IJCRR - 13(2), January, 2021
Pages: 148-153
Date of Publication: 16-Jan-2021
Print Article
Download XML Download PDF
Automated Brain Tumour Detection using Deep Learning via Convolution Neural Networks (CNN)
Author: Sanjay Kumar, Naresh Kumar, Rishabh, Inderpreet Kaur, Vivek Keshari
Category: Healthcare
Abstract:Introduction: Brain tumours are the most known and aggressive disorder, leading to a poor lifetime at the highest level. Treatment is one of the main benefits of development that saves a life. Imagery is used to analyse the tumour in brain, lung, liver, bosom, neck, etc, through tomography, appealing reverb imagery (MRI) and ultrasound imaging. And that's it. Objective: In this study, in particular, the tumour of the mind is examined through enticing reverse imagery. The enormous amount of knowledge produced by the MRI scanner, however, at any one time obstructs the manual tumour against non-tumour order. Result: The process has had several challenges, as computations for several images are reliable. An unambiguous necessity is to increase the survival rate of the programmed order. The scheduling of the mind tumour is an incredibly problematic task in the exceptional spatial and basic fluctuation that accompanies the local brain tumour. Conclusion: In this research, a programmed exploration of mind tumours is proposed using the characterization of convolution neural networks (CNNs). The most important type of composition is the completion of the use of small pits. CNN's paper has less predictability and 97.5 accuracies.
Keywords: Magnetic Resonance Imaging, Convolution Neural networks, Deep learning, Brain Tumour, Tomography, Brain cancer
Full Text:
INTRODUCTION
The Brain tumour in the human body is one of the essential organs; the irregular collection of cells consists of uncontrolled cell division, which is also called a tumour. Tumours of the cerebrum fall in two. Such second rate types (rating 1 with rating 2) as well as elevated evaluation tumours (rating 3 and grade 4). Bad quality mind Favorable is said to be the tumour. Besides, the high calibre. The Malian tumour involves cells of malignancy and affects the tissues involved. The benign tumour is non-malignant, and those parts of the mind do not spread to others. Otherwise, a malignant tumour is called a lymph tumour. It brings swift passage. 12 For the most part, brain-attractive reverberation imaging is worn to identify. The tumour plus the tumour progression model procedure. Attractive reverberation imaging offers increasingly clinical image data since the CT or ultrasound image. Attractive reverberation imaging gives in mind .1-3
Tissue subtlety data on the structure and deviation from the norm of the position of the cerebrum. Scholastics have proposed different strategies for discovering mind tumours, compiling inventories with MRI images when digitizing and shipping is conceivable Clinical photos of a PC. Neural networks (CNN) and bolster vector machines (SVM) are the strategies regularly used for your grand proclamation in the late years.4 However, as of late, profound learning models (DL) rectification of a moving trend in machine learning underground architecture can effectively stand for complex relations with no the need for a great number of nodes as in surface architectures. Ex: adjacent Vector machine K-Nearest (KNN) and bolster (SVM). They grew up fast along these lines to become the best in class, not at all like that. Informatics well-being areas, such as clinical imaging, clinical computing and bioinformatics.5
Brain cancer has arisen when the cell is in-between and growing unusually. This tends to be a rough accumulation when diagnosing an observational imaging technique. Two types of brain cancer are the primary brain tumour and metastatic brain cancer. Main brain cancer is the condition while the tumour is formed in the brain and tends to remain present while the brain is metastatic.
The reason for select CT images upon MRI images be as follows:
1. CT is much easier than MRI, providing an opportunity to learn in shock luggage and other acute neurological emergencies. CT can be made available at a significantly lower price than MRI.
2. The CT container shall be obtained at a significantly lower price than the MRI.
3. CT is less responsive to continuous movement throughout the test.
4. Imaging can be performed much more easily, and CT can be safer to do in claustrophobic or extremely serious cases.
5. CT can be done at no risk to the stamina with implantable control plans, such as a cardiac pacemaker, ferromagnetic vascular clips and intestinal stimulators.
