalities in the fetal brain MRI images. The normal and lesion tissue are identified with their location from the given raw images. Method: The model will perform localization, Segmentation, and Enhancement of the Fetal Brain and able to address the two significant abnormalities such as Encephalocele and Arteriovenous Malformation using the Improved Semantic Blend Segmentation Algorithm. Results: The model has been trained with the capability to segment the Region of Interest (ROI) on an average of 7.2 Seconds per input. Conclusion: The raw fetal brain images are segmented and enhanced with various classes of input and the results are analyzed which outperforms the existing techniques by saving time and achieving better accuracy.
Keywords: Fetal Brain Segmentation, Improved SBS Algorithm, Semantic Segmentation, U-Net Architecture
Full Text:
INTRODUCTION
The radiologists deal with expanding and the outstanding task at hand with more information from patients concerning electronic health care records. A definitive task is to play out the manual segmentation of the variations from the norm in the pictures from the information gathered will be more time-consuming. The qualities of the computational framework help the pathologist in a critical decrease by large mistake rate. Automatic segmentation is the best solution to address this issue with the help of evolving technology. The Machine Learning (ML) has been very staggering over the past few which conquered all the place where time consumption need be reduced and accuracy need to be increased. ML will empower clinicians to work better more precisely which will downstream lead to better outcomes for patient care.1 ML has the potential to be an excellent contributor to the entire image interpretation lifecycle beginning from image acquisition to the interpretation and communication of those results. A push is recognized in building new techniques and tools to all the more viably analyze the illness and fix the ailment can majorly affect a huge extent of the population. ML protection medical services applications can be utilized to distinguish different conditions, or any lesion which is driving vital innovation in the health care industry in this decade. On leveraging ML and advanced IT tools in the medical field will achieve data-driven or evidence-driven machines for a human optimized workflow.
Machine Learning plays an eminent role in assisting the medical field with improved early prediction of diseases and abnormalities. Medical imaging segmentation is a significant process in diagnosing abnormalities.1 When the human manually analyzes the images one by one for the abnormal lesion it will be more tedious, exceptionally mind-boggling, and inclined to high mistake rates delays in conclusion. Perhaps, the treatment can be dangerous and can have basic ramifications for a patient's wellbeing. Automatic Segmentation is a precise way to find abnormalities using Machine Learning Algorithms.
The woman of 3 out of 1000 patients have fetal abnormalities.2 The main aim is to locate, segment, and enhance the Fetal Brain Abnormalities from the Fetal MRI images, which is taken as a Region of Interest (ROI) in this work.3,4 With the advancement of profound learning, various deep learning strategies have been proposed to improve MRI image enhancement and highlighting abnormalities.5
Magnetic Resonance Imaging in short it is termed MRI, which is the peculiar modality that provides a beneficial interpretation of the fetal brain scanned images.
The MRI images are taken as the input to our model input. The image enters the room in the .nii format which is the direct output of the scanned machine. The .nii images are loaded in the model and processed into machine learning algorithms. The inference generated will assist the physician to ensure the lesion shape, size, intensity, and significantly the abnormalities of the fetal brain. This model forestalls human-based indicative mistakes and simplifies exertion during an assessment.1,6
K.Somasundaram et al.stated to localize the fetal brain using the centre of gravity (COG) technique, which is a time-consuming but efficient way to achieve the best results.6 He has achieved with less computational methods which are highly handy. The total time taken to perform the localization of the fetal brain takes less than one minute after depicting the algorithm with the input. The area-based segmentation technique was adopted by Omneya Attallah et al., results applied all the three planes of sagittal, coronal, and axial of brain images. The obtained segmented fetal brain images are classified into two categories, a) Healthy class and b) Unhealthy class. The classification accuracy was 95.6%.7
The Superpixel graphs technique was adopted to obtain fetal brain segmentation by Amir Alansary et al., which extracts the features from the segmented pixels and produces a Superpixel graph. Using the superpixel graph, the classifier will categorize whether the given fetal brain is brain or non-brain and localize the fetal brain from the given input.5,8
MATERIALS AND METHODS
Data Collection
The Fetal MRI images of 117 Dicom volumes are obtained from the Beth Israel Deaconess Medical Center, Boston associated with Harvard Medical School. The images were collected with Philips (1.5 Tesla) of T-2 weighted images.
