IJCRR - 13(10), May, 2021
Pages: 101-106
Date of Publication: 19-May-2021
Print Article
Download XML Download PDF
Abnormality Detection from X-Ray Bone Images using DenseNet Convolutional Neural Network
Author: Shukla Abhilash, Patel Atul
Category: Healthcare
Abstract:Introduction: According to the survey of the World Health Organization and the International Agency for Research on Cancer; the death rate because of cancer is increasing day by day. It is preferable to detect cancer at its earlier stage or detect any kind of lesion which can cause cancer in the future. This paper shows how Artificial Intelligence especially the Convolutional Neural Network of Deep Learning can be used to detect abnormality from X-Ray bone images. Objective: To detect the abnormality in bone from X-Ray Images. Methods: MURA (Musculoskeletal Radiographs) dataset is used which was prepared by the Stanford ML group. Dataset is identified and categorized into training and validation dataset and after that data preprocessing techniques are used. This help in making the dataset convenient for the DenseNet (Densely Connected Convolutional Networks) Model. Tenserflow and Keras libraries are used to build DenseNet model. Results: Classification Table and Confusion Matrix methods are used to evaluate the performance of DenseNet (Densely Connected Convolutional Networks) Model for the detection of abnormality in bone from X-Ray Images. By using this proposed model more than 85% accuracy achieved. Conclusion: The result obtains from the proposed model will be helpful to the radiologist to make better decisions. This independent model can further be used to detect cancer of bone from X-Ray Images
Keywords: Bone Abnormality, Bone, Convolutional Neural Network, Deep Learning, DenseNet, X-Ray Images
Full Text:
Introduction
Deep Learning had a remarkable impact on the various field like agriculture, medical, education, weather, entertainment and many more in recent years. Deep Learning is the extension of machine learning methods. These methods are based on artificial neural network.1
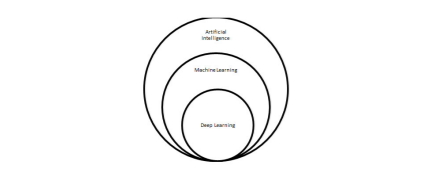
Figure 1: Figure shows that Deep Learning is the subset of Machine Learning, which come under the umbrella of the Artificial Intelligence domain2
The main benefit of deep learning over machine learning is that deep learning automatically detects features, which may be 1000 times more than what human can think of. Deep Learning techniques used to train computers to learn through a huge amount of data. A computer program can be developed which can gain knowledge and analyze any data like a human does, through deep learning methods. Research in this field has broad-spectrum in the applications like Chatbots to improve Customer experience, Spelling and grammar correction for errorless text writing, detect objects from the satellite which help to aerospace and military, auto-generation of music as per human mood, service which senses the unusual things in industrial plant, cancer cell detection in medical research, self-driving car and many others. In many of the fields, we have to deal with images rather than data or information. In computer vision, this process is well known as image processing.3 This image processing can be done more effectively and efficiently with the help of Convolution Neural Network, which is modelled, based on the concept of deep learning. Convolution Neural Network has three layers called input, hidden and output. Various operations like convolution, activation, padding, pooling, normalization and Softmax performed to obtain the desired output in the hidden layer of CNN.CNN become a newly emerging field in deep learning through which image can be read and analyze to produce a significant result.4
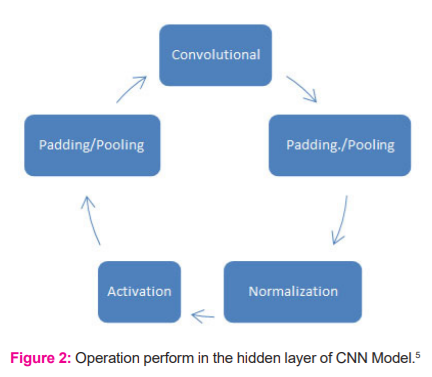
Cancer, which considers as an abnormal growth of the cell, which harasses and spread into any human body organ. As per the record of ICMR-NICPR (Indian Council of Medical Research – National Institute of Cancer Prevention and Research), More than 2.5 million people live with this disease in India. On average 7 lakhs of new patients with cancer registered and around 6 lakhs of people lose their life because of cancer. According to World Health Organization (WHO), Worldwide 9.6 million people were estimated to have died from cancer in 2018.6 As per the survey of the International Agency for Research on Cancer (IARC), there will be 21.7 million cases and 13 million deaths in 2030 predicted because of cancer. In India, over 80% of cancer cases are detected late and patiently advised to go for advanced-stage treatment.7