Past research Work
The FCM segmentation of tumours and non-tumour districts shall be used. Besides, wavelets are isolated using a variety of discrete Wavelet Shift (DWT) phases. In the long run, deep neural red (DNN) is incorporated into the tumour order with incredible precision. This approach is in contrast to KNN, simple discriminate (LDA) and marginal successive streamlining (SMO) ordering techniques. Precision levels of 94.96 per cent in the DNN cerebral cancer base classification, however, the unpredictability is extremely high and the sensitivity is exceedingly poor.3 Biophysiomechanical tumour the development model is used to break down the growth of patients' tumour bit by bit. Will be used for gliomas and large tumours with incentives to achieve enormous tumour mass tests. Discreet and persistent techniques are combined for the creation of a tumour model. The proposed conspiracies offer the possibility of potentially imaging a tumour-dependent portion of the tumour in the vault-based map book. In any case, the calculation hastens. The new multi-fractal (MultiFD) involves the extraction and identification of the Gadabouts spontaneity act and the cerebral tumour fragment.6 Mind surface tumour hankie is evacuated using a MultiFD function extraction map. Improved Gadabouts characterization strategies are used to discover that the brain tissue given is a tumour or non-tumour tissue. The unpredictability of this is high. En4, for vowel image brain projection put together structure, works concerning. The work of the course is also extricated in this technique. And there is no real need for any improvement in LIPC accurate.5
A seeded tumour division strategy with new cell automation (CA) is introduced, which is contrasted and realistic cutting depending on the division technique. Seed determination and amount of suspense (VOI) shall be calculated for the successful division of the cerebral tumour. The division of the tumour region is also organized in this research. Unpredictability is very weak.7 The accuracy, however, is low. En6, the division of the mind tumour conspires. Alternate division calculation to perform High execution than the current strategy. In any case, there is a high multifaceted nature. Analysis of the division of cerebral tumours is added. Talk about different division techniques, for example, division areas, edge-based division, fluffy C represents division, Atlas-dependent division, Margo Random Field Segmentation (MRF), deformable model, deformable geometric model, precision, power, validity. En8, the determination of half-and-a-half capacity with fixed characterization applies to the mental tumour demonstrative procedure GANNIGMAC, choice.8,9 An all-encompassing tree-based methodology is used, packing C to meet the choice standards. It also deviates from the preference criteria using the determination of half and half of the functions comprising the blend (GANNIGMAC+ MRMR C + Bagging C + Decision Tree). In 9, the diffuse regulation theory is extended to order and division. The Diffuse Interference Method (DIS) is an unusual technique for mind division the controlled classification is favoured for fluffy enrollment. The execution is high and the accuracy is poor. In 10, the histogram balance is used to differentiate optimization. So fluffy Means-based division (FCM) is used to separate the tumour from the working mind image. From that point on, the Gabor utility is withdrawn from the anomaly synapses of the channel. In the long run, the haze with K is used by the closest neighbor grouping (KNN) to discover the irregularity of the desirable reverberation picture of the mind.8 The multifaceted nature is small, streaming with poor precision. Another programmed characterization of mind tumours in this work. This is achieved by the convolution of the neural network.
Literature Survey
Convolve the creation of addition to its limited neighbours near the seed of any component of the picture. This is the same as mathematical convergence. It must exist known for the sense of the medium procedure life form perform convolution, despite being similarly indicated with the example, that we keep two-three with matrix, the main one being instantly the process of flipping the sequence along with the pillar of the kernel, added to increase the shutter. The coordinate component9 in the resulting picture will be a partial grouping in which all participants join the medium panel into a discretionary appetizer base. The objective is to test downhill an illustration of the input (image-secret deposit-quantity produced medium) that falls to its dimensionality so that total rests of the type in a district scrap can be survived on the way.10 This is the implementation in the portion to help in a daydreaming form of the character more than suitable. Since the computational prices are good, by dipping the number of the study parameter plus it provides a fundamental invariance of conversion towards the interior symbol. FCN includes filled relative within a completely connected layer in the previous deposit for each activation while seeing during standard Neural Networks. Their container activations are therefore determined by a modest increase and overcome partiality. Collective weights are mainly used in two layers in the copy to use weights. This money is used to fund two separate modes with the incentive of the corresponding parameter.11 This is mainly the income that could exist in similar medium rudiments during or after different rear radiation. The specific rudiments make it simple to convert as an alternative of people on or after a solitary layer next to a single layer since they are predictable.