Architecture
The deep convolutional network for the segmentation of biomedical images significantly uses U-Net Architecture. The U-Net is specifically configured for the Biomedical Images.4 In Figure 2.2.1 The U-Net architecture will resemble all other convolutional networks it comprises of countless various tasks showed by these small arrows, the input image is feed into the network in the initial phase then the data is engendered through every conceivable way till the end, the segmentation map will be received at the end of the process. Each blue box corresponds to a multi-channel feature and the number of featured channels with the scale of x and y size.
The majority of the tasks are convolutions trailed by a nonlinear activation function. It is a standard 3x3 convolution followed by a nonlinear activation function an important design choice is that it uses the valid part of the convolution which means that for a 3 by 3 convolution a 1-pixel border is lost this allows later to process large images in individual tiles. The following activity in the U-Net is max-pooling it diminishes the XY size of the element map which was represented as a downward arrow.
The max-pooling follows up on each channel independently it just engenders the maximum activation from each 2x2 window to the following feature map.
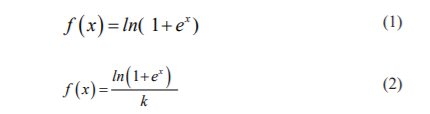
After every max-pooling operation, it expands the number of featured channels by a factor of 2 all things considered the arrangement of convolutions and max-pooling operations brings about a spatial contraction where it gradually increases the bot and at the same time decreased the wear. The standard classification ends with this step. Presently, for all features to change a single yield vector the unit has an extra extension way to make a high-resolution segmentation map. This expansion path comprises an arrangement of up convolutions and connection with the relating high-resolution features from the contracting path. This convolution utilizes a learned kernel to map every feature vector to the 2 x 2-pixel output window, trailed by a nonlinear activation function. The output segmentation map contains two distinct channels, foreground and background class. Due to the haphazard convolutions, the map is smaller than the input image. The Softmax Output layer is given by,

Where S denotes the input vector.
Procedure
The fetal brain MRI image is taken as input to the system, where the images will be in the .nii or .nii.gz format of the T2 imaging standard. In this Research, The 94 volume of Healthy and 23 Unhealthy fetal brains was used. The Proposed steps are illustrated in figure 3.1 to perform the Improved Semantic Blend Segmentation (SBS) Algorithm followed by the Optimal Slice Enhancement (OSE) Algorithm.
i) The pre-processing step includes the dataset preparation for the localization and segmentation. Initially, the images are converted into .nii format into .jpg format using the python library named med2image. This library will take all the .nii files from the source folder as input and converts them into the JPG files and store them in the destination folder.
Each slice from the input volume is converted into a jpg image additionally comprises the Sagittal, axial and coronal views are divided separately. The next step is to analyze whether the images all the images in the folder are of the same size. This step is performed using a python library, PIL. This module will take all the images from the Source folder and convert into the same size of 256 x 256 pixel.
Now, the images are split into two sets of folders, called train and testing. The train folder carries 80% of the images and the rest of the images were placed in the test folder. The U-Net model generated image label will be in the .xml file which is to be converted into .csv for further process. The generated .csv file will contain detailed data regarding file_name, width, and height. In addition to that, .csv will have a labelling column, xmin, ymin, xmax, and ymax with its associated class.
ii) In the Processing Step, The Tensor Flow record (TF Record) file needs to be generated, using the classes declared. In this Research, the classes will be Fetal Brain (Region of Interest) and Unhealthy Fetal brain with various abnormalities. The TF Record will be generated using the .csv files with the associated training images and the same will be performed for the testing images with test.csv in parallel the label map (LM) file will be created. The U-Net architecture will be used to localize the ROI.
The model is configured with the training and testing record directory and set ready for training. The MRI scans of the fetal brain images are divided into two different categories, First Folder contains the Healthy Fetal MRI images (Normal) and Unhealthy Fetal images (Abnormal) which is used for the training phase. The training will be started to learn the ROI image features and the model is trained with NVIDIA GPU 1050. The fetal brain images are trained using obtained image features using Machine Learning. The training will be started to learn the ROI image features and the model is trained with NVIDIA GPU 1050. The fetal brain images are trained using obtained image features using Machine Learning.
