IJCRR - 12(20), October, 2020
Pages: 152-156
Date of Publication: 27-Oct-2020
Print Article
Download XML Download PDF
Potato Plant Disease Detection Using Convolution Neural Network
Author: Pitchai R, Sharath Kumar G, Ashutosh Varma D, Madhu Babu CH
Category: Life Sciences
Abstract:Background: In countries like India, whose primary occupation is agriculture faces a huge loss when the crops get affected by any disease. These diseases attack the crops in various stages and destroy the entire production. Since most of the diseases are transmitted from one crop to another there is much need to detect the exact type of disease the crop has been affected so that farmers can take the required steps to the \"save the crops\" and production. But detecting the kind of disease that a crop has been affected is very difficult for farmers since there are various kinds of diseases. Method: There are so many classification techniques, such as k-Nearest Neighbor Classifier, Probabilistic Neural Network, Genetic Algorithm, Support Vector Machine, and Main Component Analysis, Artificial Neural Network, and Fuzzy Logic. It is difficult to select the best classification method as compared to other methods the system will be more reliable. This article presents a dissection of various techniques used to find the disease of the plants. Results and Observation: The developed system is capable of detecting diseases in plants and is also capable of providing treatments that can be used against them. To improve the health of the plant, we need to deal with it with sufficient knowledge of the disease and cure. The framework proposed is implemented using python and the google GPU(Graphical Processor Unit) used provided 80 % accuracy. Conclusion: The proposed model used a convolution a neural network model based on SSD mobile network for data training. Some checkpoints are created after training the model. We need to take the last Model Checkpoint to create a file that is used for testing. A particular file type with the.pb extension is created by using the checkpoint file. The model provided 80% of accuracy.
Keywords: Plant Disease Detection, Neural networks, Genetic algorithm, KNN, PCA, Fuzzy Logi
Full Text:
Introduction
India is a cultivated nation that relies on farming for approximately 70% of its population. Farmers have a wide range for the option of different suitable crops and the search for effective plant pesticides. Plant disease leads to a substantial decrease in both farm goods quality and quantity. The aim of agriculture is not just to feed people but also to provide a major energy source and solution to the worldwide problem of global warming. To order to treat and monitor the disease, the diagnosis of plant disease is very important at an earlier level. Plant disease studies include analyses of visually tracked plant trends.
During the early days, the specialist in this field was able to manually track and examine plant diseases, who would notice the light colour changes on the plant leaf, but this takes an incredibly long processing period. The image processing techniques are then used to solve this problem. Health monitoring and plant disease identification are very relevant to sustainable farming. Manually tracking plant diseases is very difficult. It needs a large amount of effort, plant disease experience and an unnecessary time for production. The analysis of photographs is also used to identify plant diseases.
Detecting diseases requires measures such as collection, preprocessing, segmentation of images, extraction of features and classifications. Within the following (Section III) the literature review provides brief details on the different methods used to detect plant diseases.
Motivation
The leaves are a delicate part of the plant; the assessment of farm harvest is of dynamic importance. The leaf's texture and colour is the most significant visual property. Therefore it is important to identify leaf diseases to assess farm products, enhance the market value and comply with quality standards. This is also important to recognise and take additional measures to further spread diseases. It's going to be too slow. When physical methods are used to classify and categorize the method, we also need the professionals to ensure it gets incorrect, and who's less readily available.
The works are categorized according to colour, scale, etc. When such procedures are documented in an automated system by using the correct software design language, the effort becomes faster and error-free. There are two main features to be achieved, speed and precision in the methods of machine-learning for plant disease detection. Technologies such as automated detection and classification of plant diseases using floor image processing techniques are required. It proves useful for farmers and warns them at the right time before the disease spreads over a wide area.