MATERIALS AND METHODS
The human mind is placed in recognition of the structure and use of the counterfeit neural system. The neural system is mainly used for vectors, evaluation, estimation, a gathering of information, shape coordination, improvement of capacity and clustering procedures.12 The neural system is divided into three types, as indicated by its interconnections. Three characterizations of neural systems are followed by input, criticism and repetition. The Feed-Forward neural system separates further Monolayer organizing and multilayer organizing. There is no hidden layer in the monolayer set. Yet, it only includes sources of knowledge and a layer of yields.13 However it may be, the multilayer consists of a layer of information, a layer of the enclosure and a layer of yield. The closed circled input is called the red repeater.
CNN-based classification algorithm
-
Apply the convolution channel to the primary layer.
-
The channel affectability can be reduced by smoothing the Convolution channel subsampling.
-
Moving the sign happens as of one coating to another, the layer is being monitored the initiation layer.
-
Set the time frame of preparation using a direct crushing unit.
-
The neuron in the process coating is associated with each neuron in the back layer.
-
In the planning, the misfortune layer is included at the end, providing comment to the neural system (Figure 1).
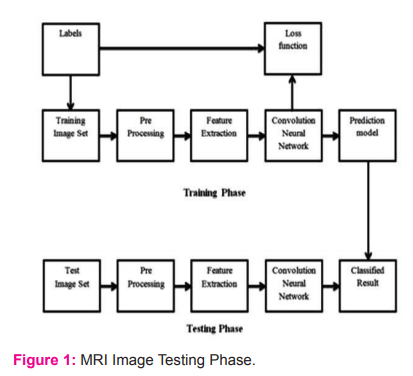
The dataset provided with brain tumour features is alienated keen on two courses, which are a preparation set and a testing set, making the system in two phases. First of all, the training phase, in this process, we fit the training set of data in CNN using a feature detection modelling the Brain Tumour Prediction System18. After finishing the model making, we fit the test dataset over the model to the test process and then measure the loss value. This is the procedure for the CNN network to predict brain tumours.
System Architectural Design
Convolution Neural Network is a nourishing onward neural system to be usually worn to analyse visual imagery by dispensation data with lattice-like topology. A CNN is also recognized as a “ConvNet” In CNN each picture is represented in the shape of an array of pixel principles (Figure 2).
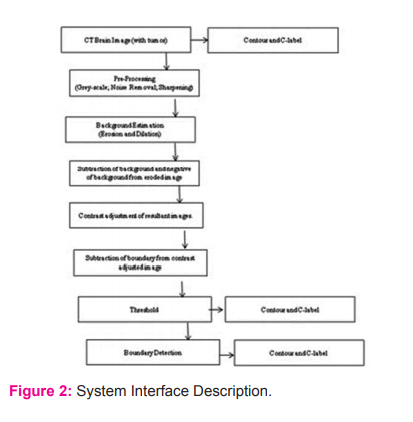
ResultS AND DISCUSSION
In the Brain Tumour using the CNN technique and test the result of a Brain tumour or Non-Brain Tumour Images are: Below are some images showing the dissimilarity amid automatic segmentation plus the real earth truth values. From the images shown below, we can see that the future model for LGG patients might notice the size, shape, size and the intra tumoural arrangement fairly exactly. Though the segmentation of tumour in LGG patients is considered as a very hard job owing to the not have of statistics, our replica for LGG patients based on move knowledge is drama quite well as tin can be seen as of the imagery underneath. Figure 3 showed two classes that are having brain tumour images and not having brain tumour images.