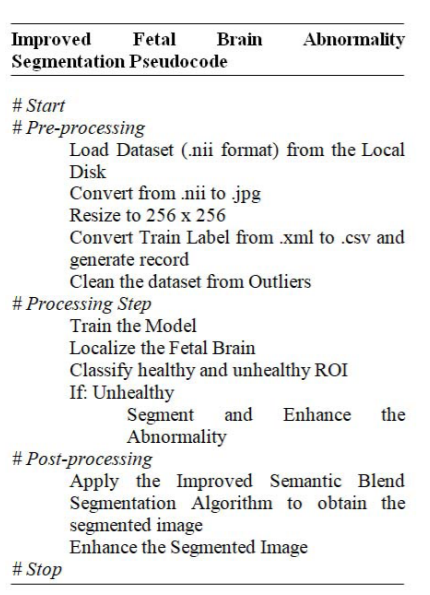
The trained model is used for predicting the abnormalities from the given input. The test image is passed to the model to detect whether the given image is healthy or unhealthy. If Unhealthy, which type of abnormalities are identified. The model was trained with 3 different classes. Class 1, depicts the healthy fetal brain, Class 2 and 3 which address the abnormality of Encephalocele and Arteriovenous Malformation respectively. In all these classes, the model will spotlight the physician with, what type of abnormality is found, and what is the impact o Post-Processing Step, the segmentation of the fetal brain is initiated by feeding the input image to the Improved Semantic Blend Segmentation Algorithm. The algorithm will blend the processed image into the designated LM file to obtain the segmented image as the output.3 The resultant output will be enhanced.
RESULTS AND DISCUSSION
The raw input image is taken as the input to the algorithm, which processes the segmented enhanced image as the output. Figure 1 illustrates, the complete incremental process of the system output. In Figure 1(a), a slice of the MRI fetal brain is chosen using the algorithm with the best view for analysis, which is initially in the format .nii. Figure 1(b), the label of the fetal brain is extracted from the input image.
The Figure 1(c-f), the enhanced image of the fetal brain is processed to make better visualization analysis for the physician to detect the abnormalities. Figure 1(g,h), leads to the segmentation of the fetal brain and its enhancement representation.
The interpretative representation of the Class 1 category of the fetal brain which is normally free from abnormalities is exhibited in Figure 1. Class 2 and 3 of Encephalocele and Arteriovenous Malformation respectively are processed in Figure 2, which provides the broad resource and perception for the physicians to analyze the patient’s disease progress and take the decision, incrementally better than the previous methods.
All the images were generated automatically after getting the input from the local disk, which is ultimately faster than the manual handling of the MRI images. The significant aspect of the system is to reduce the time of segmenting the fetal MRI images.
A batch of 17 MRI cases are passed to the model in Table 1 the abnormalities are localized and segmented in 318 Seconds. The model process on an average of 18.74 Sec/per case, which is highly efficient when compared to all existing models.
The bar chart and graph in Figure 4 and 5 respectively interprets the individual case time taken and compared with the other volume passed in the batch. The total time taken to complete the total batch of 17 MRI volume of images is 5 minutes and 18 seconds.
CONCLUSION
The classification, localization, and segmentation are performed by this model. The classification informs whether the given input is the normal or abnormal fetal brain. This is the first milestone that delivers significant data about the given input. The localization is performed on the next step to depict the ROI position, with its meticulous shape and size. If the brain is abnormal the lesion tissue is emphasized in the phase. In the final phase, Segmentation of the fetal brain is performed by using the Improved Semantic Blend Segmentation Algorithm, which provides the fetal brain and its abnormalities. This model was a tower of strength to physicians, who work with fetal brain segmentation.
Acknowledgement: Authors acknowledgement the enormous assistance received from the scholars whose articles are cited and included in references of this manuscript. The author is thankful to authors/editors/publishers of all those articles, journals and books from where the literature for this article has been reviewed and discussed.
Conflict of Interest: Nil
Source of Funding: Nil
Authors' Contribution: The first author involved in conceptualization, conducted the study, collected data, perform implementation, wrote manuscript, reviewed. The second and third author guided the research work in making necessary amendments in the write-up and final review.
