IJCRR - 4(7), April, 2012
Pages: 06-10
Print Article
Download XML Download PDF
UNIQUE APPLICATION OF DATA ENTRY PROCESSES IN LONGITUDINAL MOTOR DEVELOPMENTAL STUDY
Author: Amitesh Narayan, U.V. Shenoy, Sailakshmi Ganesan, Narayan Prabhu
Category: Healthcare
Abstract:Objectives: Medical research in longitudinal motor developmental studies brings collection of large data base. Therefore need felt to develop an effective raw data entry method in electronic format using existing software i.e. MS Excel. Methods: 42 infants/children were recruited at the time of birth and were followed up to 36 months of age on Peabody Developmental Motor Scales-2nd edition (PDMS-2) instrument. All 42 subjects had been assessed for 9 times during follow ups at various chronological age points. Total numbers of follow up assessments were 378 i.e. 42 (subjects) × 9 (no. of follow up). Total number of data entry cell required for each subject in this study was approximately 2611. Thus total data entry cell in this study became i.e. 42* 2611 = 109662. Results: Through this process, each child's raw data digits i.e. >2600 cells, took only 20 to 30 minutes to enter and processed automatically as summary sheet. This process took 21-22 hours for entry of 109662 raw data digits (i.e. 42* 2611 = 109662) in the electronic format. The entered data in the summary sheet had 99% accuracy. Conclusions: This method is very useful for any number of raw data entries in electronic format in a short span of time for the research works of any type. It is easy and unique, because, it uses very simple concept of formula applications to bring the data entries in a summary sheet, while typing the data in a different sheet of the same MS Excel file, for the ease of typing and data errors verification. This method is cost effective also, since the application software MS Excel is easily accessible.
Full Text:
INTRODUCTION
An element of data is an item, idea, concept or raw fact. 1 In motor developmental studies, data should be organized in such a way that we can understand and retrieve them when needed. 2 To understand better about it, we must know what is data? The data is defined as ?representation of facts or concepts or instructions in a formalized manner, suitable for communication, interpretation or processing by manual or electronic means. 1 Data accuracy and data validity are two key principles of data quality. 3 To maintain data quality, health care data must include accuracy, validity, reliability, completeness, legibility, timeliness, accessibility, usefulness and costeffectiveness. 1,4 In recent years, data quality has been an important issue for two basic purposes: 3
- a. to promote high standards of patient care, and
- b. to facilitate accurate and timely dissemination of research data for the statistical analysis.
To be useful, the data must be accurate, valid and conform to an expected range of values. Thus it is important that collected data is correct at the point of entry and includes all relevant variables. 3 Since data recording is subject to human error, there is a need to have built-in control measures to eliminate errors, both in manual recording and computer entry. 3 Steps to check the data entry in the research record should be taken at the point of entry, because data accuracy is crucial for analysis purposes. 3 A popular method for data entry is ?Plan, Do, Check and Act? (PDCA) method. 1 The steps involve plan phase, implementation phase, check phase and act phase. 1 Longitudinal developmental studies can provide information that cannot be obtained from crosssectional studies; therefore, they have considerable appeal to research workers. 3,5 Medical research in longitudinal motor developmental studies causes collection of large data base, where data for one or more variables are recorded at more than one point in time. This necessitates that computer systems must have inbuilt edits and validation checks for accurate statistical analysis. 3, 5 Collection of accurate data is only the first step. It is also extremely important that errors are not introduced in the process of converting the data to electronic format. 6 Many studies use doubleentry verification to catch and correct the data entry errors. 