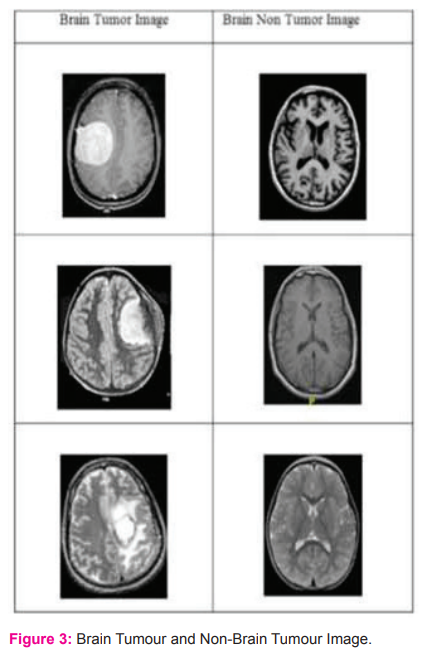
Finally, the lossless and segmented image and the data are available in Table 1 showing the result losses and accuracy and find the resultant data.
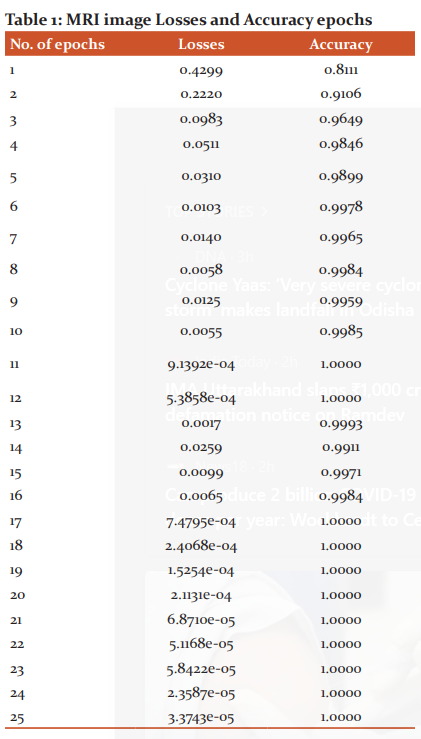
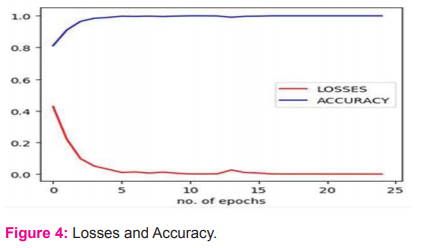
When the neural convolution network is implemented, the accuracy of the system is 0.8111 in the first epoch and the system loss is 0.4299. Throughout more times, the system increases its accuracy and reduces its losses by back-spreading and forward-spreading.15 In the last epoch, i.e. the 25th epoch, the accuracy of the system is as high as 1.0000 and the system losses are reduced to 3.3743e (Figure 4). The image above shows the output of the brain tumour and shows the output of the brain tumour after the compilation process. In this picture, there is not showing any defects according to the result ( Figure 5 and 6).
