IJCRR - 5(12), June, 2013
Pages: 134-145
Date of Publication: 28-Jun-2013
Print Article
Download XML Download PDF
FUNCTION PREDICTION USING CLUSTER ANALYSIS OF UNANNOTATED ALIGN SEQUENCES
Author: Anjan Kumar Payra
Category: Technology
Abstract:Proteins are responsible for nearly every task of cellular life, including cell shape and inner organization, product manufacture and waste cleanup, and routine maintenance. Proteins also receive signals from outside the cell and mobilize intracellular response. Experimental procedures for protein function prediction are inherently low throughput and are thus unable to annotate a non-trivial fraction of proteins that are becoming available due to rapid advances in genome sequencing technology [15]. This has motivated the development of computational techniques that utilize a variety of high-throughput experimental data for protein function prediction. So, there is need to design algorithm to find similar functional proteomic sequence from large set of sequence database. Here we present a novel unsupervised method, called Function Finder (in short F-Func) for identification function of unannotated proteomic sequence. F-Func uses clustering of sequence information represented by numerical features, performing filtering, assigned score and meet with the criterion produces decision. Using help of producing result estimate success rate of F-Func method. Estimated success rate of F-Func methods, which is almost 70%.
Keywords: Sequence, Homology, motif, F-Func, Prediction, Cluster.
Full Text:
INTRODUCTION
Proteins are macromolecules that serve as building blocks and functional components of a cell, Proteins are responsible for some of the most important functions in an organism. Such categorization of the types of functions a protein can perform has been suggested by Bork [1] et al. [1998]:
- Molecular function: The biochemical functions performed by a protein, such as ligand binding, catalysis of biochemical reactions and conformational changes.
- Cellular function: Many proteins come together to perform complex physiological functions, such as operation of metabolic pathways and signal transduction.
- Phenotypic function: The integration of the physiological subsystems, consisting of various proteins performing their cellular functions, and the interaction qof this integrated system.
In order to predict function we need to study amino acid sequence, where concept of central dogma is crucial. The central dogma [2] of molecular biology is the conversion of a gene to protein via the transcription and translation phases as shown in Fig. 1. The result of this process is a sequence constructed from twenty amino acids, and is known as the protein’s primary structure. This sequence is the most fundamental form of information available about the protein since it determines different characteristics of the protein.
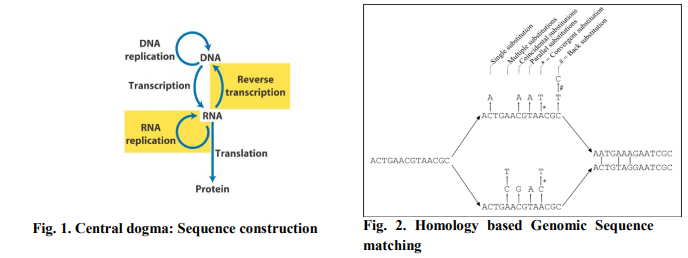
Here using data sequences are closely related with protein phosphorylation, mediated via a group of enzymes (called kinases) that performs addition of phosphate (PO4) group usually to serine (S), threonine (T), tyrosine (Y) residues, is one of the most frequent forms of posttranslational modification mechanism [3]. Function of a gene or protein can predicted using different approaches .Those are given below – ? Homology-based Function Prediction : Homologous genes or proteins are derived from a common ancestral sequence, as given in Fig. 2, where always searching for same constituent. Homologous proteins within or among species are similar in sequence and are likely, but not guaranteed to have a similar function. Comparison of an un-annotated sequence to known homologous sequences is therefore a good starting point for predicting function. Generally, a new sequence could be used to query protein databases using BLAST, to find proteins of known function with high sequence similarity, and their function is transferred to the query sequence.