Literature Survey
T. Van der Zwet et al. (2002)1 developed a model of identification of plant diseases using CNN to classify 13 different types of plant diseases. Tatem and Rogers et al. (2006)2 researched the Global Transportation Networks and the transmission of infectious diseases and their effects on epidemiology in particular. J. R. Rohr et al. (2008)3 studied the relations between climate, disease transmission and declines in amphibians. Steinwart and A. Christmann et al. (2008)4 used the algorithm K -means to cluster the data set. Mrunalini R. et al. (2011)5 identified clustering of K-means and artificial intelligence in pattern recognition of crop diseases. A. New spectral indices (NSIs) developed by K. Mahlein et al. (2013)6. NSI is useful for the identification of crops of various diseases.
H. Cartwright et al. (2015)7 identified the Artificial Neural Networks to simulate human brain model functioning. Raj Kumar et al. (2016)8 addressed Hybrid Features and Automated Leaf Disease Detection and Classification Supervised Classifier. They also built a database consisting of around 32,000 different species of plants that are pre-processed, such as image reshaping, resizing and converting to a collection. Related processing happens on the test image as well. H. Azizpour et al. (2016)9 used Convolution networks to examine many factors affecting transferability, design, training data distribution, feature extraction etc.
J. Garcia Arnal Barbedo et al. (2018)10 used the Annotated Plant Pathology Databases for the identification and recognition of diseases based on photos. G. Zhou et al. (2019)11 suggested, using FCM-KM and Faster R-CNN fusion, a technique to resolve the various problems with rice disease detection accuracy. Adnan Mushtaq et al. (2019)12 proposed a model for plant disease detection and offers solutions using coevolutionary neural networks. Raghavendran S et al. (2019)13 addressed the categorisation of leaf disease using vector machine support technique. Sankaran et al. (2010)14 surveyed some of the advanced plant disease identification techniques.
Tyr Wiesner-Hanks et al. (2019)15 outlined a two-step process for producing large quantities of high-quality training data with limited expert feedback. Liu, LOuyang, W and Wang, X et al. (2020)16 reviewed more than 300 research papers including several views on the detection of generic objects: frameworks for identification, representation of object characteristics, generation of object proposals, context modelling, training strategies, and metrics for assessment. M Pothen, E and D. M. L. Pai, (2020)17 suggested a classification system for the rice leaf disease.
The following parameters were considered: bacterial leaf blight, Leaf smut and Brown spot diseased images, and Otsu's segmentation method was used. Sun et al. (2020)18 proposed the multi-scale detection of feature fusion using neural networks of convolution. J. Li et al. (2020)19 proposed a system for the identification, location and study of marine cucumber using a Faster R-CNN. W. As a new extraction design for the Rice Blast detection Chen et al. (2020)20 implemented a revolutionary spore germination method. Rasul Ruzmetov et al. (2020)21 reviewed the morphological properties of apple trees with cytosporosis disease. Anjan Kumar Payra et al. (2013)22 protein function for prediction.
Methodology
The basic steps of plant disease detection are shown in figure 1. The steps involved in our methodology are,
-
Image Acquisition
-
Image Pre-processing
-
Image Segmentation
-
Feature Extraction
-
Classification
-
Image Acquisition: The initial procedure is to collect all infected or diseased plant leaf images via a camera or a source, which stores all infected plant leaf data. The first photo is in RGB type (Red, Green and Blue). In this colour transformation method, a device-independent colour-space transition is generated for an RGB leaf image and then used for the colour transformation method.
-
Image Pre-processing: The contaminated plant leaf in the picture produces noise. The noise here may be dust, on the leaf sand and other stuff. To get more accuracy it is important to remove the noisy data from the image. Pre-processing techniques are then used to eliminate noisy image images. There are several pre-processing techniques available some of which are Image clipping, i.e. cropping of the image of the leaf to get the area of interest. Smoothing of the image is achieved with the smoothing filter. Improvement of the picture is carried out to improve the contrast. The result it generates is dependent on any one of the techniques used.