6 New technologies are continually being developed to convert raw data to electronic format more efficiently. It is extremely important that data entry software has mechanisms to check the accuracy of entered data at the entry level itself. Some of the newest technologies involve scanning paper forms directly into the computer, or faxing forms to a central data center (where fax machine serves as scanner to convert the data to electronic format). These are efficient data entry system though not cost effective; but it is essential that scanned files are manually checked for accuracy. 6 Data Presentation is also important to ensure the quality of statistics. 3 Commonly all researchers presently type the data in MS Excel manually, whether the data to be entered is less/more without having any other easy options for error verifications. Therefore need felt to develop a cost effective raw data entry method for a longitudinal motor developmental study (having many variables) in to the electronic format using existing software i.e. MS Excel; having all essential properties of new technologies applied for the raw data entry
METHODOLOGY
In this longitudinal motor developmental study, 42 infants/children were recruited at the time of birth and were followed up to 36 months of age on Peabody Developmental Motor Scales-2 nd edition (PDMS-2)7 instrument. During ≤12 month‘s period, frequency of assessment was 3 monthly; but from ≥12 months, the same was 6 monthly. Therefore all 42 subjects had been assessed for 9 times during follow ups at various chronological age points. Thus total numbers of follow up assessments were: 42 (subjects) × 9 (no. of follow up) = 378. This process generated massive data digits. At each age point of assessments many variables were obtained for the purpose of statistical analysis of the data i.e. item scores, raw scores, standard scores, age equivalents, percentiles quotients and performance ratings. Therefore total number of data entry cell required for each subject in this study was approximately 2611. So, the total data entry cell in this study became i.e. 42* 2611 = 109662. The need of such large numbers of data digits entry in MS Excel format required us to go through a planned procedure to ensure that entered data must be cross verified for its accuracy before being subjected to statistical analysis.
New Data Entry Method:
Commonly, research data entered by the researchers needs to be arranged in such a way that it should be easily understood by the statistician in order to understand the study and its variables. In ?MS Excel‘ there is rows and columns and each data gets its entry in one cell. The data stored in cells can be recognized by its cell no. i.e. Column No. x Row No. It helps the data to be recognized easily and detect the errors in the entered data.
Generally while entering the data we often follow ?HORIZONTAL‘ wise data entry pattern (because in SPSS software, when the data gets opened through excel format, the naming of variables is automatic for each case), which is very difficult to type i.e. in a single row. If the data is many, we may lose the track of cells and so errors in the data. As a researcher, we faced extreme difficulty during horizontal manner data typing, since there were approximately 2611 cell entries in this study for each subject. Knowing that data entry must happen without any error and there must be an option for easy data retrieval as well as smooth and simple data verification. So, with the help of data entry system manager, we decided that each infant‘s record will be kept separately and a summary file will be derived from each record by using cell number references. Thus first a blank MS Excel file was prepared containing 3 sheets and the sheets were renamed as ?SUMMARY‘, ?DATA‘ and ?TOTAL‘. Their details are explained below:
a. SUMMARY Sheet: It contained the format as per the statistical requirements for the data analysis (horizontal wise). e.g.

b. DATA Sheet: It contained 239 rows (for raw data variables) and 9 columns (representing 9 age points of recordings), e.g. same way as it is shown below. Each variable was given different cell color to recognize the variables at one stoke, in order to avoid mistakes while entering the data. Also if the data entry is done vertically, it would be very easy to drag one cell to the end, provided the data to be entered is the same. By doing so, it became extremely easy to enter the raw data variables.
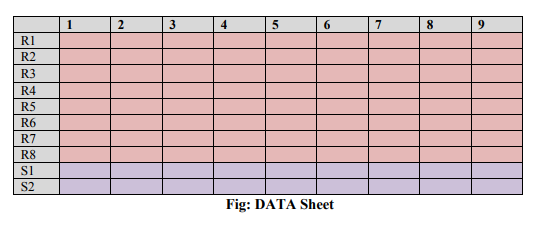
c. TOTAL Sheet: It contained 6 (rows) x 5 (columns) for 9 test results representing 9 chronological age points of assessments. It is shown below.