Several approach have proposed a multi-step strategy for functional annotation based on clustering of protein sequences according to their similarities [Xie et al. 2002; Abascal and Valencia 2003; Sasson et al. 2006]. Figure. 3 show the flowchart of the basic strategy adopted in these two studies. The algorithm starts with the construction of the similarity matrix that stores the BLAST similarity values between the protein sequences in the original training set [15]. This matrix is then used to cluster these sequences, and the annotation of a sequence in these approaches depends not on individual homologous sequences but a cluster composed of many such sequences.
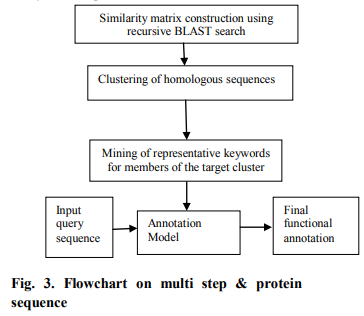
Function Prediction Using Sequence Motifs : Motifs are defined as sub-sequences which are conserved across a set of protein sequences belonging to a family [Bork and Koonin 1996]. Owing to their conservation property, they are candidates for functional sites in proteins, such as sites for ligand binding, DNA binding and interactions with other proteins, and thus are useful as clues for predicting the function of a protein [Bork and Koonin 1996; Huang and Brutlag[2001].
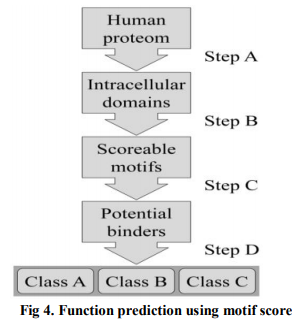
Motif databases such as PROSITE ('database of protein domains, families and functional sites') can be searched using a query sequence as given in Fig. 4. Motifs can, for example, be used to predict sub cellular localization of a protein (where in the cell the protein is sent after synthesis). ? Structure-based Function Prediction: 3D protein structure is generally better conserved than protein sequence, structural similarity is a good indicator of similar function in two or more proteins. Many programs have been developed to screen an unknown protein structure against the Protein Data Bank. The prediction of function from structure, and the ways these perspectives have been formulated in various approaches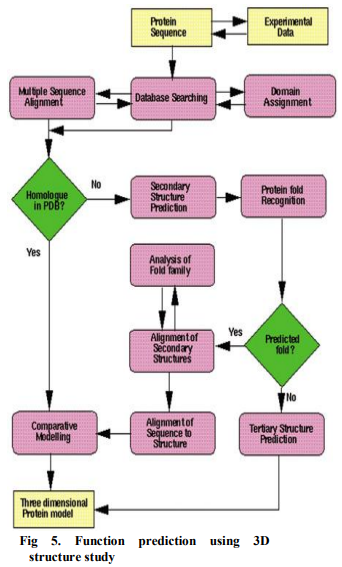
Genomic Context-based Function Prediction: The protein function prediction are not based on comparison of sequence or structure as above, but on some type of correlation between novel genes/proteins and those that already have annotations. Also known as phylogenetic profile, these genomic context based methods are based on the observation that two or more proteins with the same pattern of presence or absence in many different genomes most likely have a functional link. Whereas homology-based methods can often be used to identify molecular functions of a protein, context-based approaches can be used to predict cellular function, or the biological process in which a protein acts [15]. For example, proteins involved in the same signal transduction pathway are likely to share a genomic context across all species, as given in Fig. 6.
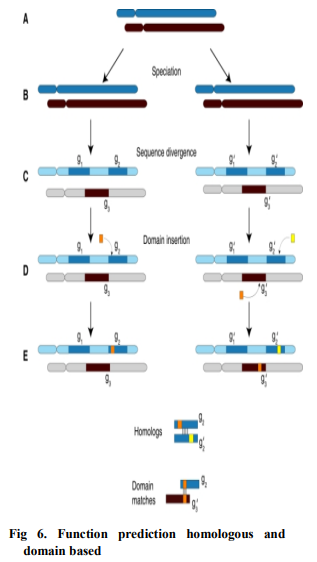
Protein interaction networks and protein complexes: A protein almost never performs its function in isolation. Rather, it usually interacts with other proteins in order to accomplish a certain function. At the highest level, they can be categorized into genetic and physical interactions. Genetic interactions occur when the mutations in one gene cause modifications in the behavior of another gene, which implies that these interactions are only conceptual and do not occur physically in a genome. Of particular interest in our project are the physical interactions between proteins, since they are more directly related to the process through which a protein accomplishes its functions [15]. The interactions can be structured to form a network, and hence the name protein interaction networks as shown in Fig. 7 and Fig. 8.