-
Image Segmentation: Segmentation means partitioning the image into separate parts with the same features, or having some resemblance. The segmentation can be achieved using various approaches such as clustering k-means, converting an RGB image to HIS model etc. Here, we used the algorithm K Means. The clustering of K-means is used to classify objects based on a collection of features into the number of classes K. Object classification is achieved by minimizing the sum of the squares of the distance between the object and the cluster in the query.
-
Feature Extraction: Extraction of features plays a key role in detecting a plant disease. Extraction function is used in many image processing applications. Colour, texture, shape, edges etc. are the characteristics that can be used in the identification of plant diseases. Morphological results are found to provide better results than the other features. Texture means how the image is formed in colour, the roughness, the image's hardness. This method collects the characteristics of tainted vegetable leaves.
-
Classification: After the extraction of the function is done, a classifier is created which is used to identify the plant disease. Depending on their leaves, the plant disease can be classified and is defined as healthy and unhealthy leaves. The unhealthy leaf is further graded based upon the type of disease with which it has been attacked.
Results
Our system is capable of reliably detecting diseases on potato plants. We need to deal with this with ample knowledge of the disease and cure to improve the plant's health. The framework implemented using python and the google GPU(Graphical Processor Unit). Figure 2 provides samples of the results of our system. Figure 2.a shows Healthy early blight potato leaf, Figure 2.b shows Healthy late blight potato leaf and Healthy potato leaf Figure 2.c. Our system delivered 80 per cent accuracy.
Discussion
The input for the model is photos of potato leaf that include two disease types, one of which is early bright and the other late bright. The images input which is passed to the model is as follows. To get more accuracy for the model, the input images are preprocessed to eliminate noise data, dust, and other stuff from the images.
We applied the transfer learning technique to a predefined convolutional neural network model i.e., SSD mobile net for training the data. SSD_Mobilenet is a neural network algorithm which is used for object detection. SSD is a framework used for multi-box detectors and Mobilenet is used for classification. Only by combining both, it is used for object detection. The job of mobile net layers is to convert the pixels from input image to feature that defines the image and hence it is used as a feature extractor. With the help of the mobile net, we can classify the image category. The following is the procedure for training and testing the model.
Initially, we collect a few hundred images that contain the required leaf images i.e., potato diseased leaf from an external source. We need to annotate the infected potato images by using a software called LabelImg because we need to draw a box around the infected region for the leaf image and must specify the class for it to train it. This Label Img software automatically will create an Extensible Markup Language (XML) file that describes the leaf image with infected region dimensions. We need to split the images data into train and test samples. The train samples are used for training the model and test samples are used for testing the model whether it is producing the required results or not.
As we have discussed in the above statement LabelImg software creates an XML file but the model( SSD mobile net) does not accept the XML file for training we need to change the XML file to another file format so that we can use that file to train the model. We can convert the XML file to comma-separated values (CSV) file by using some code. After the conversion of a file from XML to CSV use the generated train.csv file to train the model. The ssd_mobilenet model uses tensor flow as a backend for object detection. It takes more time to train the model in normal systems. So we have used google open-source cloud platform i.e., Google Colaboratory which provides GPU environment and storage space up to an extent for the user. It is faster when compared to normal systems that do not have a Graphical Processor Unit (GPU) accessibility. The platform runtime is only 12 hours So we have to be careful while training the model. To train the predefined model we need to change some code in the model configuration file to our requirement and save it.
While training, the model generates some checkpoint files at some interval of time so we need to save those checkpoints accordingly to start the training from that checkpoint even if the platform runtime restarts. We can start the training from a particular checkpoint by using a checkpoint file by changing some code in it. In the ssd_mobilenet configuration file, there will be something like execution steps. We need to mention a value by which the training ends whenever it reaches the specified execution step. The output of the model is as follows in fig 2.