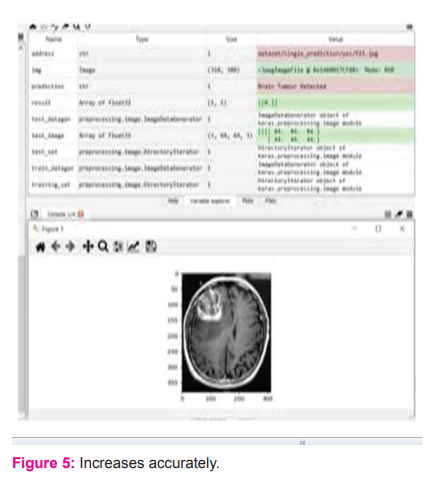
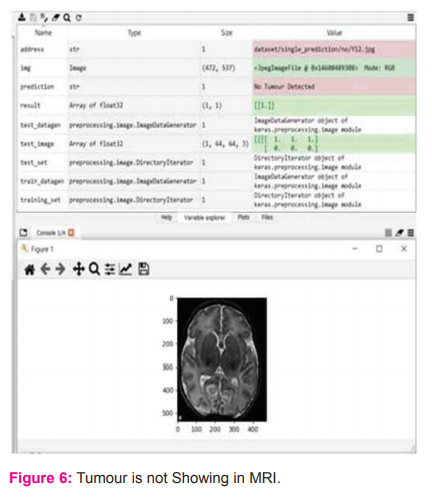
Conclusion
The fundamental goal of this thesis is to structure a highly precise, functional and ineffective programmed order of cerebral tumours. In the normal cerebrum, the tumour is grouped using the Fuzzy C Segmentation (FCM), the surface and structure, and the SVM and DNN-dependent extraction highlight the orders are made. The multifaceted complexity is very weak. In any case, the processing time is long and Accuracy is poor. Similarly, toward getting better correctness and decrease the time of measurement, a convolutions Implementation of a neural dependent grouping in the proposed plot. Besides, order the tests as predicted by the tumour or the mind.CNN is one of the deep learning strategies containing a grouping of layers for direct feed. So much Python is used for use. So many. The image is used for grouping in the net database. It's one of the models that were prepared. The preparation is finished for the last coat at this point. The rough pixel estimate with depth, width and stature values is also distinguished from CNN. Finally, the optimal angle is the average. The work of misfortune is used to achieve high accuracy. Accuracy of planning, the accuracy of approval and disasters of approval are determined. Preparation for accuracy is 97.5%. The approval quality is therefore high and the approval loss is extremely high.
Acknowledgement: Researchers examine the Automated Brain Tumour Detection using Deep Learning via Convolution Neural networks (CNN). In the CNN, convolution layer squeeze the picture as elevated skin tone, then the volitional layer reconstructs the segmented picture on or after this covering tone [12]. In this research PGI Chandigarh, Neurology Department helps in this in research provide data for experimental purpose.
Conflict of interest: There Is No Conflict Interest.
References:
-
Kumar S, Singh JN, Kumar N. An Amalgam Method efficient for Finding of Cancer Gene using CSC from MicroArray Data. Int J Emerg Technol 2020;11(3):207–211.
-
Kumar S, Negi A, Singh JN, Verma H. Deep learning for brain tumour MRI images semantic segmentation using FCN. 4th International Conference on Computing Communication and Automation (ICCCA) 2018; 14:1-4. IEEE.
-
Kumar S, Negi A, Singh JN. Semantic segmentation using deep learning for brain tumour MRI via fully convolution neural networks. information and Communication Technology for Intelligent Systems 2019;11-19. Springer, Singapore.
-
Kumar S, Negi A, Singh JN, Gaurav A. Brain Tumour Segmentation and Classification Using MRI Images via Fully Convolution Neural Networks. International Conference on Advances in Computing, Communication Control and Networking (ICACCCN) 2018; 12:178-1181).
-
Altman NS. An introduction to kernel and nearest-neighbour nonparametric regression. The Am Stat 1992;46(3):175-185.
-
Zhang J, Shen X, Zhuo T, Zhou H. Brain tumour segmentation based on refined fully convolutional neural networks with a hierarchical dice loss. arXiv preprint arXiv. 2017;1712.09093.
-
Bidros DS, Liu JK, Vogelbaum MA. Future of convection-enhanced delivery in the treatment of brain tumours. Future Oncol 2010; 6(1):117-125.
-
Sanai N. Emerging operative strategies in neurosurgical oncology. Curr Opin Neurol 2012;25(6):756-766.