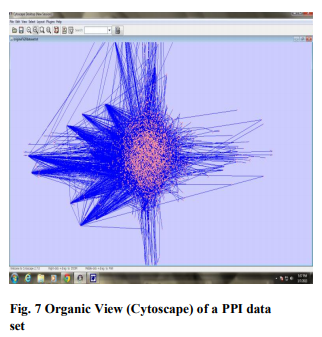
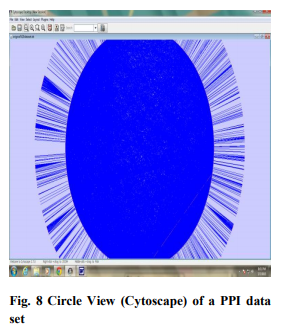
Present growth of the protein sequence and structure databases, there remains only a small fraction of proteins whose functions have been experimentally characterized. It is sometimes possible to infer the function of uncharacterized proteins by comparison to the sequences or structures of functionally annotated homolog. Common descent does not necessarily imply functional similarity, however (Hegyi and Gerstein 1999[4] ; Devos and Valencia 2000 [5]; Todd et al. 2001[6]) and functional annotation transferred from one homologous protein to another. Several investigators have considered the problem of functional site prediction using multiple sequence alignments (Casari et al. 1995[7]; Andrade et al. 1997[8]; Hannenhalli and Russell 2000; Li et al. 2003).Casari et al. (1995), applied principal component analysis to a vector representation of protein sequences in a multidimensional ?sequence space,? to derive subfamily-specific residues involved in protein function. Andrade et al. (1997) proposed a rigorous clustering algorithm based on a self-organizing map as a means to identify protein subfamilies and retrieve characteristic sequence patterns. Some methods of functional site prediction use phylogenetic analysis to identify residues associated with functional divergence (Lichtarge et al. 1996[9]; Sjolander 1998[10]; Aloy et al. 001[11]; Madabushi et al. 2002[12]). PRESENT WORK Motivation: Many approaches have been discussed in the previous section over sequence. After studying and going through various papers it can be analyzed that very few assessments had been pursued on clustering this analyzation has encouraged us to work it. I am using homology based similarity to create different clusters to predict functional group of unannotated sequences in F-Func methods. Database and Tools: Data has been collected only serine –phophorylated peptides of length 13 (i.e., 13-mers centered at serine) from the Phospho.ELM database, which are experimentally determined to be substrates of different kinases. Motif –X [13] and F-motif [14] are used for analyzing my algorithms, which are given in next section. Propose Method To predict function of an unannotated sequence using F-Func, we divide three major portions. First phase is to form clusters based on matching criteria. Next two are different approaches to predict. Algorithm: Cluster formation Step1: Consider sequence (Seqi) ={S1,S2,…..Sn}, n≤ 25000. Step 2: Eliminate all repetitions from Seqi and obtained non repeated sequence ( Seqi). Step 3: Consider central (j=6) residue element (……S……) of a sequence of length 13. Extract left pattern (L(Seqij),i≤n, 0≤ j ≤5 ) and right pattern(R(Seqij),i≤n, 7≤ j ≤13 ) for each Seqi. Step 4: Comparing the Seqi with left pattern and generate left cluster (LCP ,p