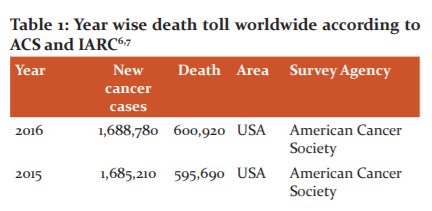
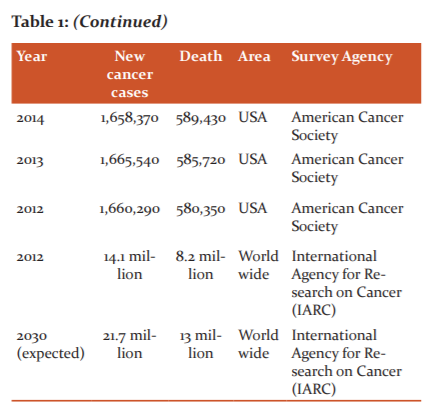
This death rate can be reduced if cancer detected in its early stage or by finding any abnormality and rectify it before it converted into a tumour. A total of 75 types of cancer exists from which one type is bone cancer. In bone cancer, also there are 19 types of bone cancer. Osteosarcoma and Ewing are the most commonly seen bone cancer. The research objective is to detect any kind of abnormality or lesion on the bone from x-Ray images at its early stage so that patient gets the treatment at the earliest and can be saving from cancer.
The number and the table suggest the diversity and adversity in the disease like cancer. So, at the preliminary stage of the research, it has been found some thrust in the research of such disease prevention and prediction. Medical science is completely dependent upon the various images, which is generated or prepared because of some report testing. It is diagnosed only after the body is completely affected by that particular disease. The changes in the body when the body host the decease initially, the decease can be prevented or cured if the reports are tested and examined in early stage.
With this initial perception and state of mind, the research title was proposed where such decease prevention can be done in a much more efficient and effective manner where the chance of decease can be predicted in advance. The corrective steps can be taken to avoid it too completely. Moreover, Artificial Intelligence with Computer Vision and Image Processing was found most suitable to research the domain.3
Literature Survey
Substantial and remarkable work in the field found which is targeting disease detection and prevention with aids of Information Technology. Algorithm to calculate the mean intensity and tumour size from MRI images found useful in the prediction of various stages of cancers and bone cancer.8 It has been found that Region Growing Algorithm is the best-suited method to detect tumour size and bone cancer stage.9 Various other methods like texture-based region growing, cellular automata edge detection, K-Mean clustering algorithm and algorithm to calculate the sum of pixel intensities also gives the better result to detect cancer. Image segmentation is one of the important processes in image processing to detect bone cancer from radiography image.10-17 Especially biomedical image segmentation based on Entropy, Fuzzy Entropy and the Least Square Method used for it.18 Neuro-Fuzzy Classifier found useful to detect different types of brain cancer. In this various image processing techniques are used and the grey level Co-occurrence Matrix method used to extract texture features.19
The Computer Vision and Image Processing Feature Extraction and Pattern Classification (CVIP-FEPC) software found useful to detect bone cancer from thermography images. Thermography image gives better result than X-Ray, CT scans and MRI images in terms of diagnostic time and reduces the exposure of radiation.20 There are some advantage and disadvantage of using computer-aided diagnosis and it was found that it gives more accurate result.21 Osteosarcoma and Ewing’s sarcoma having similar early symptoms like fever and pain.22 Radiologist also remain in dilemma in the detection of these two types of bone cancer and this type of case, computer vision technology with artificial intelligence will be useful to the radiologist to draw a decision. Looking at the era and the current trends with futuristic technologies, the tasks related to image processing can be dominated by the use of Artificial intelligence especially Convolutional Neural Network techniques of Deep Learning.23-29
Research Rationale and Methodologies
Dataset
Dataset is one of the most crucial and important components for any deep learning algorithm. This dataset is divide into three categories called training dataset, validation and test dataset. The training dataset is the backbone for any deep learning project. The training dataset is used to train the neural network and it comprehends and memorizes such data, which help the network to predict the information in future. More amount of data in the training dataset result in accurate prediction. After having a dataset, the next important task is to label the data properly so that the neural network can be trained to answer Yes/No or can able to categories the data. Validation data set are used to identify the overfitting and under the fitting issue of neural network.