-
Carlson SM, Gozani O. Emerging technologies to map the protein methylome. J Mol Biol 2014;426(20):3350-3362.
-
Eberlin LS, Norton I, Dill AL, Golby AJ, Ligon KL, Santagata S, Cooks RG, Agar NY. Classifying human brain tumours by lipid imaging with mass spectrometry. Cancer Res 2012;72(3):645-654.
-
Dahlquist KD, Salomonis N, Vranizan K, Lawlor SC, Conklin BR. GenMAPP, a new tool for viewing and analyzing microarray data on biological pathways. Nat Genet 2002;31(1):19-20.
-
Simek K, Fujarewicz K, ?wierniak A, Kimmel M, Jarz?b B, Wiench M, Rzeszowska J. Using SVD and SVM methods for selection, classification, clustering and modelling of DNA microarray data. Engi Applic Artif Intellig 2004;17(4):417-427.
-
Bilban M, Buehler LK, Head S, Desoye G, Quaranta V. Normalizing DNA microarray data. Curr Issues Mol Biol 2002;4:57-64.
-
Cho SB, Won HH. Machine learning in DNA microarray analysis for cancer classification. In Proceedings of the First Asia-Pacific bioinformatics conference on Bioinformatics 2003;19:189-198.
-
Maulik U, Chakraborty D. Fuzzy preference based feature selection and semisupervised SVM for cancer classification. IEEE Transactions on Nano Bioscience 2014 Mar 18;13(2):152-160.
-
Maji P. Mutual information-based supervised attribute clustering for microarray sample classification. Transac Knowl Data Engi 2010;24(1):127-140.
-
Duan KB, Rajapakse JC, Wang H, Azuaje F. Multiple SVM-RFE for gene selection in cancer classification with expression data. IEEE Transactions on Nano Bioscience 2005;4(3):228-234.
-
Wang X, Gotoh O. Accurate molecular classification of cancer using simple rules. BMC Med Genom 2009;2(1):64.
-
Liu H, Liu L, Zhang H. Ensemble gene selection for cancer classification. Patt Recog 2010;43(8):2763-2772.
-
Tsai YS, Aguan K, Pal NR, Chung IF. Identification of single-and multiple-class specific signature genes from gene expression profiles by group marker index. PloS One 2011;6(9):e24259.
-
Tabakhi S, Najafi A, Ranjbar R, Moradi P. Gene selection for microarray data classification using a novel ant colony optimization. Neurocomputing 2015;168:1024-1036.
-
Ren Z, Wang W, Li J. Identifying molecular subtypes in human colon cancer using gene expression and DNA methylation microarray data. Int J Oncol 2016;48(2):690-702.
-
Motieghader H, Najafi A, Sadeghi B, Masoudi-Nejad A. A hybrid gene selection algorithm for microarray cancer classification using genetic algorithm and learning automata. Inform Med Unlocked 2017;9:246-254.
-
Li Y, Kang K, Krahn JM, Croutwater N, Lee K, Umbach DM, et al. A comprehensive genomic pan-cancer classification using The Cancer Genome Atlas gene expression data. BMC Genom 2017;18(1):508.
-
Kandhasamy P, Balamurugan R, Kannimuthu S. Stellar Mass Black Hole for Engineering Optimization. In Recent Developments in Intelligent Nature-Inspired Computing 2017; 62-90. IGI Global.
-
Robson B. Computers and viral diseases. Preliminary bioinformatics studies on the design of a synthetic vaccine and a preventative peptidomimetic antagonist against the SARS-CoV-2 (2019-nCoV, COVID-19) coronavirus. Comput Biol Med 2020:103670.
-
Pickering CR, Zhang J, Neskey DM, Zhao M, Jasser SA, Wang J, et al. Squamous cell carcinoma of the oral tongue in young non-smokers is genomically similar to tumours in older smokers. Clin Cancer Res 2014;20(14):3842-3848.
|