Conclusion
Our proposed system was built taking into account the benefits of the farmers and the farming industry. The established system is capable of detecting disease in plants and also providing the remedy that can be taken against it. We will handle it by proper knowledge of the disease and the cure to improve the plant's health. The framework proposed is implemented using python and gives approximately 80 % accuracy. Use Google GPU to process will improve accuracy and speed. Shortly, we will be able to train the model with more data that can cover all the diseases that are present and it should be able to suggest the precautions. It can also be implemented in drones so that the drones capture the leaves from the top and detects the kind of disease that the plant has affected and provide the necessary instructions for the farmers to take further action to stop the disease.
Acknowledgement
We thank our colleagues from B.V. Raju Institute of Technology, Narsapur who provided insight and expertise that greatly assisted the research, although they may not agree with all of the interpretations/conclusions of this paper. We are also grateful to authors/ editors/publishers of all those articles, journals and books from where the literature for this article has been reviewed and discussed.
Conflict of Interest: There is no conflict of interest.
Financial Support: Not applicable
Human or animal study Ethical clearance letter: Not Applicable
Informed consent: Not Applicable
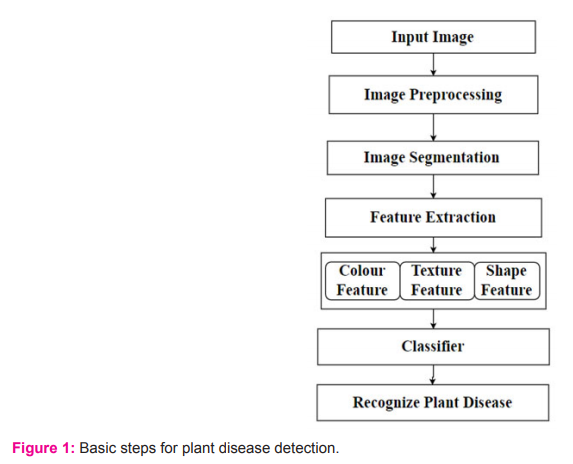
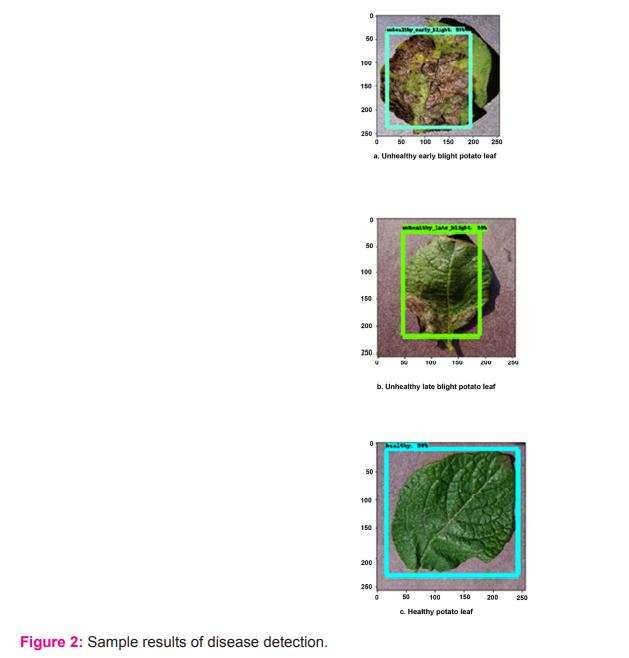
References:
-
Van der Zwet, T. Present Worldwide Distribution Of Fire Blight. Acta Hortic, 2002; 590: 33-34.
-
Tatem, D. J. Rogers, and S. I. Hay et al. Global transport networks and infectious disease spread, Advances in Parasitology, 2006; 62: 293–343.
-
Rohr JH, Raffel TR, Romansic JM, McCallum H, and P. J. Hudson et al. Evaluating the links between climate, disease spread, and amphibian declines, Proceedings of the National Academy of Sciences of the United States of America. 2008; 105(45): 17436–17441.