To trained the proposed CNN model, MURA (Musculoskeletal Radiographs) dataset is used which was prepared by the Stanford ML group and it also opens access. MURA is one of the largest datasets of bone X-Ray images. MURA contains around 60,000 images from 14,656 studies. All images are labelled as either normal or abnormal by the radiologist. To obtain a better result these images are classified into seven different classes Elbow, Finger, Forearm, hand, homers, shoulder and wrist.
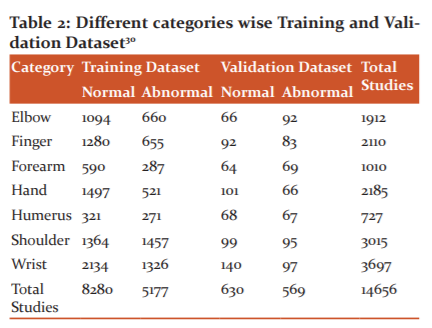
Data Preprocessing and Augmentation
Data preprocessing is the important step by which data can be frame into proper input for the proposed model. In data preprocessing, we did the following three things. Data augmentation is the method in deep learning to increase the training dataset so that it results proposed model to provide an accurate result. Normally, Data Augmentation can be done by rotating image and flipping the images horizontally and/or vertically. For the research, we had to increase the dataset for training and validation. To achieve this, we had rotated the images to 30 degrees and flipped them horizontally. Because of this, we had 2 times more dataset than we started initially.
Image Normalization
Normalization of images is required so that the proposed neural network don’t face the issue of over or underfitting. To normalize all images, the mean and standard deviation are calculated. This mean value subtracted from each image mean intensity value and then subtracts the result value by standard deviation.
Resizing Images
All CNN model required a fixed size of images as input. The model, which we proposed, required all image in 224 x224 sizes.
Proposed Model
DenseNet Model used to detect any kind of lesion from bone X-Ray images. DenseNet is architecture is based on ResNet Architecture. The major difference between ResNet and DenseNet is that in ResNet each layer received a piece of knowledge from its immediate previous layer while in DenseNet each layer received collective knowledge from all its previous layer. Batch normalization, ReLU and 1x1 Convolution operations to reduce the model complexity and size. This layer considers a bottleneck layer in DenseNet.
Advantage of DenseNet
• Better Gradient Flow
• Efficiency gained in parameter and computation
• Diversified feature identified instead of co-related feature
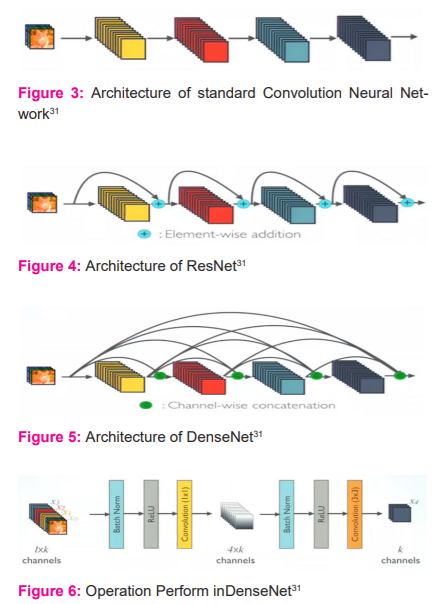
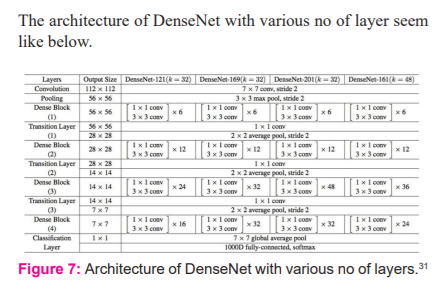
With the help of TensorFlow and Keras library, DenseNet with 169 layer and Sigmoid activation function can build such that in each epoch model loss is decrease and accuracy increase.32
RESULTS
Confusion Matrix and classification table methods are used to evaluate the model for lesion detection of X-Ray bone images.