Step 5: Select random sequence from LCP∩ RCq and consider it as unannotated sequence (Sequn). After cluster formation, our objective is to predict functions of unannotated sequences logically or mathematically, which should be new and well justified. Here, I will discuss two approaches of predicting sequences. 1st approach is given belowAlgorithm: Assigned functional group to unannotated sequence (Sequn) using mean value calculation. Step 1: Consider one of the cluster from collection of clusters (LCP or RCq ) and select sequences from it. Count the number (N0) of sequences for selected cluster. Step 2: Count maximum occurrence (Scount) of a constitutes (eg. S, G, R, P, A etc) present in the sequences of respectively clusters. Step 3: Estimate mean (M) value - . The value of M is assigned as score of the corresponding cluster. Step 4: Repeat the step 1 to 3 till there no cluster is left. LCP € Ø, RCq€ Ø. Step 5: Merge two clusters if selected constitutes (eg. S) and score values are same for both. Step 6: Select a sequence (Si) from Sequn. Estimate occurrence of constitutes in it. Assigned the maximum value to Emax . If Si can’t find suitable clusters to estimate distance for Emax and constitutes, than select next maximum occurrence of constitutes for Si and continue. Step 7: Calculate distance of Si from clustersStep 8: Sequentially Si is more nearer to the cluster with minimum value. Si is more functionally related with the cluster. The sequence Si is placed and function is assigned of the respective cluster. Step 9: Finally, estimate success rate of prediction. Through multiple sequence alignments, patterns of characteristic residues may emerge. Above algorithm is based on basic paradigms Homology. Here basic principal is -
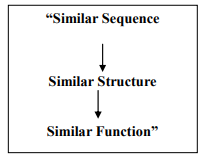
Success rate is always crucial while predicting functional group of a sequence. Here, key in my approach is principal. 2 nd approach is quite similar with 1st approach, it will provide more accurate result as compare previous one. The approach is given belowAlgorithm: Assigned functional group to unannotated sequence (Sequn) using max value calculation of sequence constituents. Step 1: Consider one of the cluster from collection of clusters (LCP or RCq ) and select sequences from it. Count the number (N0) of sequences for selected cluster. Step 2: Count maximum occurrence (Scount) of a constitutes (eg. S, G, R, P, A etc) present in the sequences of respectively clusters. Step 3: Estimate max (CM) constitutes value to assign score of a cluster. [eg. Cluster (N) contains S=8, G=3,R=2.Then CM =8 for S of Cluster(N)] Step 4: Repeat the step 1 to 3 till there no cluster is left. LCP € Ø, RCq€ Ø. Step 5: Merge two clusters if selected constitute (eg. S) and score values are same for both. Step 6: Select a sequence (Si) from Sequn .Estimate occurrence of constitutes in it. Assigned the maximum value to Emax . If Si can’t find suitable clusters to estimate distance for Emax and constitutes, than select next maximum occurrence of constitutes for Si and continue. Step 7: Calculate distance of Si from clusters Step 8: Sequentially Si is more nearer to the cluster with minimum value. Si is more functionally related with the cluster. The sequence Si is placed and function is assigned of the respective cluster.
Step 9: Finally, estimate success rate of prediction. Illustration using example Algorithms of F-Func are applied over prealigned data set of length 13 mers. So, here obtain motif using F-motif [13] and motif-X [14] tools, generate web logo, which is given.