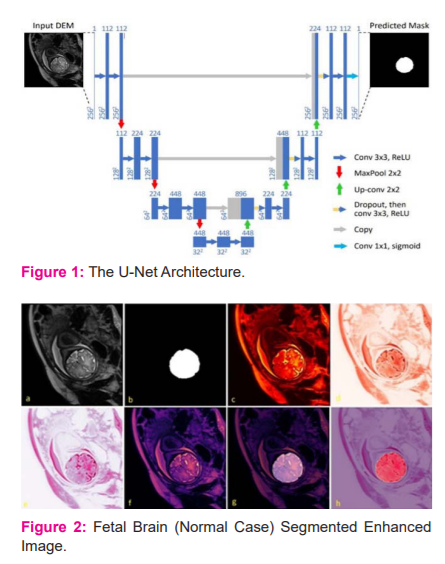
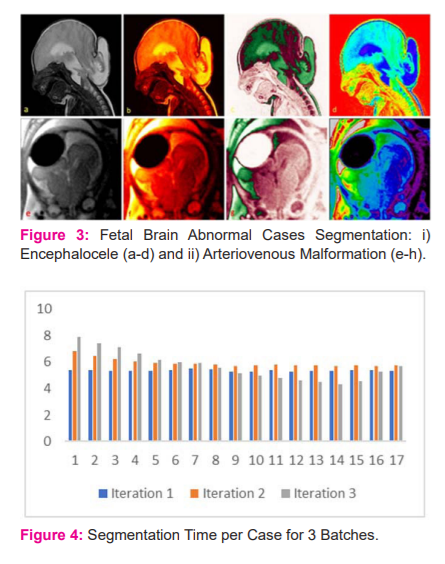
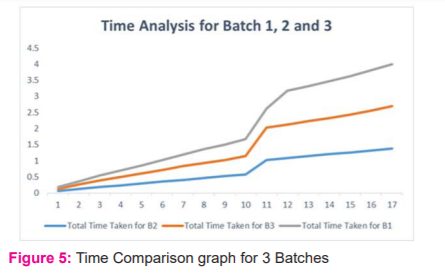
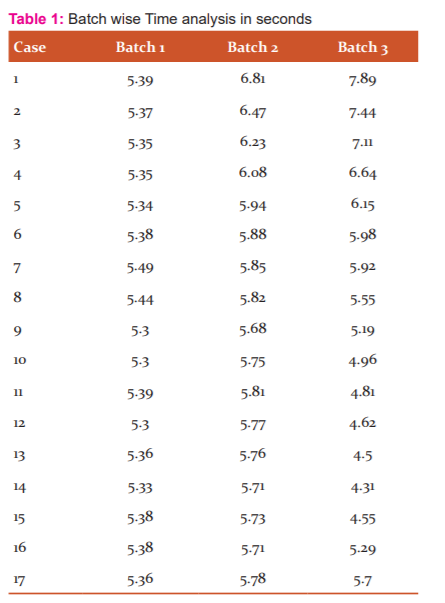
References:
-
Wiljer D. Developing an Artificial Intelligence–Enabled Health Care Practice: Rewiring Health Care Professions for Better Care. J Med Imaging Radia Sci 2019;50(4):8-14.
-
Sharma KN, Kamra A. A Model for Mammogram Image Segmentation based on Hybrid Enhancement. Int J Curr Res Rev 2020;12(16): 34-39.
-
Bhide P, Gund P, Kar A. Prevalence of Congenital Anomalies in an Indian Maternal Cohort: Healthcare, Prevention, and Surveillance Implications. PLoS One. 2016;11(11):1-13.
-
Vijayalakshmi, Kumar NS. A Review on Fetal Brain Structure Extraction Techniques from Human MRI Images. Int J Comp Sci Engi 2018; 6(4): 239-24.
-
Alansary A, Lee M, Keraudren K, Kainz B, Malamateniou C, Rutherford M, et al., Automatic Brain Localization in Fetal MRI Using Superpixel Graphs. In Revised Selected Papers of the First International Workshop on Machine Learning Meets Medical Imaging (LNCS). 2015; 9487(1):13–22.
-
Somasundaram K, Gayathri SP, Shankar RS, and Rajeswaran R. Fetal head localization and fetal brain segmentation from MRI using the center of gravity. International Computer Science and Engineering Conference (ICSEC), Chiang Mai. 2016; 1-6.
-
Attallah O, Sharkas, Maha. A Gadelkarim H. Fetal Brain Abnormality Classification from MRI Images of Different Gestational Age. Brain Sci 2019;9(9): 231-252.
-
Tourbier S, Automated template-based brain localization and extraction for fetal brain MRI reconstruction. Neuroimage 2017; 155(1):460-472.
|