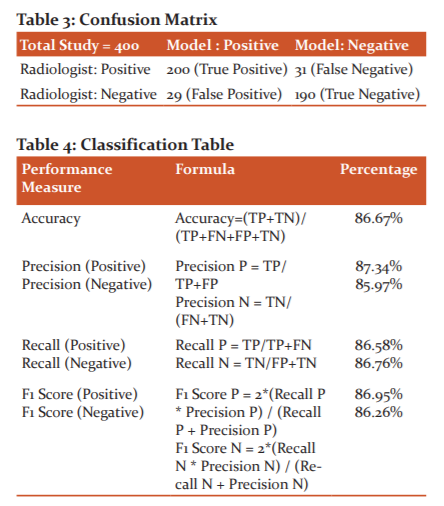
Conclusions
From the result, it can be concluding that the DenseNet Model can be used to detect abnormality from Bone X-Ray images. The same model can be used for cancer detection which can be detected from x-Ray images. These results will be helpful to the radiologist for the prediction of abnormality and presence of cancer in the X-Ray and MRI images.
Acknowledgement: The authors acknowledge the support received from the researcher whose articles are cited and included in references to this manuscript. The authors are also grateful to authors/ editors/publishers of all those articles, journals and books from where the literature for this article has been reviewed and discussed.
Conflict of Interest: NIL
Source of Funding: NIL
Authors’ contribution
Mr. Abhilash Shukla: Study Design, Literature Review, Dataset, Implementation
Dr. Atul Patel:: Data and Statistical analysis, Manuscript Review
References:
1. Yann L, Bengio Y, Hinton G. Deep learning. Nature 2015;521(7553):436-444.
2. Bini SA. Artificial Intelligence, Machine Learning, Deep Learning, and Cognitive Computing: What Do These Terms Mean and How Will They Impact Health Care? J Arthropl 2018;33(8):2358-2361.
3. Wiley V, Lucas T. Computer Vision and Image Processing: A Paper Review. Int J Artif Intellig Res 2018;2(1):28-36.
4. Jia X. Image recognition method based on deep learning. 29th Chinese Control and Decision Conference (CCDC), Chongqing, 2017.
5. Indoliaa S, Goswami A, Mishra SP, Asopa P. Conceptual Understanding of Convolutional Neural Network- A Deep Learning Approach. International Conference on Computational Intelligence and Data Science (ICCIDS 2018), Gurugram, 2018.
6. World Health Organization, 12 September 2018. Available: https://www.who.int/news-room/fact-sheets/detail/cancer. [Accessed 1 June 2020].
7. The International Agency for Research on Cancer (IARC), "IARC, WHO," International Agency for Research on Cancer (IARC), 2019. [Online]. Available: https://www.iarc.fr/featured-news/. [Accessed October 2020].
8. Reddy KC, Anisha PR, Narasimha Prasad L V. A novel approach for detecting bone cancer and its stage based on mean intensity and tumour size. Rec Res Appl Comp Sci 2015;162-171.
9. Reddy KC, Anisha PR, Raju GVS. A Novel Approach for Detecting the Tumor Size and Bone Cancer Stage Using Region Growing Algorithm. 2015 International Conference on Computational Intelligence and Communication Networks, 2015.
10. Senthilkumaran N, Reghunadhan R. Edge Detection Techniques for Image Segmentation - A Survey of Soft Computing Approaches. Int J Rec Trends in Engi 2009;1(2):250-254.
11. Hakeem AA, Tirumala R, Ahsan I. A New Approach to Image Segmentation for Brain Tumor detection using Pillar K-means Algorithm. Int J Adv Res Comp Commun Engi 2013;2(3):1429-1436.
12. Abd-Ellah K, Awad AI, Khala AA, Hamed HFA. A review on brain tumour diagnosis from MRI images: Practical implications, key achievements, and lessons learned. Magn Reson Imaging 2019;61:300-318.
13. Zaitoun NM, Aqel MJ. Survey on Image Segmentation Techniques. Procedia Comp Sci 2015;65;797-806.
14. Kaur A, Kumar R, Kainth K. Review Paper on Image Segmentation Techniques. Int J Adv Res Comp Sci Softw Engi 2016;6(7):336-339.
15. Kumari R, Sharma N. A Study on the Different Image Segmentation Technique. Int J Engi Innov Technol 2014;4(1).
16. Jain SN, Patil BG. Cancer Cells Detection Using Digital Image Processing Methods. Int J Latest Res Sci Technol 2014;3(4):45-49.