Illustration using example:
Algorithms of F-Func are applied over prealigned data set of length 13 mers. So, here obtain motif using F-motif [13] and motif-X [14] tools, generate web logo, which is given in Fig. 9. Cluster formation, assign scores to the clusters using above
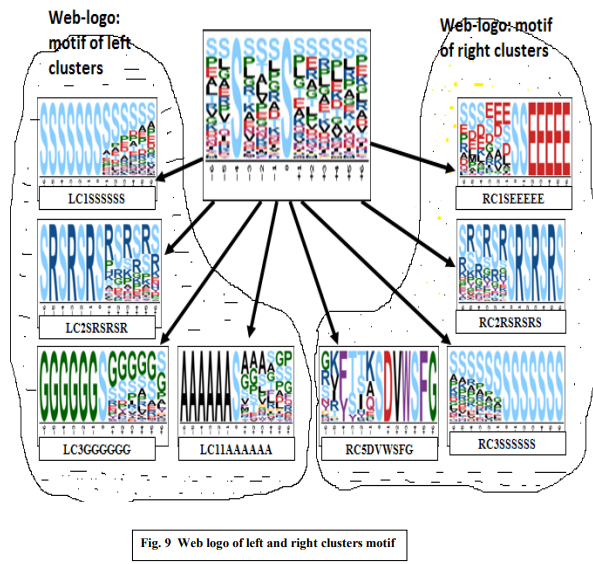
given algorithms are given below. Depending on left and right match sequence form left and right clusters assigned scores using mean and max value calculation. In tabular format, it is given in table 1
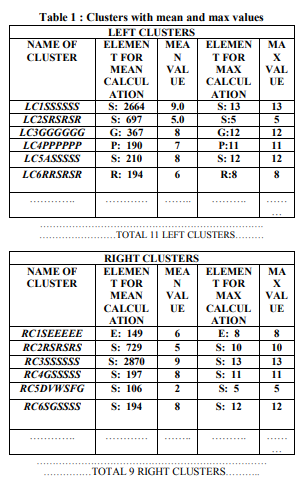
After assigned score values to clusters, next select sequence from intersection of left and right cluster randomly and treated it as unannotated sequence. Considering an unannotated sequence to predict functional group, count max occurrence of constituent and assign as a score of the sequence. Estimate distance values (dj ) from all clusters (LCP and RCq ) using above given algorithms. It is given below in Fig. 10. Unannotated sequence will assign by the functional group of the cluster whose distance value is minimum.
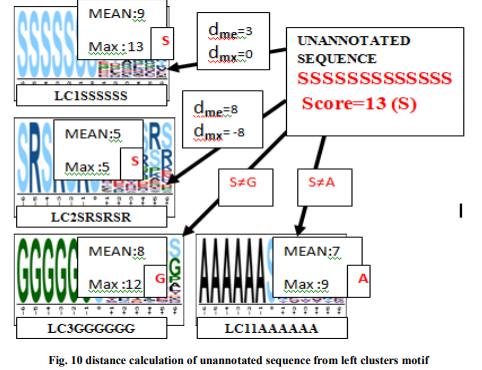
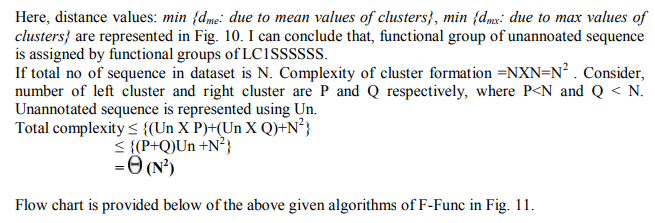
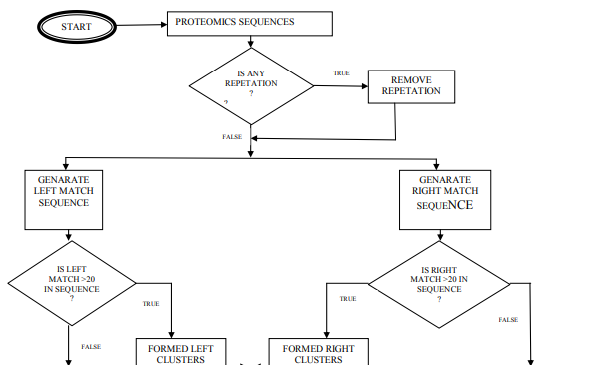
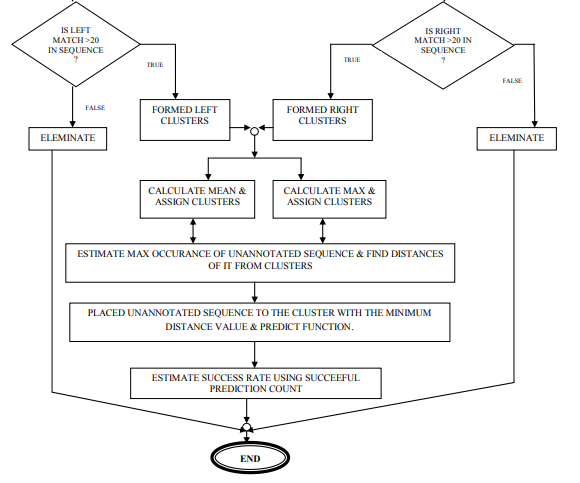
Proposed algorithms of F-Func are applied to predict functional groups of unannoated sequences. The results are given in tabular format as table 2. It is concluded to results that, Max approach produces better outcomes as compare Mean approach. It is represented graphically in Fig.12.