-
Steinwart and Christmann R. Support Vector Machines, Springer Science and Business Media, New York, NY, USA, 2008.
-
Mrunalini R. Badnakhe. An Application of K-Means Clustering and Artificial Intelligence in Pattern Recognition for Crop Diseases, 2011 International Conference on Advancements in Information Technology With workshop of ICBMG 2011: 134-138.
-
Mahlein AK et al. Development of spectral indices for detecting and identifying plant diseases, Remote Sensing of Environment. 2011; 128: 21–30.
-
Cartwright H, Ed., Artificial Neural Networks, Humana Press, 2015.
-
Raj Kumar S, Sowrirajan S. Automatic Leaf Disease Detection and Classification using Hybrid Features and Supervised Classifier, Int. J. Adv. Res. Electrical, Electronics and Instrumentation Engg. 2016; 5(6): 4556-4563.
-
Azizpour H et al. Factors of Transferability for a Generic ConvNet Representation, IEEE Transactions on Pattern Analysis and Machine Intelligence, 2016; 38(9): 1790-1802.
-
Garcia J, Arnal B. Annotated Plant Pathology Databases for Image-Based Detection and Recognition of Diseases, in IEEE Latin America Transactions,2018; 16(6): 1749-1757.
-
Zhou G, Zhang W, Chen A, He M and Ma X. Rapid Detection of Rice Disease Based on FCM-KM and Faster R-CNN Fusion, IEEE Access, 2019;7: 143190-143206.
-
Adnan Mushtaq et al. Plant Disease Detection using CNN & Remedy, Int. J. Adv. Res. Electrical, Electronics and Instrumentation Engg. 2010; 8(3): 622-626.
-
Raghavendran S et al. Leaf Disease Classification using SVM Classifier in Cloud, Int. J. Innovative Techn. Exploring Engg. (IJITEE), 2019; 9(1): 4146-4149.
-
Sankaran S, Mishra A, Ehsani R, and Davis C et al. A review of advanced techniques for detecting plant diseases, Computers and Electronics in Agri. 2010; 72(1):1–13,
-
Tyr Wiesner-Hanks et al. Millimeter-Level Plant Disease Detection From Aerial Photographs via Deep Learning and Crowdsourced Data, Tech. Adv. Plant Sci. 2019; 10: 1-11.
-
Liu, L., Ouyang, W., Wang, X. et al. Deep Learning for Generic Object Detection: A Survey. Int. J. Comput Vis, 2020; 128: 261–318.
-
Pothen ME and Pai DM, Detection of Rice Leaf Diseases Using Image Processing, 2020 Fourth International Conference on Computing Methodologies and Communication (ICCMC), Erode, India, 2020; 424-430.
-
Sun J, Yang Y, He X and Wu X, Northern Maize Leaf Blight Detection Under Complex Field Environment Based on Deep Learning, in IEEE Access, 2020; 8: 33679-33688.
-
Li J, C. Xu L, Jiang, Y, Xiao L, Deng Z and Han Z, Detection and Analysis of Behavior Trajectory for Sea Cucumbers Based on Deep Learning, in IEEE Access, 2020; 8: 18832-18840.
-
Chen W, Lin Y, Ng Y, Liu C and Lin Y. Rice Talk: Rice Blast Detection Using Internet of Things and Artificial Intelligence Technologies, in IEEE Internet of Things Journal, 2020; 7:2: 1001-1010.
-
Rasul Ruzmetov, Yulduz Matyakubova, Ikram Abdullaev. Cytosporosis Diseases of Apple Trees (Reinette Simirenkomalus) and It’s Distribution in the Lower Amudarya Region International Journal of Current Research and Review. 2020;12 (14): 62-67.
-
Payra, Anjan. Function Prediction using Cluster Analysis of Unannotated Align Sequences. International Journal of Current Research. 2013; 5: 134.
|