17. Yasrib A, Suhaimi MA. Image processing in medical applications. J Inform Technol Impact 2003;3(2):63-68.
18. Vitulano S, Ruberto CD, Nappi M. Different methods to segment biomedical images. Pattern Recog Lett 1997;18(11):11-13.
19. Joshi DM, Rana NK, Misra VM. Classification of Brain Cancer using Artificial Neural Network. 2nd International Conference on Electronic Computer Technology. 2010.
20. Amini M, Liu P, Umbaugh SE, Marino DJ, Loughin CA. Thermographic image analysis method in detection of canine bone cancer (osteosarcoma). 5th International Congress on Image and Signal Processing, Chongqing, 2012.
21. Muhammed Anshad PY, Kumar SS. Recent methods for the detection of the tumour using computer-aided diagnosis — A review. International Conference on Control, Instrumentation, Communication and Computational Technologies (ICCICCT), Kanyakumari, 2014.
22. Kansal R, Gupta P, Arora M, Mattoo P, Khurana A, Bhasin I. Osteosarcoma or Ewing’s sarcoma? Radiologist’s Dilemma. Schol J Appl Med Sci 2014;2(5):1817-1820.
23. Igor S, Aleksej A. Convolutional Neural Network Based Automatic Object Detection on Aerial Images. IEEE Geoscience and Remote Sensing Letters 2016:1-5.
24. Krishnammal PM, Selvakumar R. Convolutional Neural Network-based Image Classification and Detection of Abnormalities in MRI Brain Images. International Conference on Communication and Signal Processing, 2019.
25. Yadav DP, Rathor SS. Bone Fracture Detection and Classification using Deep Learning Approach. International Conference on Power Electronics & IoT Applications in Renewable Energy and its Control. 2020.
26. Wang C, Huang D, Jionglong Su, Limin Yu FM. Generalization and Visual Comprehension of CNN Models on Chromosome Images. IOP Conf. Series: Journal of Physics. 2020;1487:012027.
27. Fatih D, Daban A, Sengur A. A New Deep CNN model for Environmental Sound Classification. IEEE 2020;8:66529-66537.
28. Niu L, Song YQ, Su J, Zhang HM. A deep learning study of extracting navigation area from CAD blueprints. Ann Photogrammetry Remote Sens Spat Inform Sci 2018;4:155-162.
29. Yoder JA. Determining Optimum Drop-out Rate for Neural Networks. The Bridge, The Magazine of IEEE-Eta Kappa Nu. 2018;115(2):10-16.
30. Rajpurkar P, Irvin J, Bagul A, Ding D, Duan T, Mehta H, et al. MURA: Large Dataset for Abnormality Detection in Musculoskeletal Radiographs. 1st Conference on Medical Imaging with Deep Learning. 2018.
31. Huang G, Liu Z, Maaten L, Weinberger KQ. Densely Connected Convolutional Networks. Conference on Computer Vision and Pattern Recognition, 2017.
32. Bharali P, Bhuyan C, Boruah A. Plant Disease Detection by Leaf Image Classification Using Convolutional Neural Network. Springer. Singapore. 2019.
33. Krizhevsky A, Sutskever I, Hinton GE. Imagenet classification with deep convolutional neural networks. Advances in Neural Information Processing Systems. 2012.
34. Yann L. Gradient-Based Learning Applied to Document. Proc IEEE 1998.
35. Kim D H, MacKinnon T. Artificial intelligence in fracture detection: transfer learning from deep convolutional neural networks. Clin Radiol 2018;5:439-445.
36. Lei X, Pan H, Huang X. A dilated CNN model for image classification. IEEE 2019;7:124084-1240-5.
37. Manikonda S, Gaonkar D. IDM based on image classification with CNN. J Engi 2019;10:7256-7262.
38. Priya N, Radhika C. A Survey on Predicting Autism Spectrum Disorder using Machine Learning techniques. Int J Emerg Technol 2019;10(3):422-427.
39. Siegel RL, Miller KD, Jemal A. Cancer statistics. Am Canc Soc J 2020;70(1):7-30.
40. Thaker N, Shukla A. Python as Multi-Paradigm Programming Language. Int J Comp Appl 2019;177(31):38-42.
41. Tripathii AM, Upadhyay A, Rajput AS, Kumar B. Automatic detection of fracture in femur bones using image processing. International Conference on Innovations in Information Embedded and Communication Systems, 2017.
|