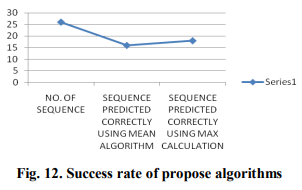
CONCLUSION
It is quite clear that, using homology we can predict unannoated sequences successfully. Even success rate can be more than 70% as per my algorithms. Hopefully F-Func algorithms will provide better results for larger dataset. My proposed work adds a dimension to existing methods. Hopefully, performance of the F-Func algorithms will increase if length of left-match and right-match is increased.
ACKNOWLEDGEMENT
It is great help received from the scholars whose articles cited and included in references of this manuscript. I am also grateful to authors / editors / publishers of all those articles, journals and books from where the literature for this article has been reviewed and discussed. Hence, I am grateful to IJCRR editorial board members and IJCRR team of reviewers who have helped me to bring quality to this
References:
1. Bork, P. and Koonin, E.V. 1998. Predicting functions from protein sequences—Where are the bottlenecks? Nat. Genet. 18 313–318.
2. A.K.Payra and S.Saha, IJCET.2013.Generic approach for predicting unannotated protein pair function using protein.page.142-159
3. Cheng, Chung, Aguan, Yang, Wang, N.Paul, PLoS ONE,2011, Dicovery of protein Phosphorylation Motif through Exploratory Data analysis.
4. Anna R. Panchenko, Fyodor Kondrashov, and Stephen Bryant - Prediction of functional sites by analysis of sequence and structure conservation, 2004Devos, D. and Valencia, A. 2000. Practical limits of function prediction. Proteins 41 98–107.
5. Todd, A.E., Orengo, C.A., and Thornton, J.M. 2001. Evolution of function in protein superfamilies, from a structural perspective. J. Mol. Biol. 307 1113–1143.
6. Casari, G., Sander, C., and Valencia, A. 1995. A method to predict functional residues in proteins. Nat. Struct. Biol. 2 171–178.
7. Andrade, M.A., Casari, G., Sander, C., and Valencia, A. 1997. Classification of protein families and detection of the determinant residues with an improved self-organizing map. Biol. Cybern. 76 441–450.
8. Lichtarge, O., Bourne, H.R., and Cohen, F.E. 1996. An evolutionary trace method defines binding surfaces common to protein families. J. Mol. Biol. 257 342–358.
9. Sjolander, K. 1998. Phylogenetic inference in protein superfamilies: Analysis of SH2 domains. Proc. Int. Conf. Intell. Syst. Mol. Biol. 6 165–174.
10. Aloy, P., Querol, E., Aviles, F.X., and Sternberg, M.J. 2001. Automated structure-based prediction of functional sites in proteins: Applications to assessing the validity of inheriting protein function from homology in genome annotation and to protein docking. J. Mol. Biol. 311 395–408.
11. Madabushi, S., Yao, H., Marsh, M., Kristensen, D.M., Philippi, A., Sowa, M.E., and Lichtarge, O. 2002. Structural clusters of evolutionary trace residues are statistically significant and common in proteins. J. Mol. Biol. 316 139–154.
12. http://motif-x.med.harvard.edu/motif-x.htm
13. http://f-motif.classcloud.org 14. “Computational Approaches for Protein Function Prediction: A Survey?- Gaurav Pandey, Vipin Kumar and Michael Steinbach
|