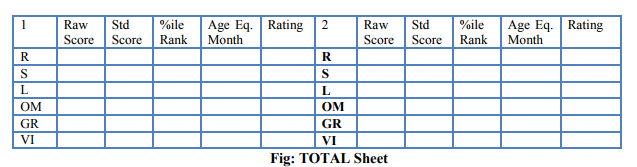
Finally all the data were transported for each case in SUMMARY sheet through the formula (the cell value which is appeared in the other sheet) E.g. As shown below. Here AK is Column, and 3 is Row. So in cell AK3 formula is stored as =DATA!B311 i.e. in DATA sheet B311 cell value).
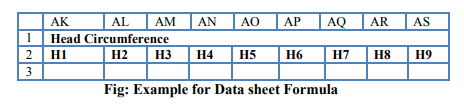
Through this process, the values stored in other sheets are brought to the SUMMARY SHEET, which is actually required for the statistical use (see appendix-1).
RESULTS
By this process, each child‘s raw data (>2600 cells) took only 20 to 30 minutes to enter and processed automatically as SUMMARY SHEET, which is actually required for the statistical analysis (see appendix-1). The SUMMARY SHEET contains data of all children in one place. Through this mechanism total raw data digits, i.e. 42* 2611 = 109662, took 21-22 hours for entry in the electronic format. The accuracy of entered data was verified manually by creating a PDF file print physically of each case (see appendix-2). The entered data in the SUMMARY SHEET had 99% accuracy. DISCUSSION The process adopted in this study for raw data entry is unique and cost effective, as MS EXCEL is commonly used application software. Simple formula concepts were applied in this study to bring the data together as SUMMARY SHEET in horizontal orientation (while typing the data in vertical orientation or in tabular form) (see appendix-1). By this process, large number of raw data digits could easily be converted in to the electronic format in short span of time, with 99% accuracy (with options for data error verification), for statistical analysis purposes. This would be a very useful concept for senior or junior level individual researchers, as it can save their lots of time required for data typing, and help in preventing data digit errors during data entry.
CONCLUSIONS
This method is very useful for any number of raw data entries in electronic format in a short span of time for the research works of any type. It is easy and unique, because, it uses very simple concept of formula applications to bring the data entries in a summary sheet, while typing the data in a different sheet of the same MS Excel file, for the ease of typing and data errors verification. This method is cost effective also, since the application software MS Excel is easily accessible.
ACKNOWLEDGEMENT
Authors acknowledge the immense help received from the scholars whose articles are cited and included in references of this manuscript. The authors are also grateful to authors / editors / publishers of all those articles, journals and books from where the literature for this article has been reviewed and discussed. Authors also wish to acknowledge Mr. K. A. Shenoy of Sri Venkateshwar Computer Camp, Car Street, Mangalore for giving this concept and preparing the MS Excel format files.
References:
1. Abdelhak M, Grostick S, Hankin MA, Jacobs E. Health Information: Management of a Strategic Resource. Philadelphia, WB Saunders Company, 1996.
2. Davis N, Lacour M. Introduction to information technology. Philadelphia, WB Saunders, 2002.
3. Improving data quality: A guide for developing countries. World Health Organization. World Health Organization 2003. Regional office for the western pacific, United Nations Avenue, 1000 Manila, Philippines. ISBN 92 9061 050 6
4. Donabedian A. quality assessment and assurance: unity of purpose, diversity of means. Inquiry: 25, 1988: 173–195.
5. Roche AF, Guo S. Methods and analysis issues in longitudinal studies of growth. Wright State University School of Medicine. http://www.amstat.org/sections/srms/procee dings/papers/1988_024.pdf.
6. Whitney CW, Lind BK, Wahl PW. Quality assurance and quality control in longitudinal studies. Epidemiol Rev. 20 (1), 1998: 71-80.
7. Folio MR, Fewell RR. Peabody Developmental Motor Scales: Examiner‘ Manual. 2nd ed. Austin, Tex: PRO-ED. Inc; 2000
|